Learning Salient Features for Speech Emotion Recognition Using CNN
2018 First Asian Conference on Affective Computing and Intelligent Interaction (ACII Asia)(2018)
摘要
In this work, a framework based on Convolution Neural Network (CNN) is proposed for speech emotion recognition (SER). We focus on extracting the most salient frames via the proposed CNN structure from the entire frame sequence to represent the utterance. A particular pooling method named global k-max pooling is utilized in our CNN structure (GCNN) to achieve the above objective. We implemented SER experiments on Interactive Emotional Dyadic Motion Capture (IEMOCAP), results are compared to those of some other CNN structures to validate the advancement of the presented framework. The experimental results turn out that GCNN outperforms others CNN models. Besides, experiments are also done to explore how many key frames should be output from GCNN to involve salient emotional information, results illuminate that limited length representation is properer while too long representation is likely containing redundant information decreasing the performance of the model.
更多查看译文
关键词
Speech emotion recognition,Convolution neural network,Representation learning,Global k-max pooling
AI 理解论文
溯源树
样例
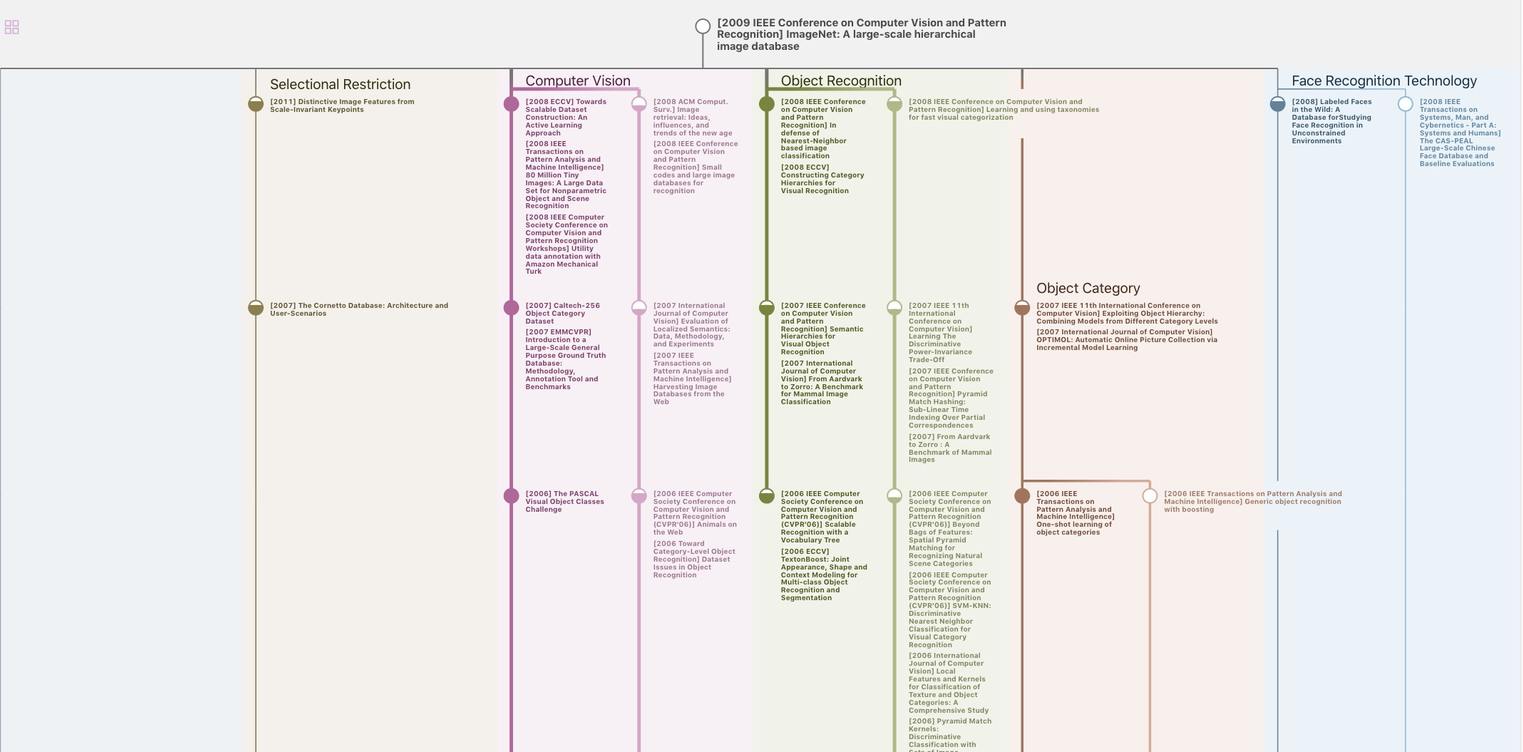
生成溯源树,研究论文发展脉络
Chat Paper
正在生成论文摘要