A fast attribute reduction method for large formal decision contexts
International Journal of Approximate Reasoning(2019)
摘要
Attribute reduction in formal decision contexts is an important issue in formal concept analysis, which can help us to discover the knowledge hidden in formal decision contexts. However, most reduction methods in formal decision contexts are very time-consuming due to two main problems. The first is that one needs to construct a discernibility matrix after generating all the formal concepts of formal decision contexts. This is not an easy task because it requires much more storage space and computation time. Another problem is that most reduction methods are based on the Boolean reasoning and the computational complexity of which is exponential in the worst case. To overcome these problems, we propose a new attribute reduction method for formal decision contexts in this paper. A more simplified discernibility matrix which does not need to generate all the formal concepts is first constructed. It shows that the storage space and computation time are far less than the original method. Furthermore, different from the Boolean reasoning method, an approximation algorithm for obtaining a minimum reduct in formal decision contexts based on graph theory is designed. Finally, experiments are carried out to verify the effectiveness of the proposed method. The results, on 22 large data sets, demonstrate that the proposed method not only produces smaller subset of attributes but also has better performance in both storage space and speed.
更多查看译文
关键词
Attribute reduction,Concept lattices,Formal decision contexts,Graph theory
AI 理解论文
溯源树
样例
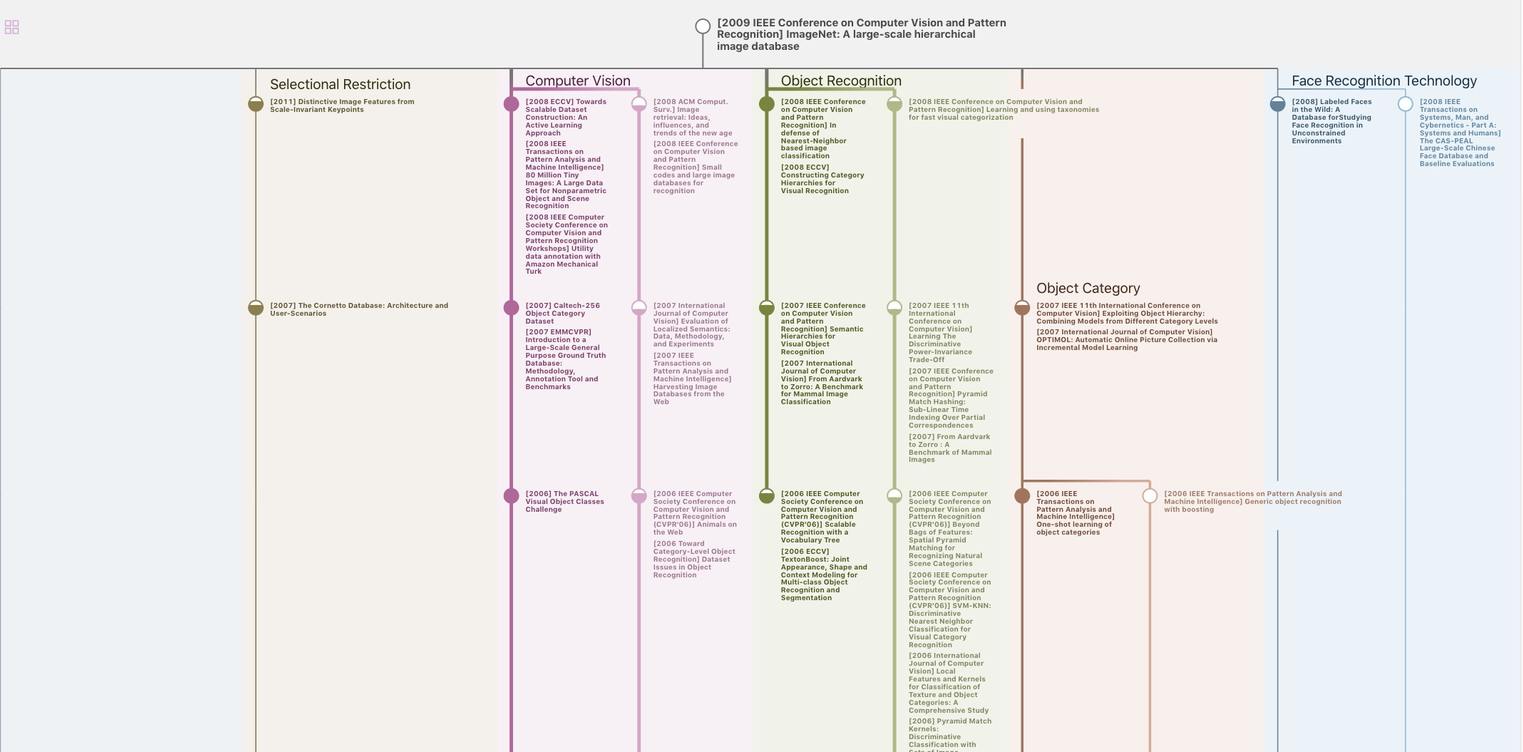
生成溯源树,研究论文发展脉络
Chat Paper
正在生成论文摘要