VLSI Architectures for the Restricted Boltzmann Machine.
JETC(2017)
摘要
Neural network (NN) systems are widely used in many important applications ranging from computer vision to speech recognition. To date, most NN systems are processed by general processing units like CPUs or GPUs. However, as the sizes of dataset and network rapidly increase, the original software implementations suffer from long training time. To overcome this problem, specialized hardware accelerators are needed to design high-speed NN systems. This article presents an efficient hardware architecture of restricted Boltzmann machine (RBM) that is an important category of NN systems. Various optimization approaches at the hardware level are performed to improve the training speed. As-soon-as-possible and overlapped-scheduling approaches are used to reduce the latency. It is shown that, compared with the flat design, the proposed RBM architecture can achieve 50% reduction in training time. In addition, an on-the-fly computation scheme is also used to reduce the storage requirement of binary and stochastic states by several hundreds of times. Then, based on the proposed approach, a 784-2252 RBM design example is developed for MNIST handwritten digit recognition dataset. Analysis shows that the VLSI design of RBM achieves significant improvement in training speed and energy efficiency as compared to CPU/GPU-based solution.
更多查看译文
关键词
Restricted Boltzmann machine (RBM),neural network (NN),VLSI,reduced-latency,high-speed,memory reduction,overlapped-scheduling
AI 理解论文
溯源树
样例
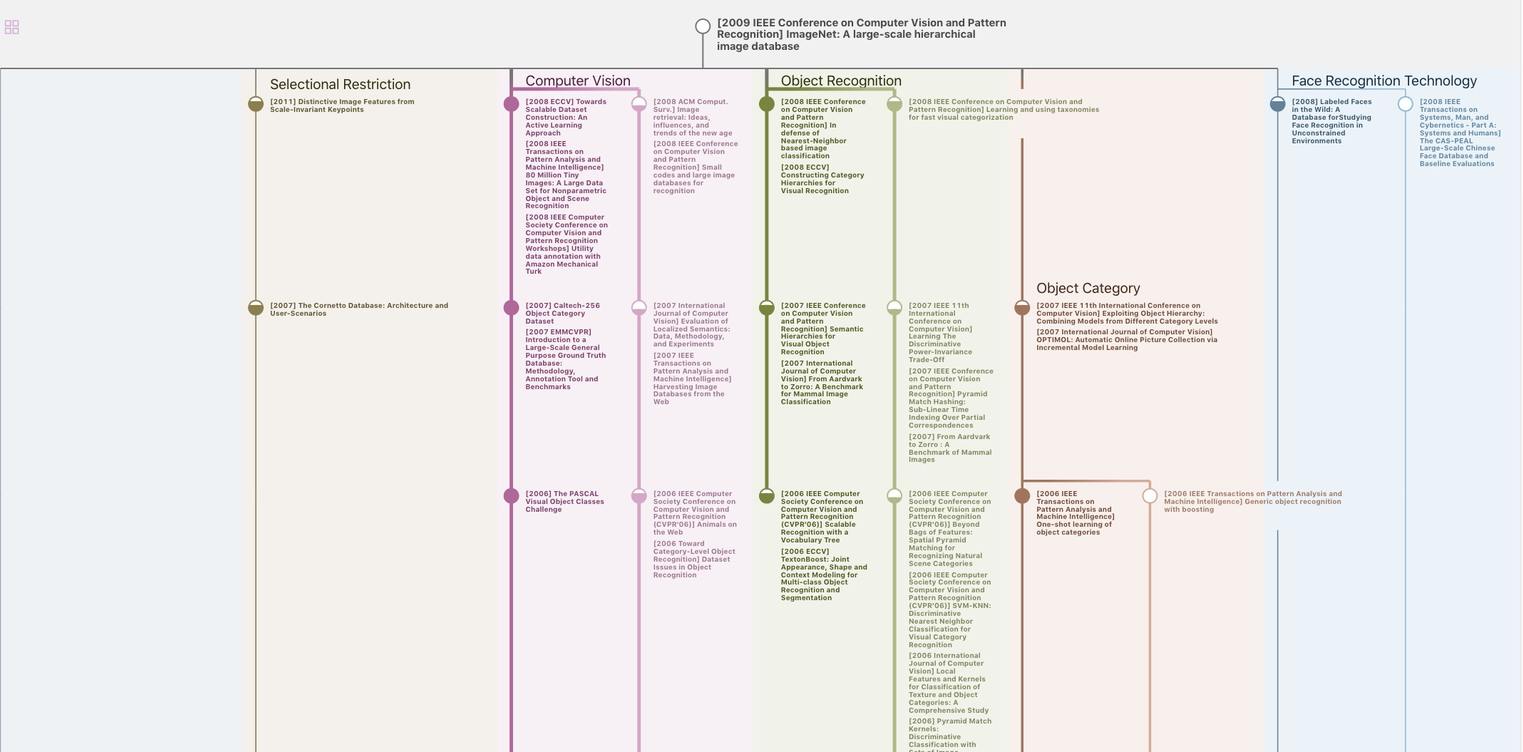
生成溯源树,研究论文发展脉络
Chat Paper
正在生成论文摘要