Collaborative Explanation of Deep Models with Limited Interaction for Trade Secret and Privacy Preservation
Companion Proceedings of The 2019 World Wide Web Conference(2019)
摘要
An ever increasing number of decisions affecting our lives are made by algorithms. For this reason, algorithmic transparency is becoming a pressing need: automated decisions should be explainable and unbiased. A straightforward solution is to make the decision algorithms open-source, so that everyone can verify them and reproduce their outcome. However, in many situations, the source code or the training data of algorithms cannot be published for industrial or intellectual property reasons, as they are the result of long and costly experience (e.g. this is typically the case in banking or insurance). We present an approach whereby individual subjects on whom automated decisions are made can elicit in a collaborative and privacy-preserving manner a rule-based approximation of the model underlying the decision algorithm, based on limited interaction with the algorithm or even only on how they have been classified. Furthermore, being rule-based, the approximation thus obtained can be used to detect potential discrimination. We present empirical work to demonstrate the practicality of our ideas.
更多查看译文
关键词
Auditing, Explainability, Machine Learning, Privacy, Transparency
AI 理解论文
溯源树
样例
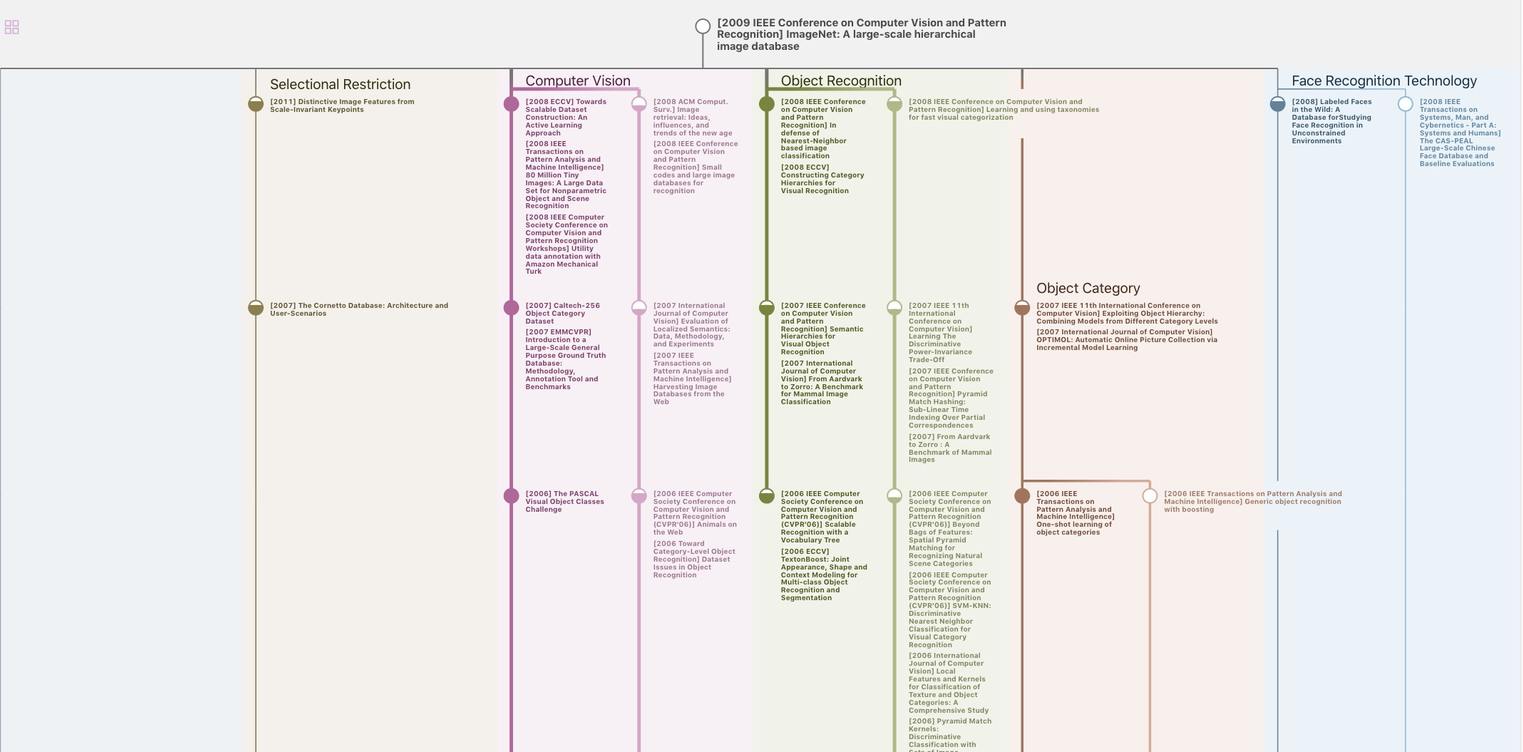
生成溯源树,研究论文发展脉络
Chat Paper
正在生成论文摘要