Deep learning approach to generate offline handwritten signatures based on online samples
IET Biometrics(2019)
摘要
Reduced training sets are major problems typically found on the task of offline signature verification. To increase the number of samples, the use of synthetic signatures can be taken into account. In this work, a new method for the generation of synthetic offline signatures by using dynamic and static (real) ones is presented. The synthesis is here faced under the perspective of supervised training: the learning model is trained to perform the task of online-to-offline signature conversion. The approach is based on a deep convolutional neural network. The main goal is to enlarge offline training dataset in order to improve performance of the offline signature verification systems. For this purpose, a machine-oriented evaluation on the BiosecurID signature dataset is carried out. The use of synthetic samples (in the training phase) generated with the proposed method on a state-of-the-art classification system exhibits performance similar to those obtained using real signatures; moreover, the combination of real and synthetic signatures in the training set is also able to show improvements of the equal error rate.
更多查看译文
关键词
learning (artificial intelligence),image classification,handwriting recognition,feedforward neural nets,convolution
AI 理解论文
溯源树
样例
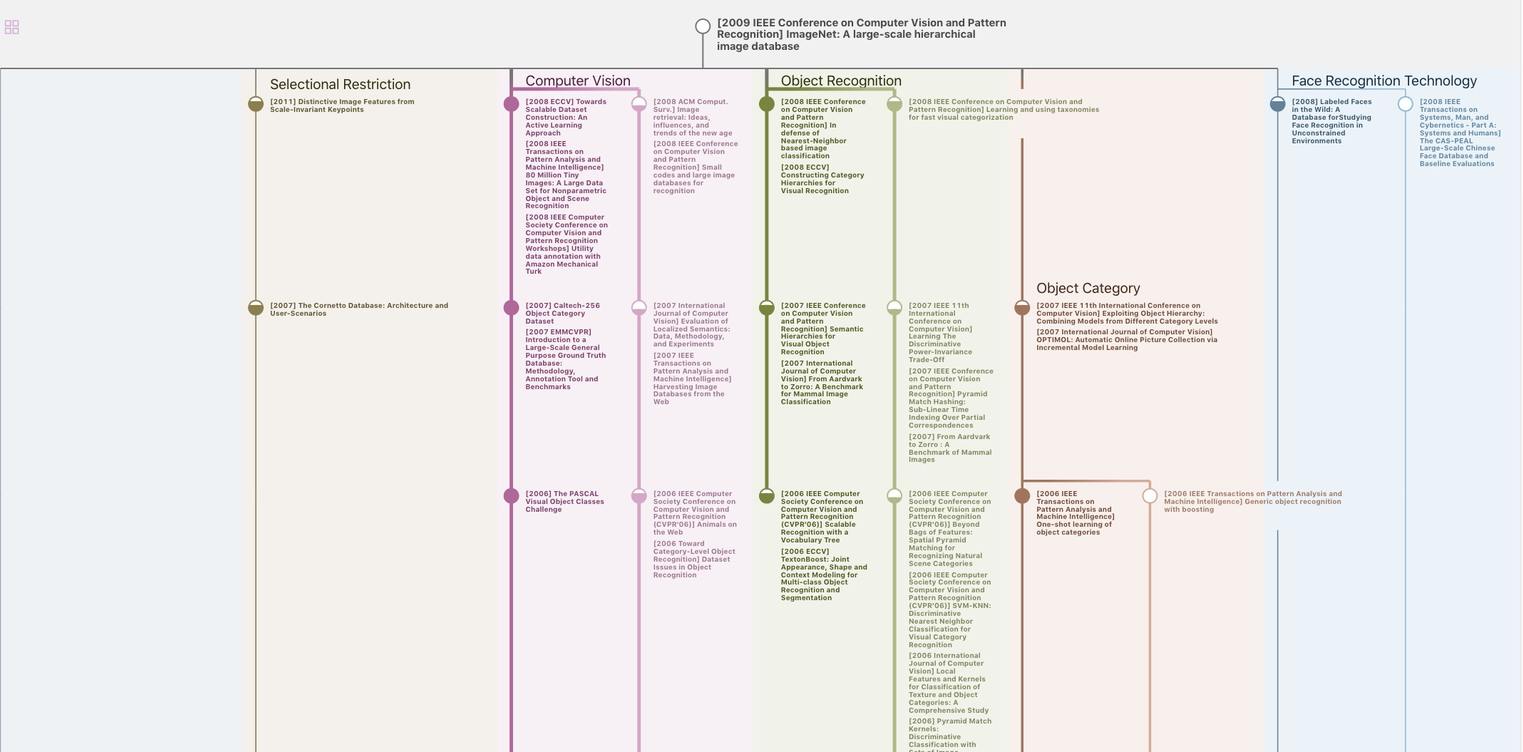
生成溯源树,研究论文发展脉络
Chat Paper
正在生成论文摘要