Bayesian inference for nanopore data analysis.
arXiv: Biological Physics(2019)
摘要
Nanopore sensors detect the substructure of individual molecules from modulations in an ion current as molecules pass through them. In this work, we present the classification of features in the substructure as a case study to illustrate the power of Bayesian inference when analysing nanopore data. A brief introductory section provides an overview of the core concepts, followed by a detailed description of the analysis procedure to facilitate other researchers to add Bayesian inference to their toolbox. Our hybrid approach of a classical peak-finding algorithm and Bayesian model comparison allows the probabilistic classification of features as 0 or 1 bits by calculating relative evidences for two competing models. We correctly classify on average ~ 70% of bits for individual events and use the probabilistic nature of the approach to calculate a cumulative estimate with an accuracy of u003e 94%. The technique presented here is readily extensible to models of the translocation process which can take into account arbitrary molecular designs, our approach may therefore be used to analyse a wide range of features observed in nanopore experiments.
更多查看译文
AI 理解论文
溯源树
样例
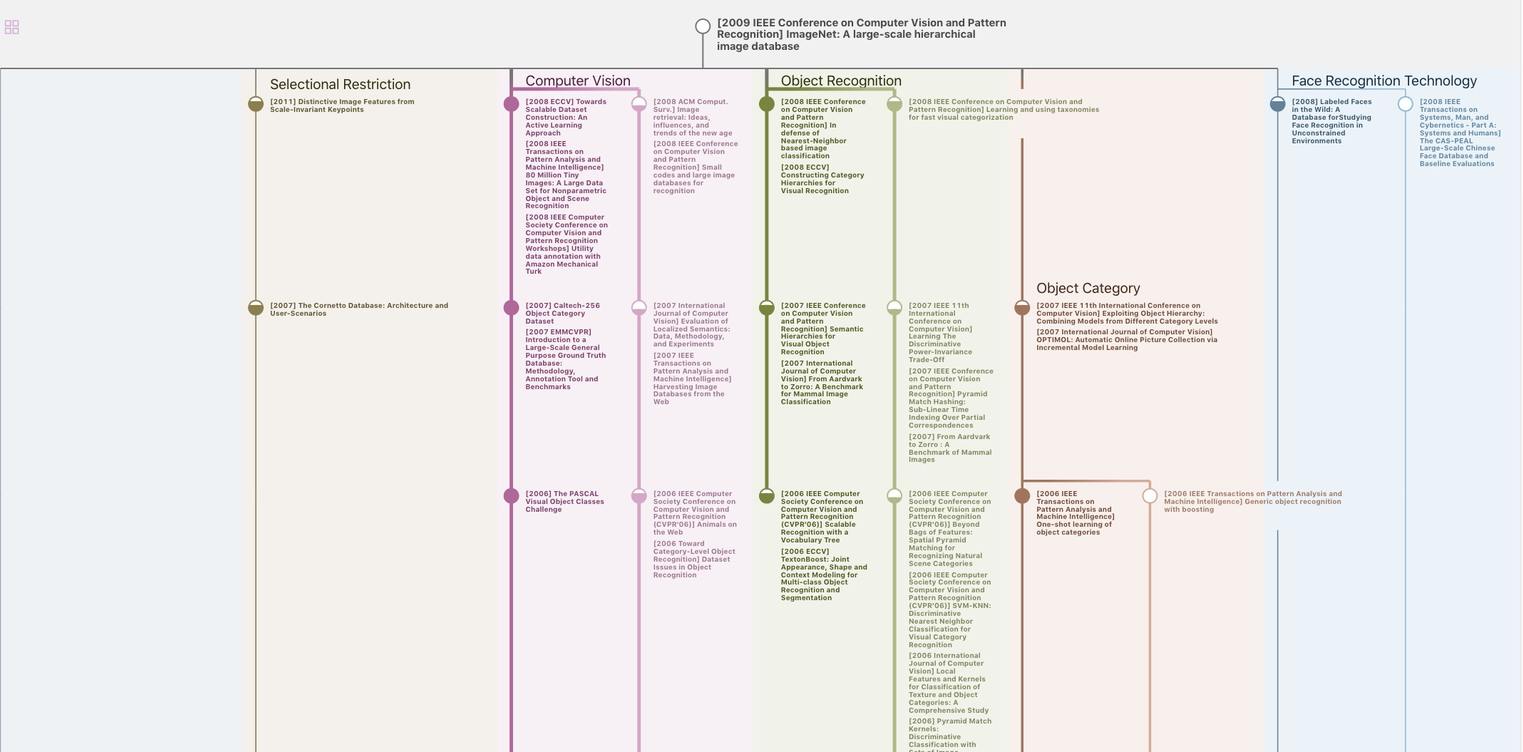
生成溯源树,研究论文发展脉络
Chat Paper
正在生成论文摘要