Enhanced Brain Inspired Model for Face Categorization Using Mutual Information Maximization
2018 25th National and 3rd International Iranian Conference on Biomedical Engineering (ICBME)(2018)
摘要
Human visual system can robustly and simply recognize complex objects in cluttered natural scenes. So far, numerous computational models have been developed to mimic the computational process of this considerable system for machine vision systems. HMAX is known as one of the best computational models which have been inspired by hierarchical structure of the human visual cortex. During learning stage of the HMAX, a large number of small part of training images, called patches, are extracted at random positions. These patches are in various sizes and orientations. The random selection of patches, not only degrades the performance but also increases the computational complexity of HMAX-based object recognition systems. In this paper, we focus on this drawback and propose a new method based on information theory to select more relevant patches and remove redundant ones. The proposed method is developed for a face categorization task in which the purpose is to detect the presence or absence of faces in real world images. The performance of the proposed method has been evaluated on face image database CalTech101 and its recognition rate is superior to the original HMAX by more than 5%.
更多查看译文
关键词
Computational modeling,Feature extraction,Gabor filters,Brain modeling,Face,Visualization,Entropy
AI 理解论文
溯源树
样例
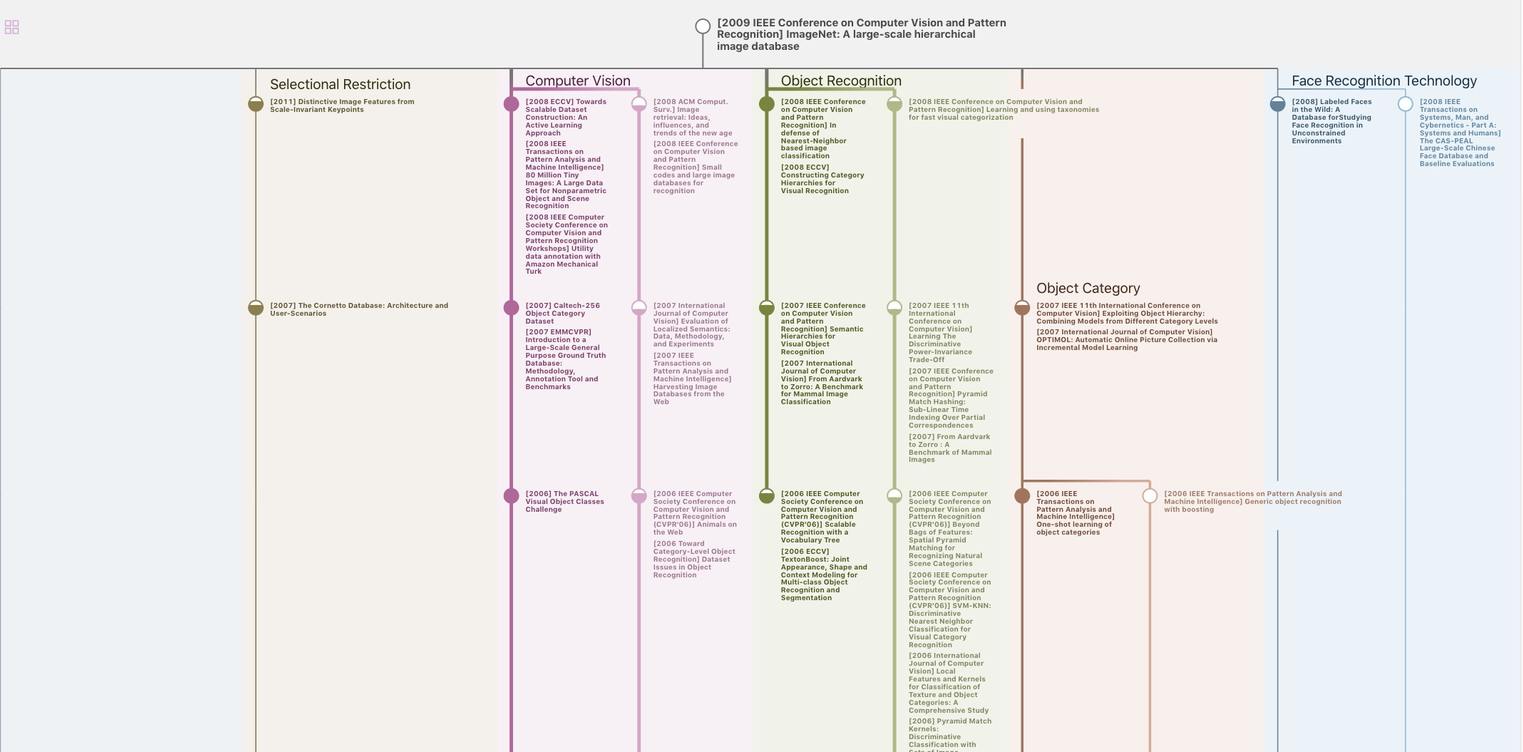
生成溯源树,研究论文发展脉络
Chat Paper
正在生成论文摘要