O77 Deep learning for EEG classification for outcome prediction of postanoxic coma
Clinical Neurophysiology(2017)
摘要
Objectives Electroencephalography is increasingly used for outcome prediction of comatose patients after cardiac arrest. Here, we explore a deep learning approach using convolutional neural networks. Methods Using epochs from continuous EEGs from 287 patients at 12 h and 399 patients at 24 h after cardiac arrest, we trained and validated a convolutional neural network with raw EEG data (longitudinal bipolar montage). The network consisted of one convolutional layer and two fully-connected neuronal layers. Clinical outcome at six months was classified by the Cerebral Performance Category scale (CPC), dichotomized as good (CPC 1–2) or poor (CPC 3–5). In 5 min artifact-free epochs, partitioned into 10 s snippets, we trained the network using data from 80% of the patients. Validation was performed in the remaining patients. The network was implemented in Python using Keras and Theano and a CUDA-enabled GPU. Results Outcome prediction was most accurate at 12 h after cardiac arrest, with a sensitivity of 58% at 100% specificity for the prediction of poor outcome. Good outcome could be predicted at 12 h with a sensitivity of 58% at a specificity of 97%. Training of the network took 50 min, classification was realized within 1 s. Discussion Deep learning allows outcome prediction with higher accuracies than visual EEG assessment, however it does not reveal which EEG features are the most discriminative. Conclusions We present a convolutional neural network for objective and reliable outcome prediction after cardiac arrest. Significance Deep learning might be an important future tool for neuro-monitoring in the ICU.
更多查看译文
AI 理解论文
溯源树
样例
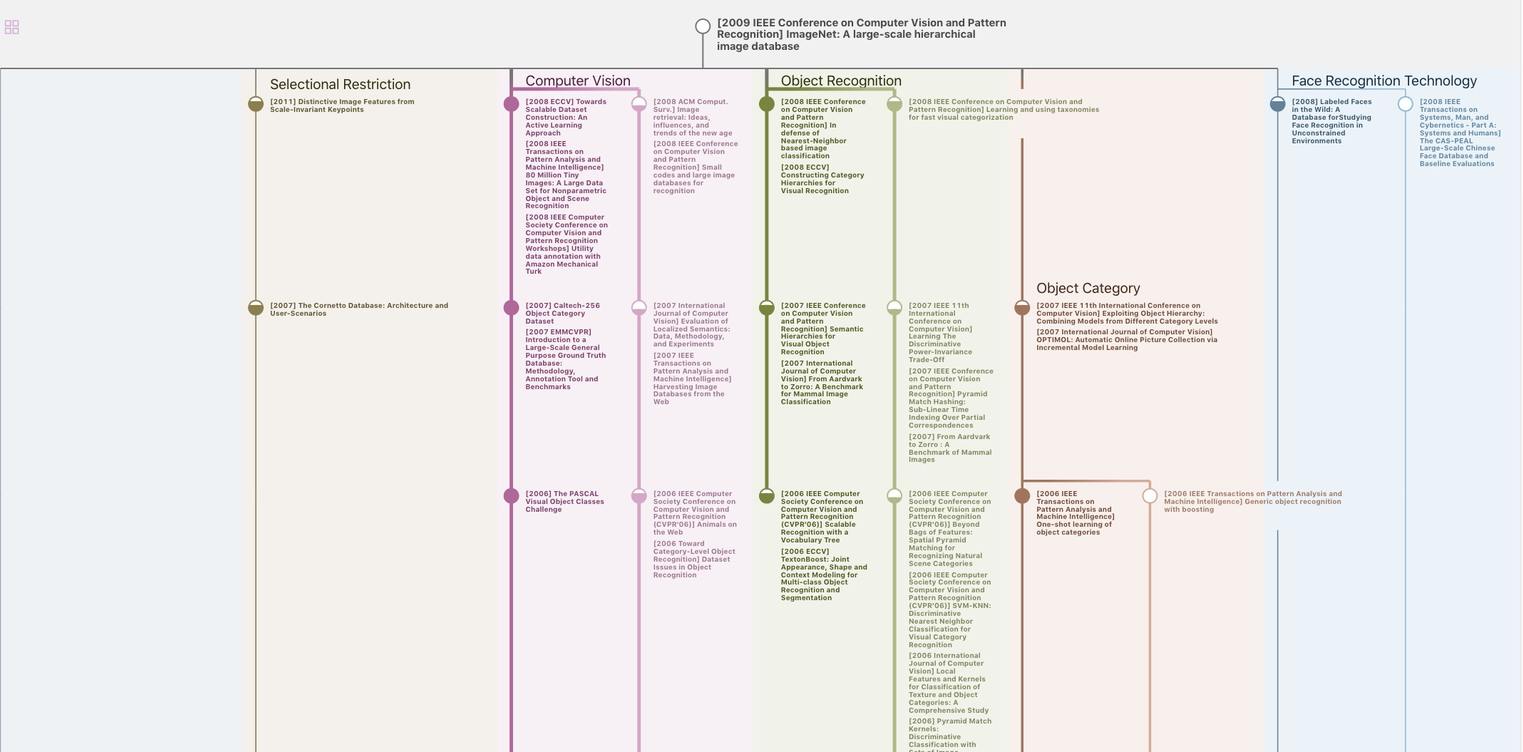
生成溯源树,研究论文发展脉络
Chat Paper
正在生成论文摘要