Embedding the single-cell sample manifold to reveal insights into cancer pathogenesis and disease heterogeneity
bioRxiv(2018)
摘要
Single-cell data are now being collected in large quantities across multiple samples and gene profiling runs. This introduces the need for computational methods that can compare and stratify samples that are represented themselves as complex, high-dimensional objects. We introduce PhEMD as an analytical approach that can be used for this purpose. PhEMD uses Earth Mover9s Distance (EMD), a distance between probability distributions that is sensitive to differences at multiple levels of granularity, in order to compute an accurate measure of dissimilarity between single-cell samples. PhEMD then generates a low-dimensional embedding of the samples based on this dissimilarity. We demonstrate the utility of the PhEMD sample embedding by using it to subtype melanoma and clear-cell renal cell carcinomas based on their immune cell profiles. These analyses reveal sources of inter-sample heterogeneity that have potentially clinically actionable implications, given the recent adoption of immunotherapy as an effective treatment for these cancers. We also apply PhEMD to a newly-generated 300-sample CyTOF drug screen experiment, where the effects of 233 kinase inhibitors are measured at the single-cell resolution in 33 protein dimensions. In doing so, we find that PhEMD reveals novel insights into the effects of small-molecule inhibitors on breast cancer cell subpopulations undergoing epithelial-to-mesenchymal transition. Finally, by leveraging the Nystrom extension method for diffusion maps, we demonstrate that the results of PhEMD can be integrated with other data sources and data types to predict the single-cell phenotypes of samples not directly profiled. Our analyses demonstrate that PhEMD is highly scalable and compatible with leading batch effect correction techniques, allowing for the simultaneous comparison of many single-cell samples.
更多查看译文
AI 理解论文
溯源树
样例
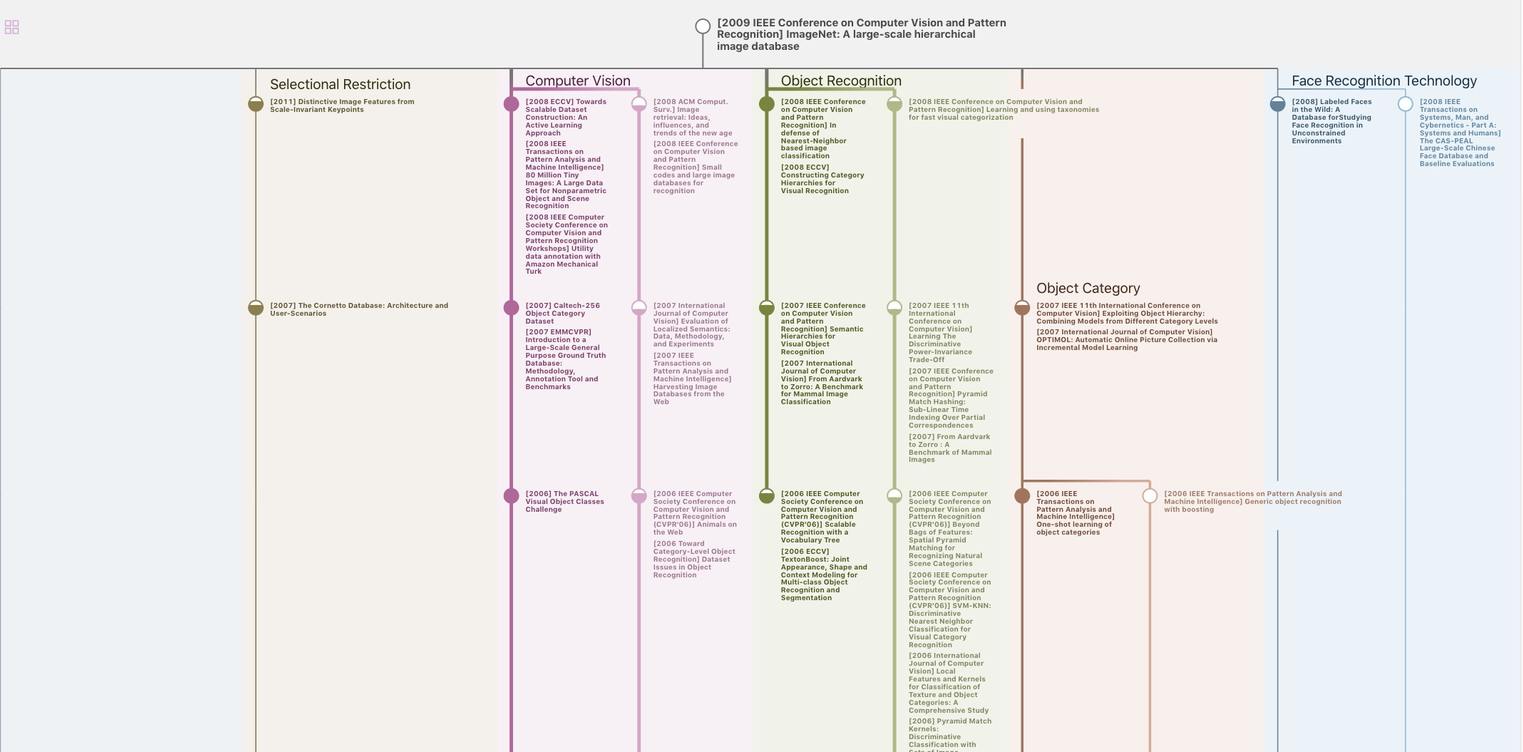
生成溯源树,研究论文发展脉络
Chat Paper
正在生成论文摘要