The WDC Training Dataset and Gold Standard for Large-Scale Product Matching
Companion Proceedings of The 2019 World Wide Web Conference(2019)
摘要
A current research question in the area of entity resolution (also called link discovery or duplicate detection) is whether and in which cases embeddings and deep neural network based matching methods outperform traditional symbolic matching methods. The problem with answering this question is that deep learning based matchers need large amounts of training data. The entity resolution benchmark datasets that are currently available to the public are too small to properly evaluate this new family of matching methods. The WDC Training Dataset for Large-Scale Product Matching fills this gap. The English language subset of the training dataset consists of 20 million pairs of offers referring to the same products. The offers were extracted from 43 thousand e-shops which provide schema.org annotations including some form of product ID such as a GTIN or MPN. We also created a gold standard by manually verifying 2200 pairs of offers belonging to four product categories. Using a subset of our training dataset together with this gold standard, we are able to publicly replicate the recent result of Mudgal et al. that embeddings and deep neural network based matching methods outperform traditional symbolic matching methods on less structured data.
更多查看译文
关键词
deep matching, embeddings, entity resolution, evaluation data, product matching, schema.org annotations
AI 理解论文
溯源树
样例
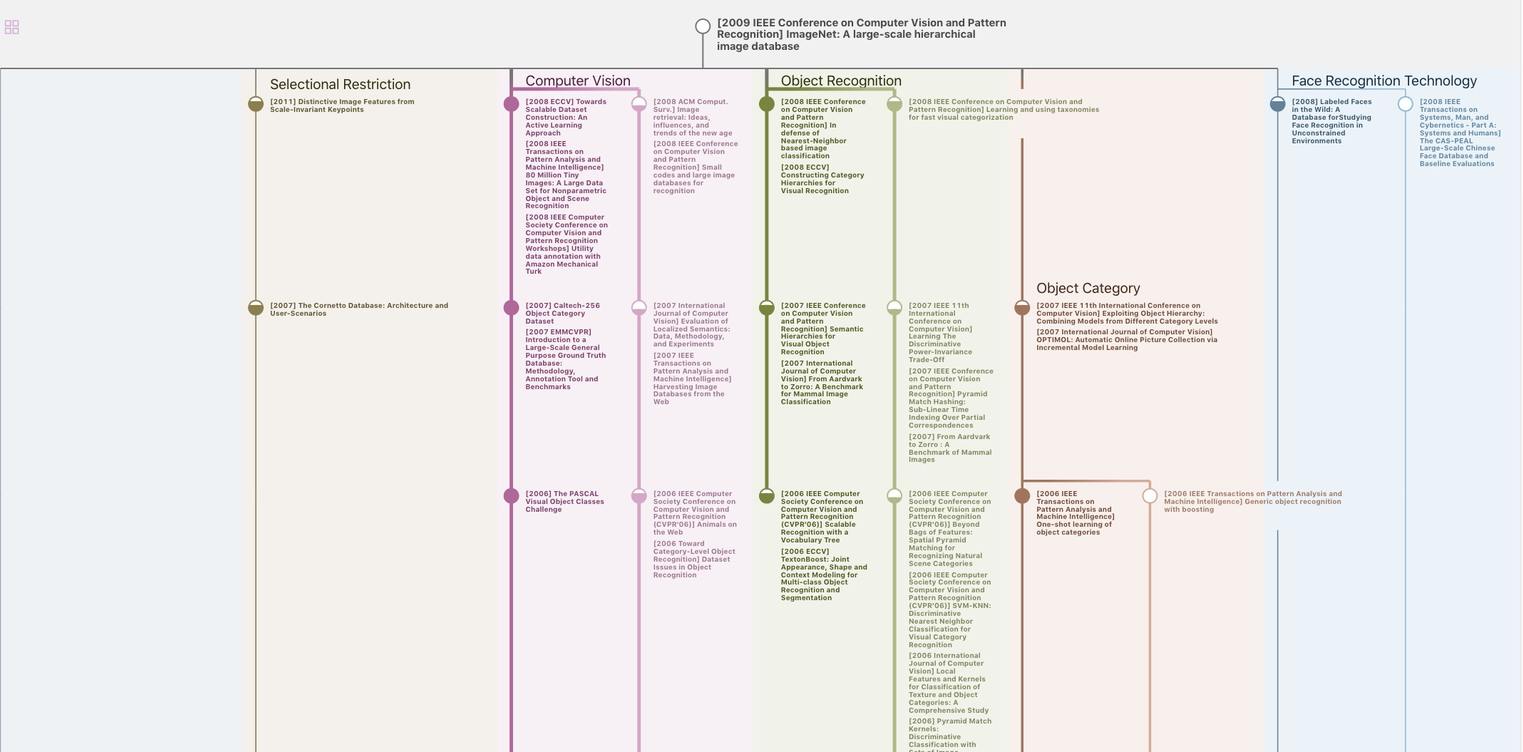
生成溯源树,研究论文发展脉络
Chat Paper
正在生成论文摘要