SNPs-based Hypertension Disease Detection via Machine Learning Techniques
2018 24th International Conference on Automation and Computing (ICAC)(2018)
摘要
Machine learning and data mining techniques have recently gained more popularity in the field of Medical diagnosis, especially for the analysis of the human genome. One of the most significant sources of human genome variation is Single Nucleotide Polymorphisms (SNPs), which have been associated with multiple human diseases. Several techniques have been developed for distinguishing between affected and healthy samples of SNP data. In this study, conditional mutual information maximisation (CMIM) has been employed in order to identify a subset of the most informative SNPs to be used in with various classifications algorithms for the detection of hypertension disease. Five classification algorithms have been evaluated, namely k-Nearest Neighbours (KNN), Artificial Neural Networks (ANN), Naive Bayes (NB), Linear Discriminant Analysis (LDA) and Support Vector Machines (SVM), along with their combination into an unweighted majority voting ensemble classification scheme. The experimental evaluation of the proposed approach via supervised classification experiments showed that the ensemble approach using the SVM, 5-NN, and NB classifiers achieves the highest classification accuracy (93.21%) and F1 score (91.72%), demonstrating the suitability of the proposed approach for the detection of hypertension disease from SNPs data.
更多查看译文
关键词
Hypertension disease,SNPs,machine learning,feature selection,ensemble classification
AI 理解论文
溯源树
样例
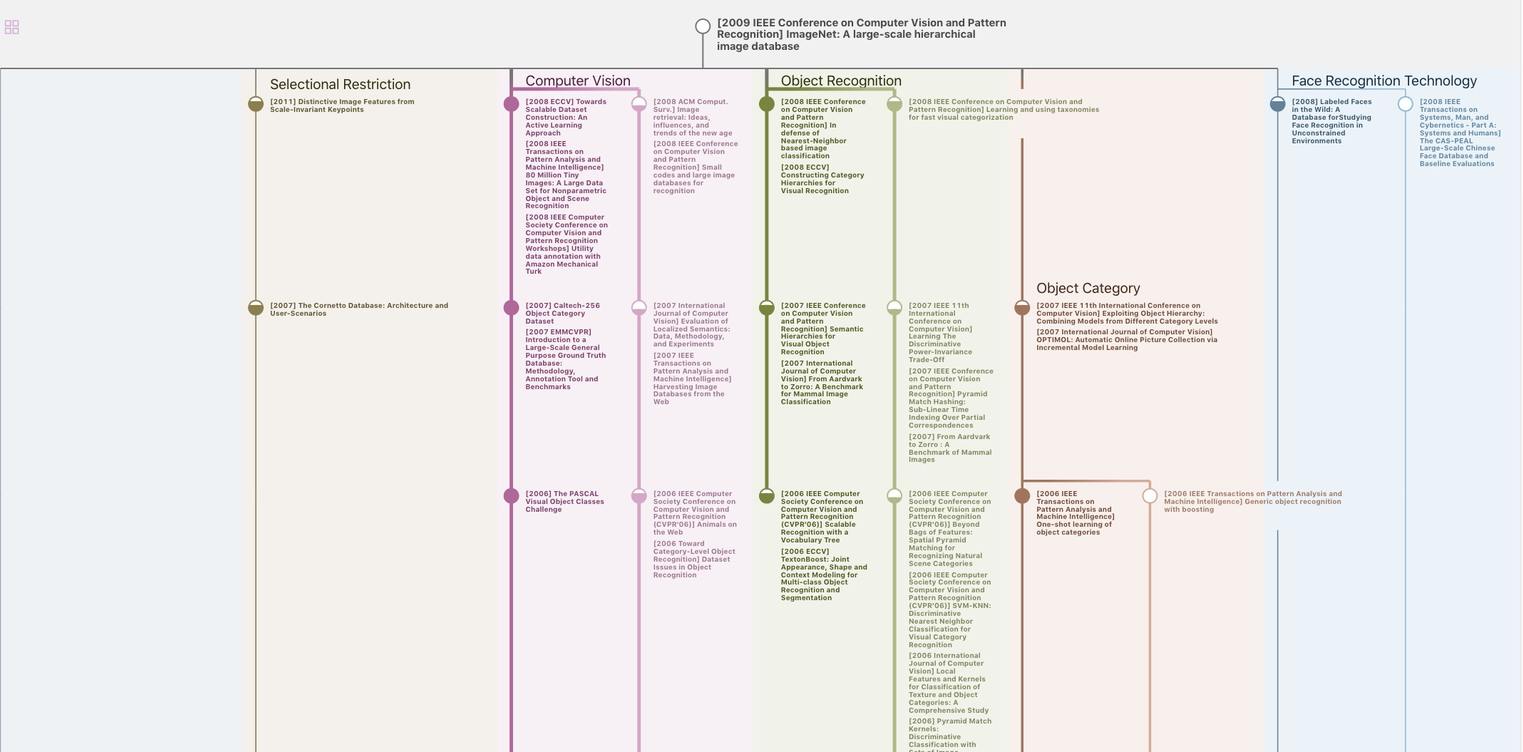
生成溯源树,研究论文发展脉络
Chat Paper
正在生成论文摘要