Modeling and Mining Domain Shared Knowledge for Sentiment Analysis.
ACM Trans. Inf. Syst.(2017)
摘要
Sentiment classification aims to automatically predict sentiment polarity (e.g., positive or negative) of user generated sentiment data (e.g., reviews, blogs). In real applications, these user-generated sentiment data can span so many different domains that it is difficult to label the training data for all of them. Therefore, we study the problem of sentiment classification adaptation task in this article. That is, a system is trained to label reviews from one source domain but is meant to be used on the target domain. One of the biggest challenges for sentiment classification adaptation task is how to deal with the problem when two data distributions between the source domain and target domain are significantly different from one another. However, our observation is that there might exist some domain shared knowledge among certain input dimensions of different domains. In this article, we present a novel method for modeling and mining the domain shared knowledge from different sentiment review domains via a joint non-negative matrix factorization–based framework. In this proposed framework, we attempt to learn the domain shared knowledge and the domain-specific information from different sentiment review domains with several various regularization constraints. The advantage of the proposed method can promote the correspondence under the topic space between the source domain and the target domain, which can significantly reduce the data distribution gap across two domains. We conduct extensive experiments on two real-world balanced data sets from Amazon product reviews for sentence-level and document-level binary sentiment classification. Experimental results show that our proposed approach significantly outperforms several strong baselines and achieves an accuracy that is competitive with the most well-known methods for sentiment classification adaptation.
更多查看译文
关键词
Information Retrieval, Natural Language Processing, Sentiment Analysis
AI 理解论文
溯源树
样例
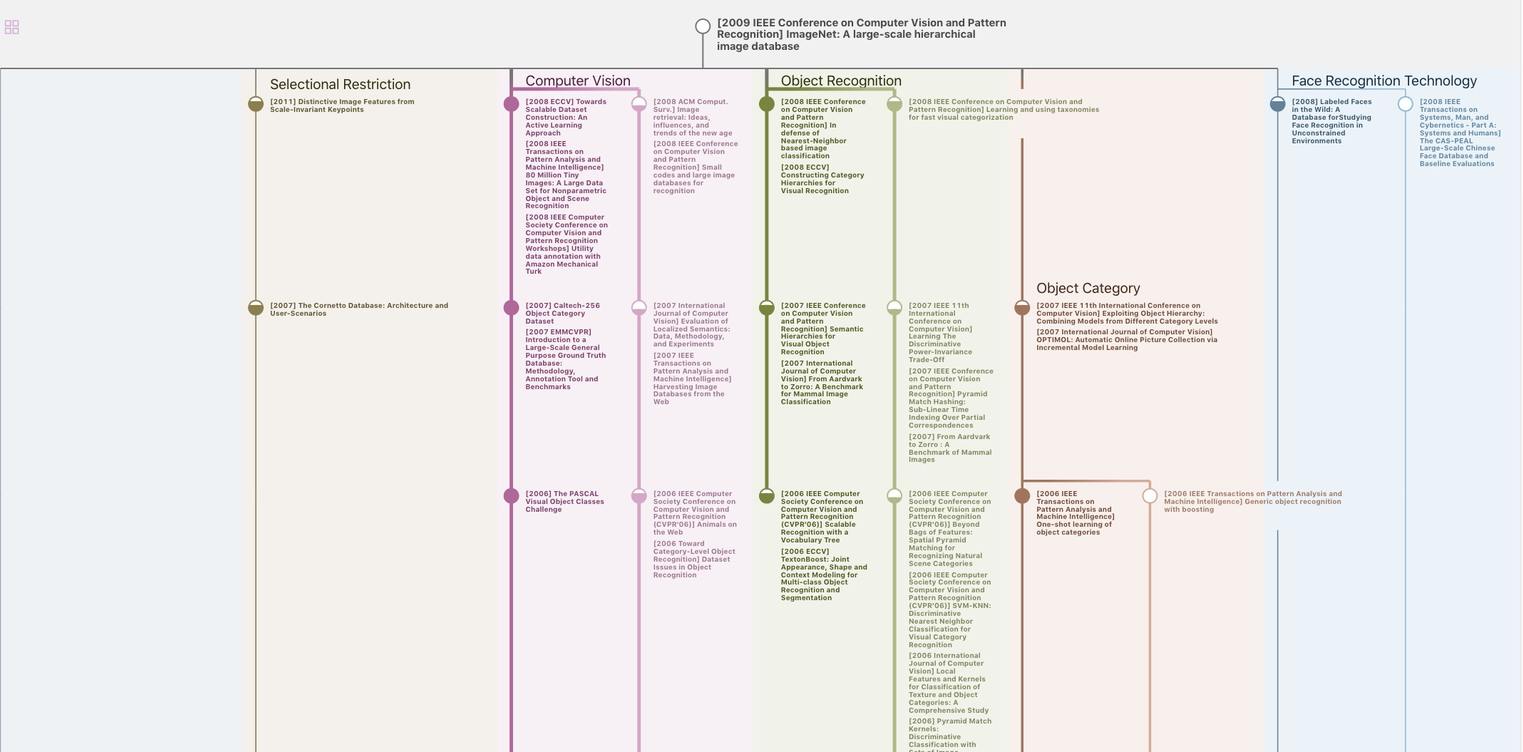
生成溯源树,研究论文发展脉络
Chat Paper
正在生成论文摘要