Probabilistic class hierarchies for multiclass classification.
Journal of Computational Science(2018)
摘要
The improvement in the performance of classifiers has been the focus of attention of many researchers over the last few decades. Obtaining accurate predictions becomes more complicated as the number of classes increases. Most families of classification techniques generate models that define decision boundaries trying to separate the classes as well as possible. As an alternative, in this paper, we propose to hierarchically decompose the original multiclass problem by reducing the number of classes involved in each local subproblem. This is done by deriving a similarity matrix from the misclassification errors given by a first classifier that is learned for this, and then, using the similarity matrix to build a tree-like hierarchy of specialized classifiers. Then, we present two approaches to solve the multiclass problem: the first one traverses the tree of classifiers in a top-down manner similar to the way some hierarchical classification methods do for dealing with hierarchical domains; the second one is inspired in the way probabilistic decision trees compute class membership probabilities. To improve the efficiency of our methods, we propose a criterion to reduce the size of the hierarchy. We experimentally evaluate all of the proposals on a collection of multiclass datasets showing that, in general, the generated classifier hierarchies outperform the original (flat) multiclass classification.
更多查看译文
关键词
Multiclass classification,Class hierarchy inference,Hierarchy of classifiers
AI 理解论文
溯源树
样例
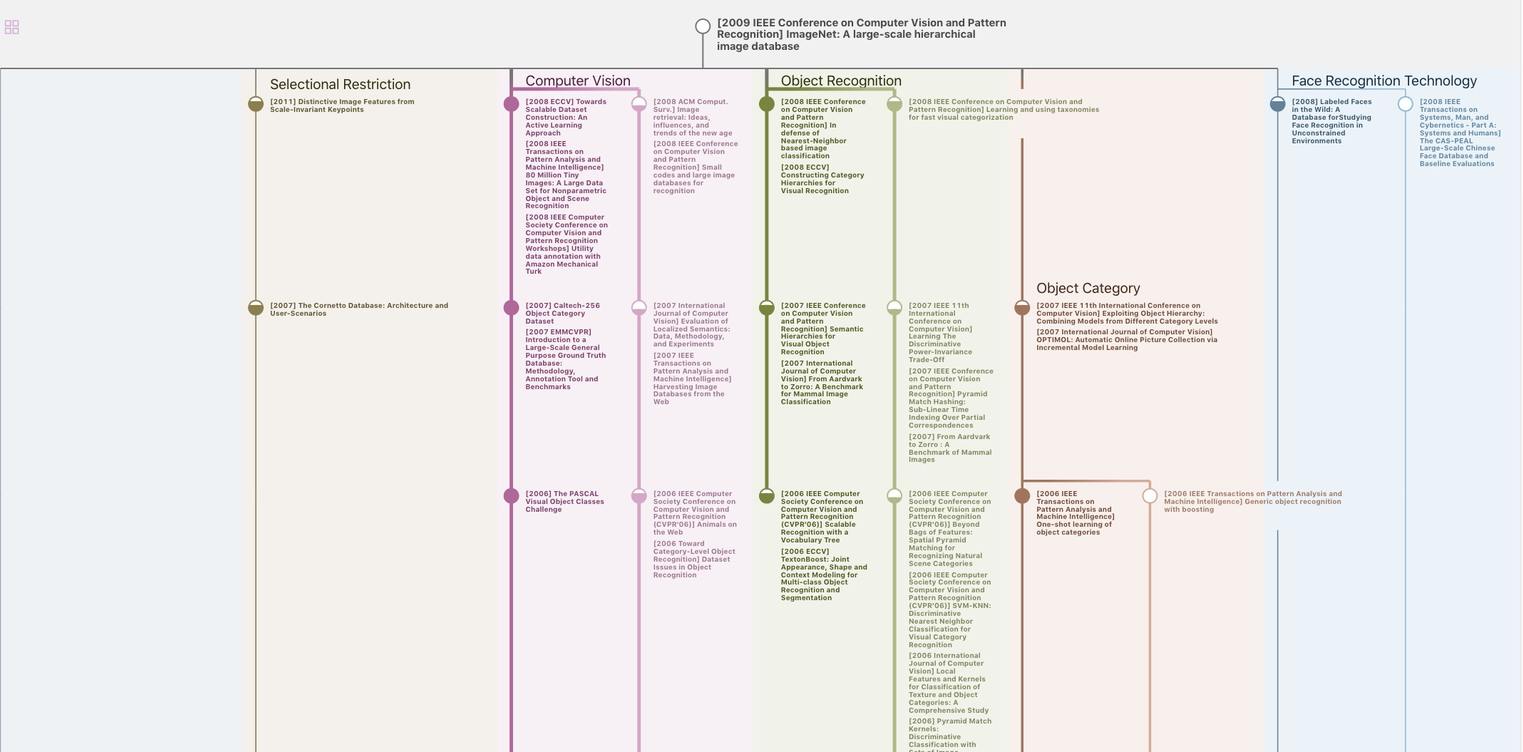
生成溯源树,研究论文发展脉络
Chat Paper
正在生成论文摘要