Globally Optimal Inlier Set Maximization for Atlanta World Understanding
IEEE Transactions on Pattern Analysis and Machine Intelligence(2020)
摘要
In this work, we describe man-made structures via an appropriate structure assumption, called the Atlanta world assumption, which contains a vertical direction (typically the gravity direction) and a set of horizontal directions orthogonal to the vertical direction. Contrary to the commonly used Manhattan world assumption, the horizontal directions in Atlanta world are not necessarily orthogonal to each other. While Atlanta world can encompass a wider range of scenes, this makes the search space much larger and the problem more challenging. Our input data is a set of surface normals, for example, acquired from RGB-D cameras or 3D laser scanners, as well as lines from calibrated images. Given this input data, we propose the first globally optimal method of inlier set maximization for Atlanta direction estimation. We define a novel search space for Atlanta world, as well as its parametrization, and solve this challenging problem using a branch-and-bound (BnB) framework. To alleviate the computational bottleneck in BnB, i.e., the bound computation, we present two bound computation strategies: rectangular bound and slice bound in an efficient measurement domain, i.e., the extended Gaussian image (EGI). In addition, we propose an efficient two-stage method which automatically estimates the number of horizontal directions of a scene. Experimental results with synthetic and real-world datasets have successfully confirmed the validity of our approach.
更多查看译文
关键词
Atlanta frame,RGB-D image,branch-and-bound,global optimization,scene understanding
AI 理解论文
溯源树
样例
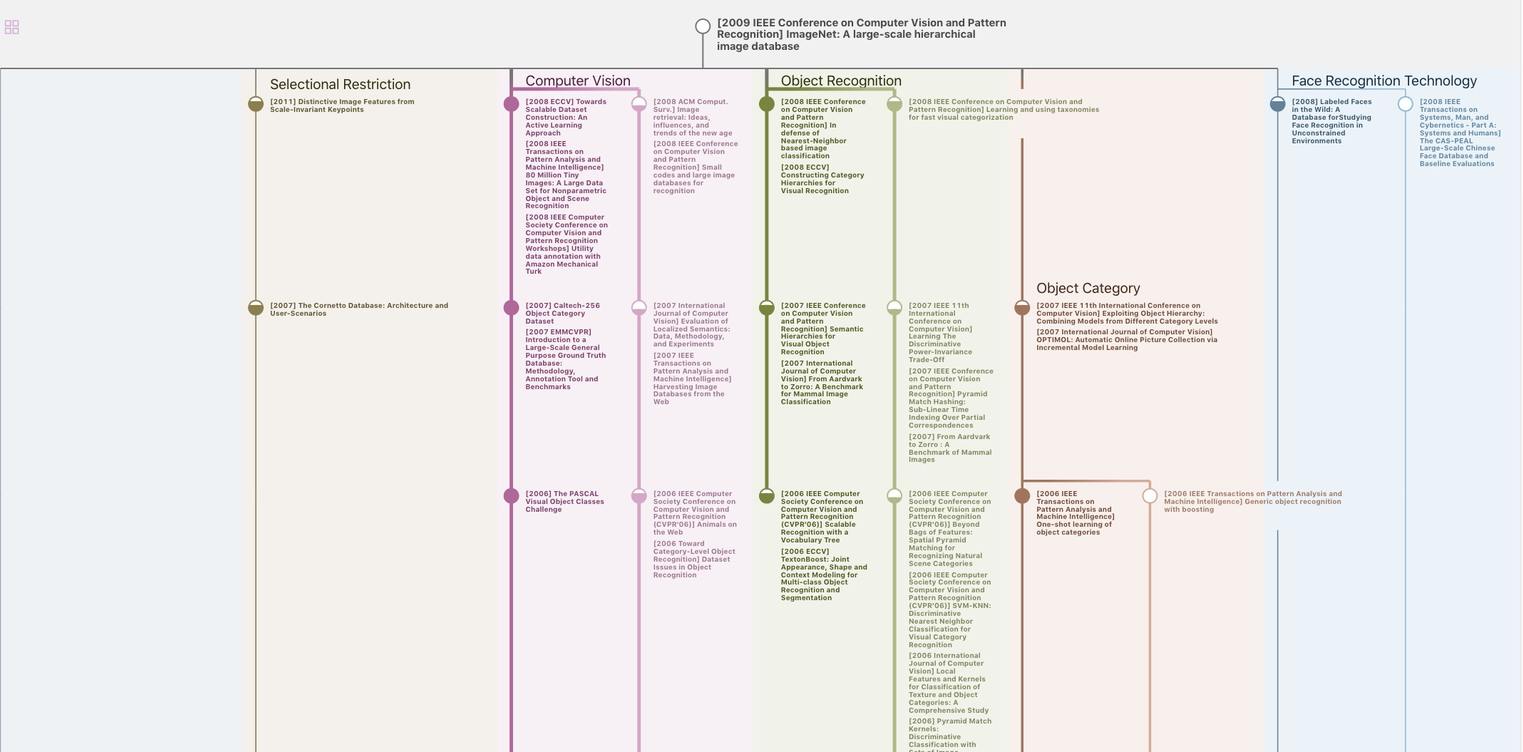
生成溯源树,研究论文发展脉络
Chat Paper
正在生成论文摘要