Learning-Based Outdoor Localization Exploiting Crowd-Labeled WiFi Hotspots
IEEE Transactions on Mobile Computing(2019)
摘要
The ever-expanding scale of WiFi deployments in metropolitan areas has made accurate GPS-free outdoor localization possible by relying solely on the WiFi infrastructure. Nevertheless, neither academic researches nor existing industrial practices seem to provide a satisfactory solution or implementation. In this paper, we propose WOLoc (WiFi-only Outdoor Localization) as a learning-based outdoor localization solution using only WiFi hotspots labeled by crowdsensing. On one hand, we do not take these labels as fingerprints as it is almost impossible to extend indoor localization mechanisms by fingerprinting metropolitan areas. On the other hand, we avoid the over-simplified local synthesis methods (e.g., centroid) that significantly lose the information contained in the labels. Instead, WOLoc adopts a semi-supervised manifold learning approach that accommodates all the labeled and unlabeled data for a given area, and the output concerning the unlabeled part will become the estimated locations for both unknown users and unknown WiFi hotspots. Moreover, WOLoc applies text mining techniques to analyze the SSIDs of hotspots, so as to derive more accurate input to its manifold learning. We conduct extensive experiments in several outdoor areas, and the results have strongly indicated the efficacy of our solution in achieving a meter-level localization accuracy.
更多查看译文
关键词
Wireless fidelity,Urban areas,Databases,Manifolds,Mobile computing,Global Positioning System,Wireless sensor networks
AI 理解论文
溯源树
样例
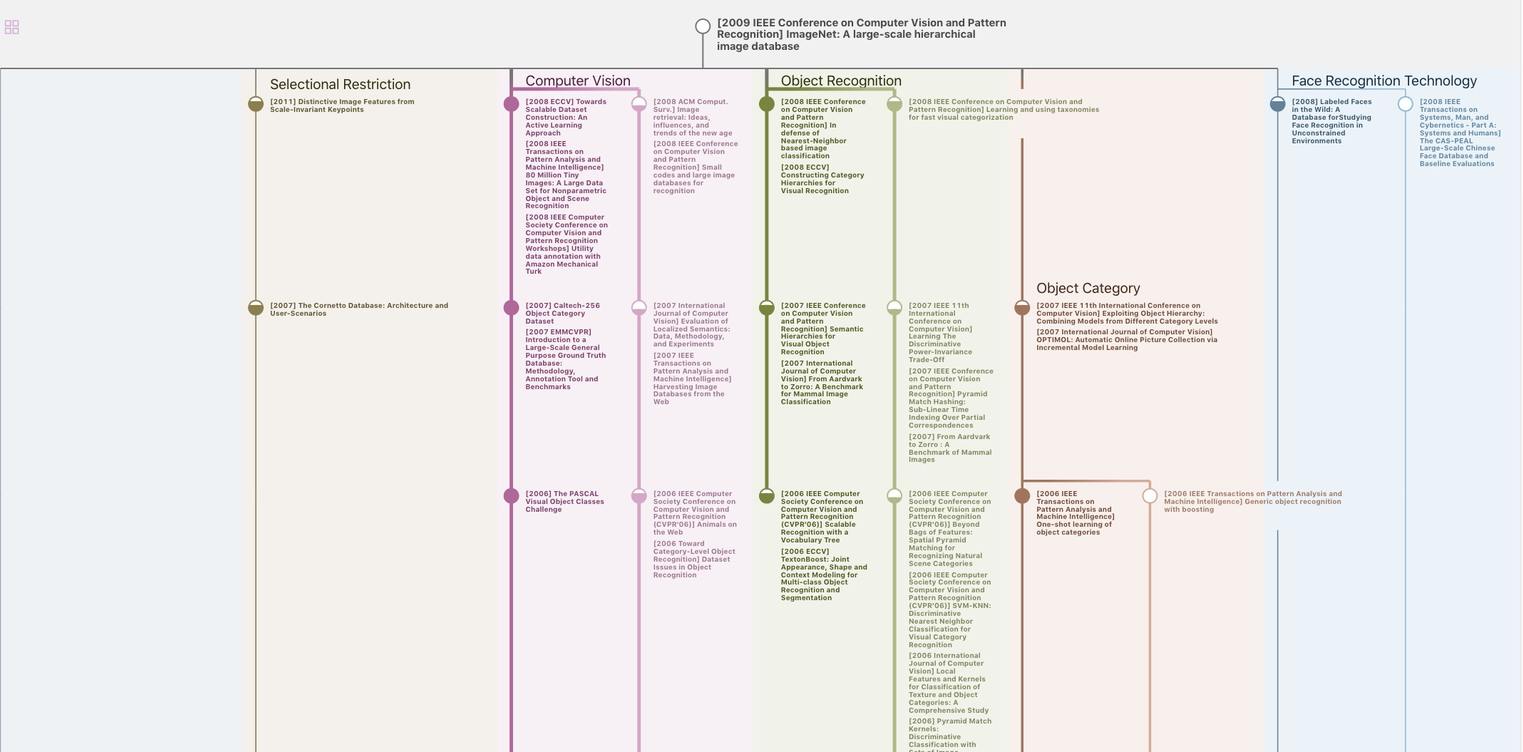
生成溯源树,研究论文发展脉络
Chat Paper
正在生成论文摘要