Reputation Deflation Through Dynamic Expertise Assessment in Online Labor Markets
WWW '19: The Web Conference on The World Wide Web Conference WWW 2019(2019)
摘要
Current reputation systems in online labor markets (e.g., Freelancer, PeoplePerHour) experience three major shortcomings: (1) reputation inflation (i.e., reputation scores are inflated to “above average” values) (2) reputation attribution (i.e., attribution of reputation scores to individual skills is unfeasible) and (3) reputation staticity (i.e., reputation scores are uniformly averaged over time). These shortcomings render online reputation systems uninformative, and sometimes even misleading. This work proposes a data-driven approach that deflates reputation scores by solving the problems of reputation attribution and saticity. The deflating process starts with projecting any random set of skills to a set of competency dimensions. For each competency dimension, a Hidden Markov Model estimates a contractor's current (but latent) competency-specific expertise. Aggregation of competency-specific estimates provides expertise predictions for any given set of required skills. Empirical analysis on 61,330 completed tasks from a major online labor market shows that the resulting estimates are deflated and they better predict contractor performance. These results suggest a series of implications for online (labor) markets and their users.
更多查看译文
关键词
Digital markets, Expertise assessment, HMM, Online markets, Reputation deflation, Word embeddings
AI 理解论文
溯源树
样例
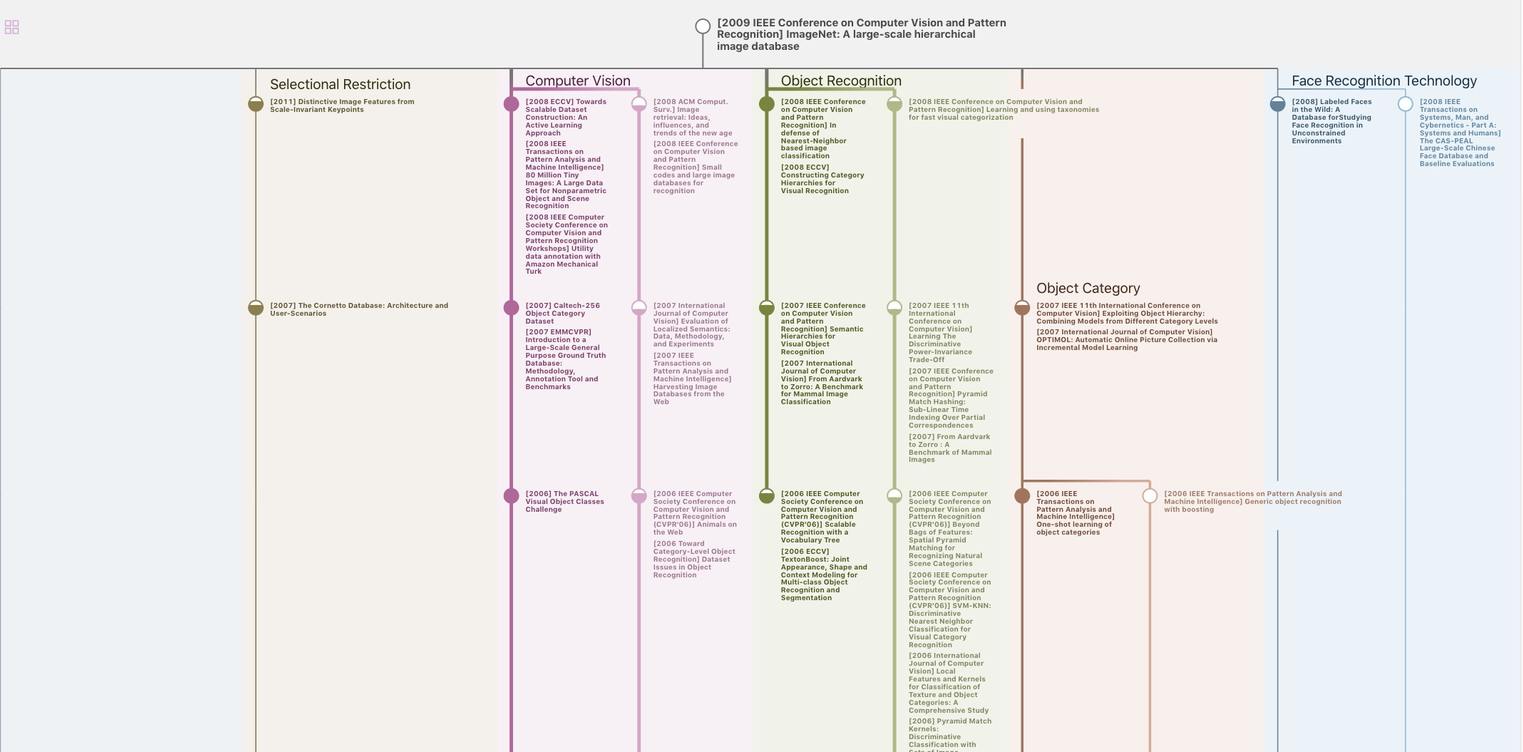
生成溯源树,研究论文发展脉络
Chat Paper
正在生成论文摘要