General multilevel adaptations for stochastic approximation algorithms of Robbins–Monro and Polyak–Ruppert type
Numerische Mathematik(2019)
摘要
In this article we present and analyse new multilevel adaptations of classical stochastic approximation algorithms for the computation of a zero of a function f:D →ℝ^d defined on a convex domain D⊂ℝ^d , which is given as a parameterised family of expectations. The analysis of the error and the computational cost of our method is based on similar assumptions as used in Giles (Oper Res 56(3):607–617, 2008 ) for the computation of a single expectation. Additionally, we essentially only require that f satisfies a classical contraction property from stochastic approximation theory. Under these assumptions we establish error bounds in p th mean for our multilevel Robbins–Monro and Polyak–Ruppert schemes that decay in the computational time as fast as the classical error bounds for multilevel Monte Carlo approximations of single expectations known from Giles (Oper Res 56(3):607–617, 2008 ). Our approach is universal in the sense that having multilevel implementations for a particular application at hand it is straightforward to implement the corresponding stochastic approximation algorithm.
更多查看译文
关键词
Primary 62L20, Secondary 60J10, 65C05
AI 理解论文
溯源树
样例
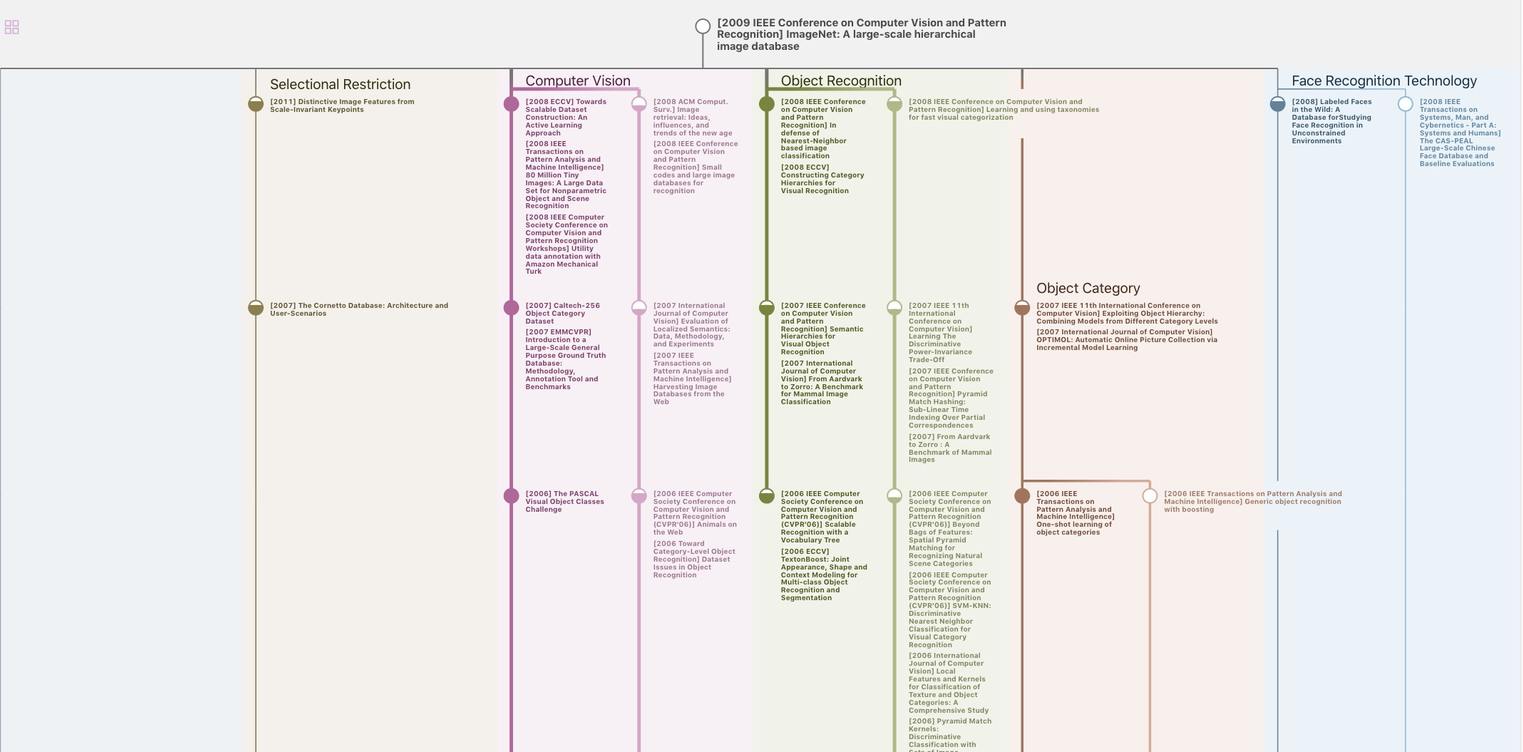
生成溯源树,研究论文发展脉络
Chat Paper
正在生成论文摘要