Can we learn from wrong models?: an experimental study on learning from oversimplified simulation models
Proceedings of the 2018 Winter Simulation Conference(2018)
摘要
Simplifying a model is a necessity in order to create it, but extreme simplification can lead to a wrong - inaccurate or unrepresentative - model. If we end up with such a model, can we still learn from it? This paper investigates possible usefulness and learning outcomes from using wrong models in Simulation. An experiment comparing learning of a model of two different fidelity levels - oversimplified and adequate - is set on a pre/post-test basis utilizing a psychological framework to measure differences within two groups of students. The results suggest that users of the oversimplified version managed to gain a similar level of learning to those using the adequate, while they denoted their model as wrong but still useful for their tasks. Future work will tackle the factors that constitute to creating wrong models and wrong model uses in practice by interviewing simulation experts.
更多查看译文
AI 理解论文
溯源树
样例
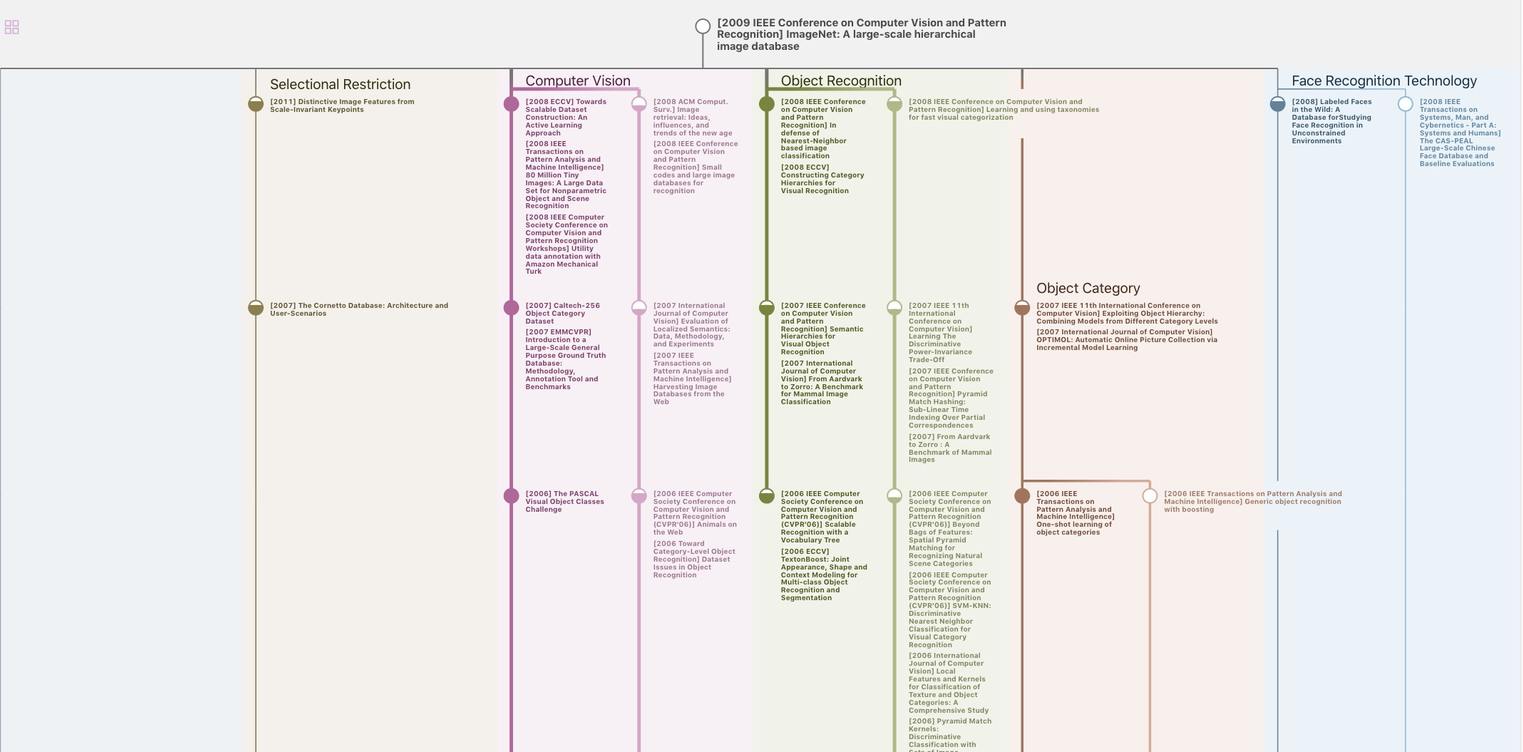
生成溯源树,研究论文发展脉络
Chat Paper
正在生成论文摘要