Deep Convolutional Transfer Learning Network: A New Method for Intelligent Fault Diagnosis of Machines with Unlabeled Data
IEEE Transactions on Industrial Electronics(2019)
摘要
The success of intelligent fault diagnosis of machines relies on the following two conditions: 1) labeled data with fault information are available; and 2) the training and testing data are drawn from the same probability distribution. However, for some machines, it is difficult to obtain massive labeled data. Moreover, even though labeled data can be obtained from some machines, the intelligent fault diagnosis method trained with such labeled data possibly fails in classifying unlabeled data acquired from the other machines due to data distribution discrepancy. These problems limit the successful applications of intelligent fault diagnosis of machines with unlabeled data. As a potential tool, transfer learning adapts a model trained in a source domain to its application in a target domain. Based on the transfer learning, we propose a new intelligent method named deep convolutional transfer learning network (DCTLN). A DCTLN consists of two modules: condition recognition and domain adaptation. The condition recognition module is constructed by a one-dimensional (1-D) convolutional neural network (CNN) to automatically learn features and recognize health conditions of machines. The domain adaptation module facilitates the 1-D CNN to learn domain-invariant features by maximizing domain recognition errors and minimizing the probability distribution distance. The effectiveness of the proposed method is verified using six transfer fault diagnosis experiments.
更多查看译文
关键词
Fault diagnosis,Convolution,Feature extraction,Training,Testing,Probability distribution,Learning systems
AI 理解论文
溯源树
样例
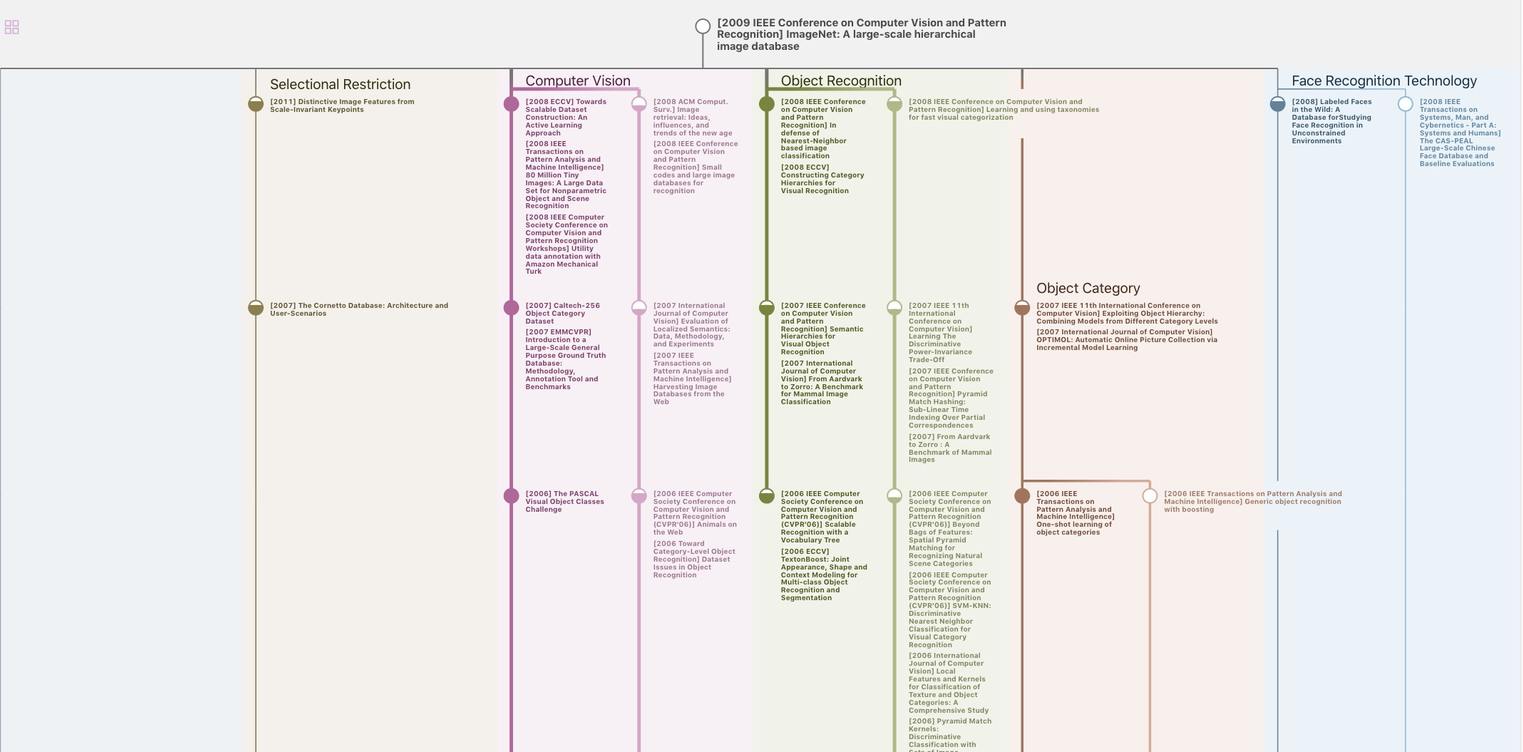
生成溯源树,研究论文发展脉络
Chat Paper
正在生成论文摘要