Change Detection in High Resolution Satellite Images Using an Ensemble of Convolutional Neural Networks
2018 Asia-Pacific Signal and Information Processing Association Annual Summit and Conference (APSIPA ASC)(2018)
摘要
In this paper, we propose a novel change detection algorithm for high resolution satellite images using convolutional neural networks (CNNs), which does not require any preprocessing, such as ortho-rectification and classification. When analyzing multi-temporal satellite images, it is crucial to distinguish viewpoint or color variations of an identical object from actual changes. Especially in urban areas, the registration difficulty due to high-rise buildings makes conventional change detection techniques unreliable, if they are not combined with preprocessing schemes using digital surface models or multi-spectral information. We design three encoder-decoder-structured CNNs, which yield change maps from an input pair of RGB satellite images. For the supervised learning of these CNNs, we construct a large fully-labeled dataset using Google Earth images taken in different years and seasons. Experimental results demonstrate that the trained CNNs detect actual changes successfully, even though image pairs are neither perfectly registered nor color-corrected. Furthermore, an ensemble of the three CNNs provides excellent performance, outperforming each individual CNN.
更多查看译文
关键词
Decoding,Image segmentation,Satellites,Image color analysis,Urban areas,Satellite broadcasting,Feature extraction
AI 理解论文
溯源树
样例
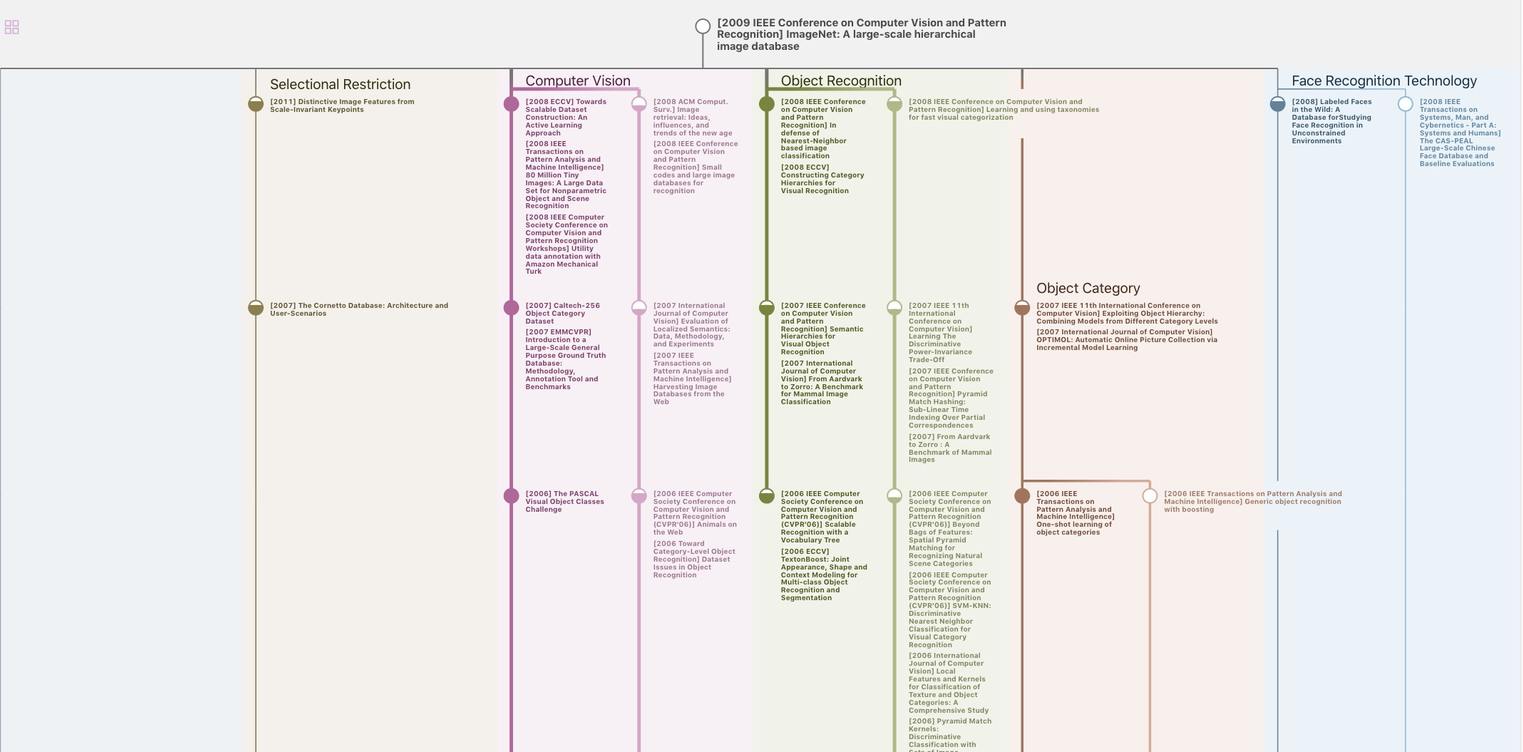
生成溯源树,研究论文发展脉络
Chat Paper
正在生成论文摘要