Time-Frequency Mask-based Speech Enhancement using Convolutional Generative Adversarial Network.
Asia-Pacific Signal and Information Processing Association Annual Summit and Conference(2018)
摘要
Speech Enhancement (SE) system deals with improving the perceptual quality and preserving the speech intelligibility of the noisy mixture. The Time-Frequency (T-F) masking-based SE using the supervised learning algorithm, such as a Deep Neural Network (DNN), has outperformed the traditional SE techniques. However, the notable difference observed between the oracle mask and the predicted mask, motivates us to explore different deep learning architectures. In this paper, we propose to use a Convolutional Neural Network (CNN)-based Generative Adversarial Network (GAN) for inherent mask estimation. GAN takes an advantage of the adversarial optimization, an alternative to the other Maximum Likelihood (ML) optimization-based architectures. We also show the need for supervised T-F mask estimation for effective noise suppression. Experimental results demonstrate that the proposed T-F mask-based SE significantly outperforms the recently proposed end-to-end SEGAN and a GAN-based Pix2Pix architecture. The performance evaluation in terms of both the predicted mask and the objective measures, dictates the improvement in the speech quality, while simultaneously reducing the speech distortion observed in the noisy mixture.
更多查看译文
关键词
speech enhancement,generative adversarial network,convolutional neural network,inherent mask estimation
AI 理解论文
溯源树
样例
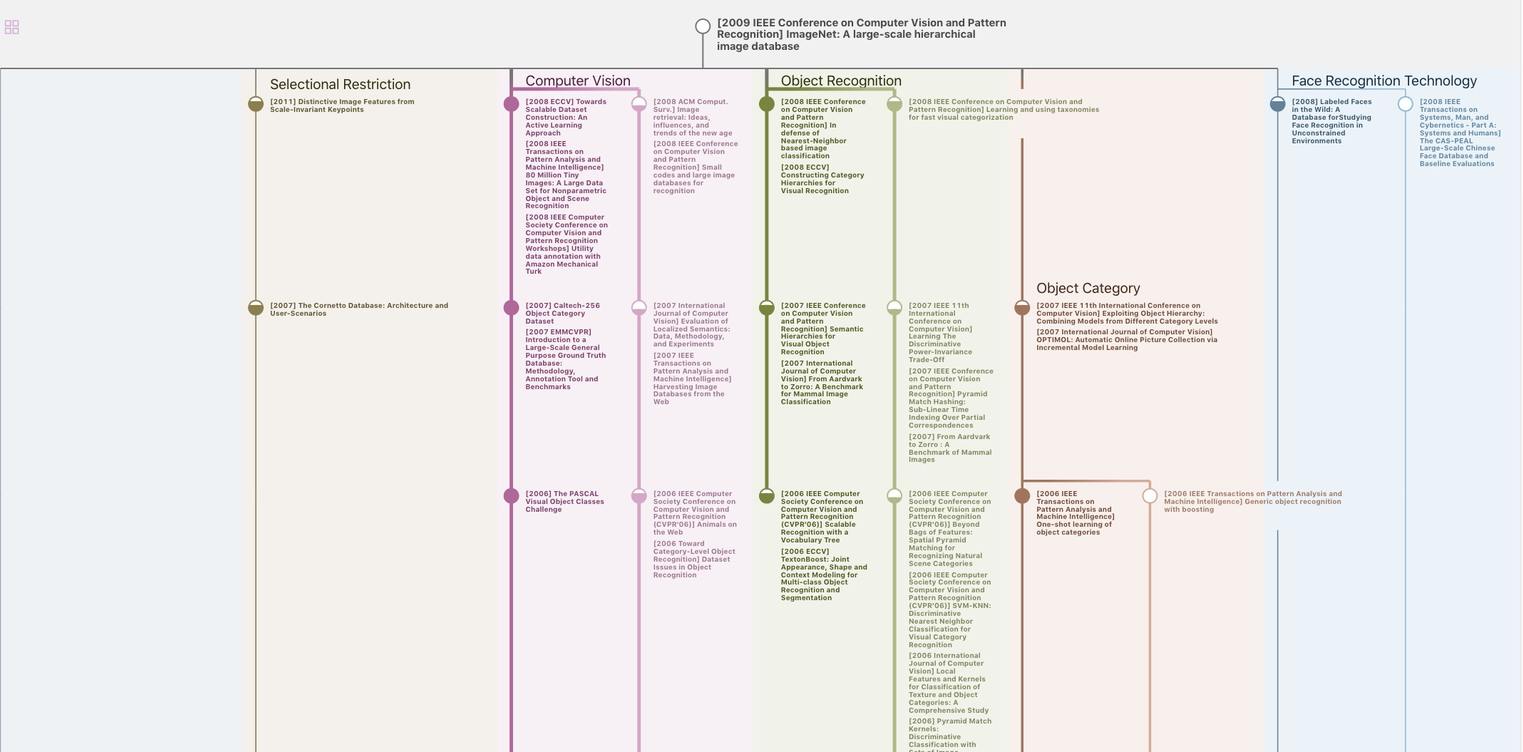
生成溯源树,研究论文发展脉络
Chat Paper
正在生成论文摘要