Deep Convolutional modeling of human face selective columns reveals their role in pictorial face representation
bioRxiv(2018)
摘要
Despite the massive accumulation of systems neuroscience findings, their functional meaning remains tentative, largely due to the absence of realistically performing models. The discovery that deep convolutional networks achieve human performance in realistic tasks offers fresh opportunities for such modeling. Here we show that the face-space topography of face-selective columns recorded intra-cranially in 32 patients significantly matches that of a DCNN having human-level face recognition capabilities. Three modeling aspects converge in pointing to a role of human face areas in pictorial rather than person identification: First, the match was confined to intermediate layers of the DCNN. Second, identity preserving image manipulations abolished the brain to DCNN correlation. Third, DCNN neurons matching face-column tuning displayed view-point selective receptive fields. Our results point to a convergent evolution of pattern similarities in biological and artificial face perception. They demonstrate DCNNs as a powerful modeling approach for deciphering the function of human cortical networks.
更多查看译文
AI 理解论文
溯源树
样例
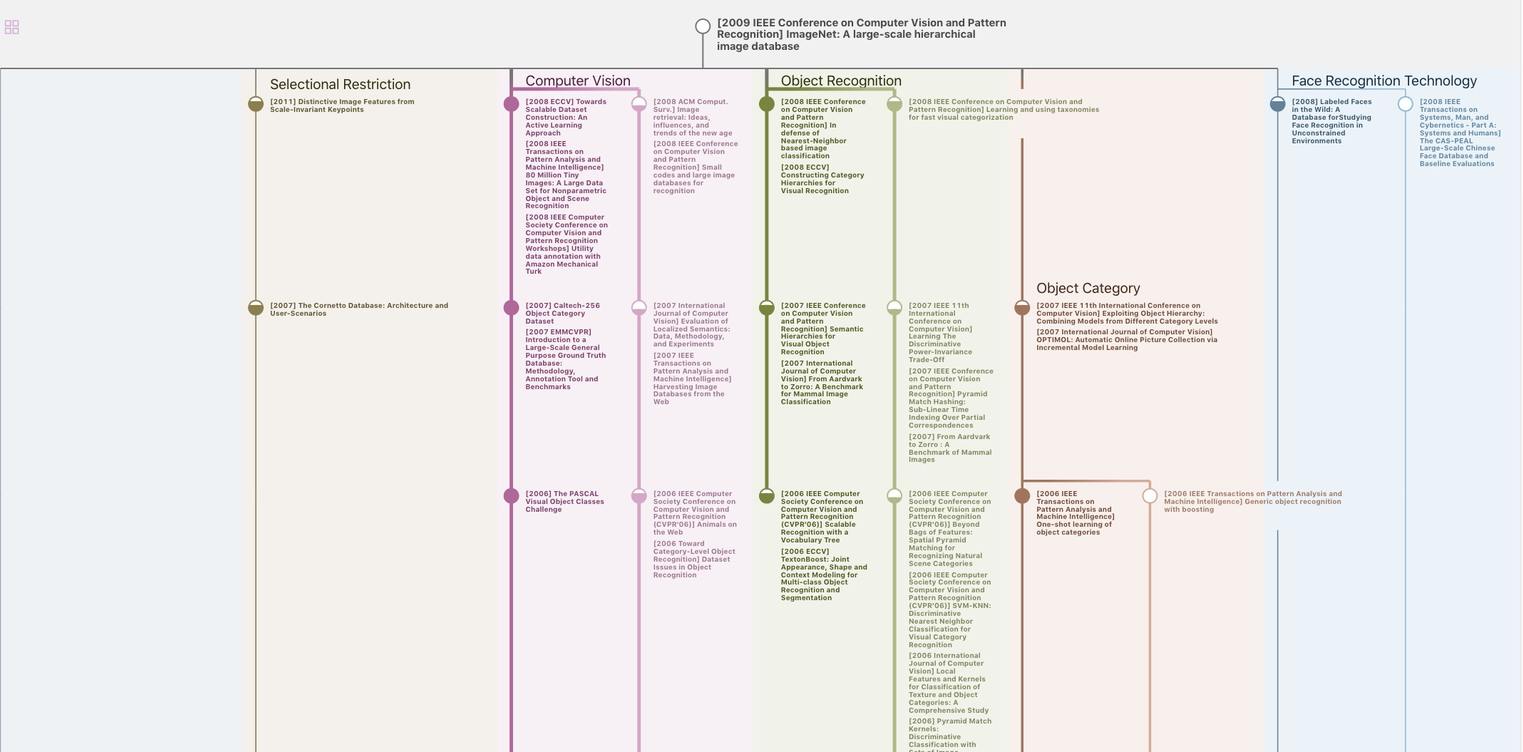
生成溯源树,研究论文发展脉络
Chat Paper
正在生成论文摘要