Multi-class Twitter Data Categorization and Geocoding with a Novel Computing Framework.
Cities(2020)
摘要
This study details the progress in transportation data analysis with a novel computing framework in keeping with the continuous evolution of the computing technology. The computing framework combines the Labeled Latent Dirichlet Allocation (L-LDA)-incorporated Support Vector Machine (SVM) classifier with the supporting computing strategy on publicly available Twitter data in determining transportation-related events to provide reliable information to travelers. The analytical approach includes analyzing tweets using text classification and geocoding locations based on string similarity. A case study conducted for the New York City and its surrounding areas demonstrates the feasibility of the analytical approach. Approximately 700,010 tweets are analyzed to extract relevant transportation-related information for one week. The SVM classifier achieves >85% accuracy in identifying transportation-related tweets from structured data. To further categorize the transportation-related tweets into sub-classes: incident, congestion, construction, special events, and other events, three supervised classifiers are used: L-LDA, SVM, and L-LDA incorporated SVM. Findings from this study demonstrate that the analytical framework, which uses the L-LDA incorporated SVM, can classify roadway transportation-related data from Twitter with over 98.3% accuracy, which is significantly higher than the accuracies achieved by standalone L-LDA and SVM.
更多查看译文
关键词
Social media,New York,Traffic operation,Short-term planning,Machine learning,Traffic management policy
AI 理解论文
溯源树
样例
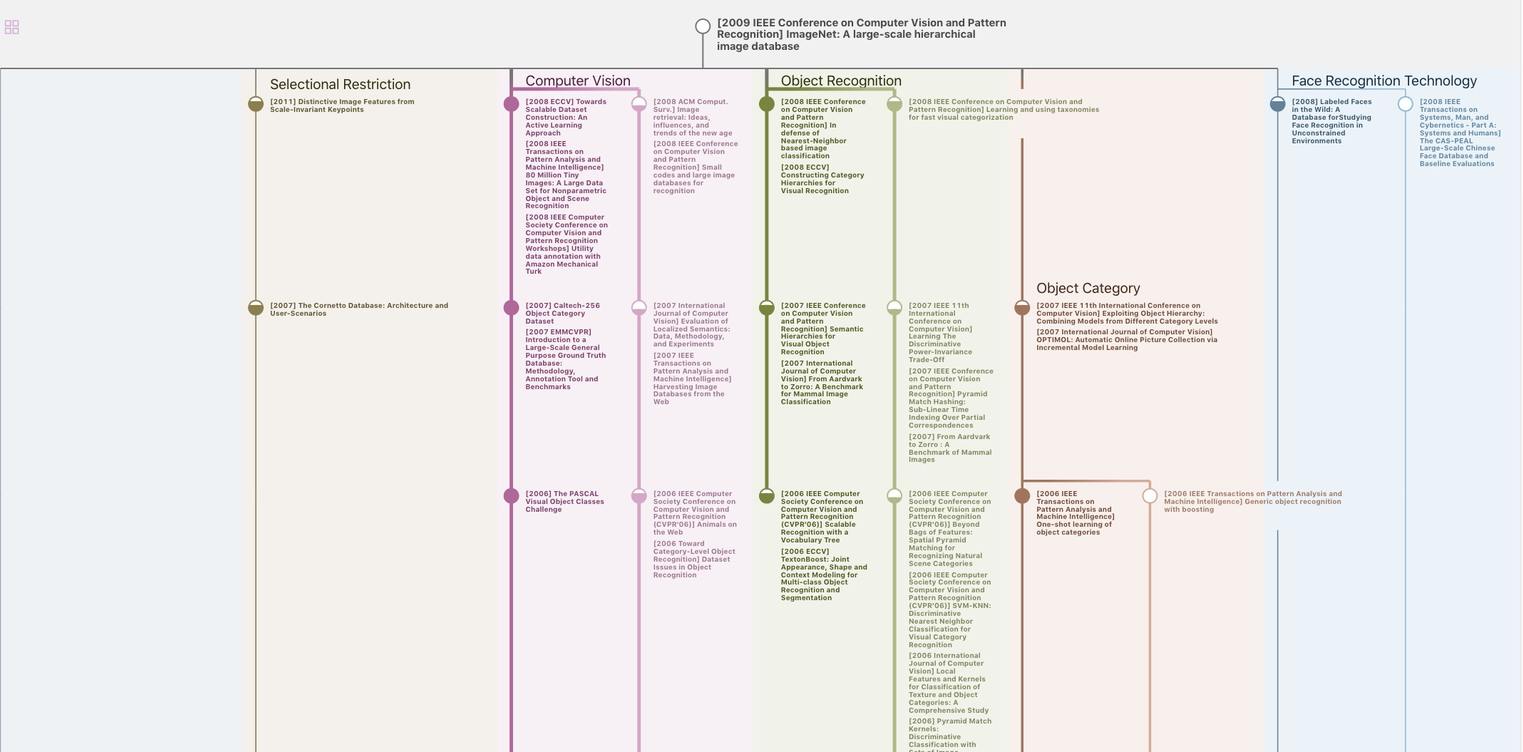
生成溯源树,研究论文发展脉络
Chat Paper
正在生成论文摘要