Empirical Mode Decomposition in Defence Data Analysis
ieee international conference on complex systems(2018)
摘要
Repetitive, but not strictly periodic, trends in the temporal data can present a challenge to the analysis of short-term patterns. Military examples of such time-series include violence data or vessel detections. Empirical mode decomposition (EMD) has been used across a variety of different fields such as biology and plasma physics to deal with non-stationarities in the data. This methodology enables separation of different modes intrinsic to the data and it does not require a priori assumptions about time dependence of various data sub-components, such as periodicity of variations. We show the application of this methodology to two distinct types of data. The Afghanistan violence data provided an example of a sparse, limited dataset. With EMD we were able to identify a multi-year cycle, without the skewed trend in the vicinity of turning points. In contrast, ship detection data for the Canadian West coast provide an example of a large data set. Unfortunately, the analysis of the summary detection data led to the presence of noise limiting our ability to identify specific patterns in the data. The analysis could be improved by geographically dividing the data into a number of small areas and conducting separate analysis for each area. Despite this, the EMD demonstrated its usefulness and applicability, enhancing the analysis of these two datasets compared to more conventional approaches.
更多查看译文
关键词
Empirical mode decomposition, Non-stationarities, Time series analysis
AI 理解论文
溯源树
样例
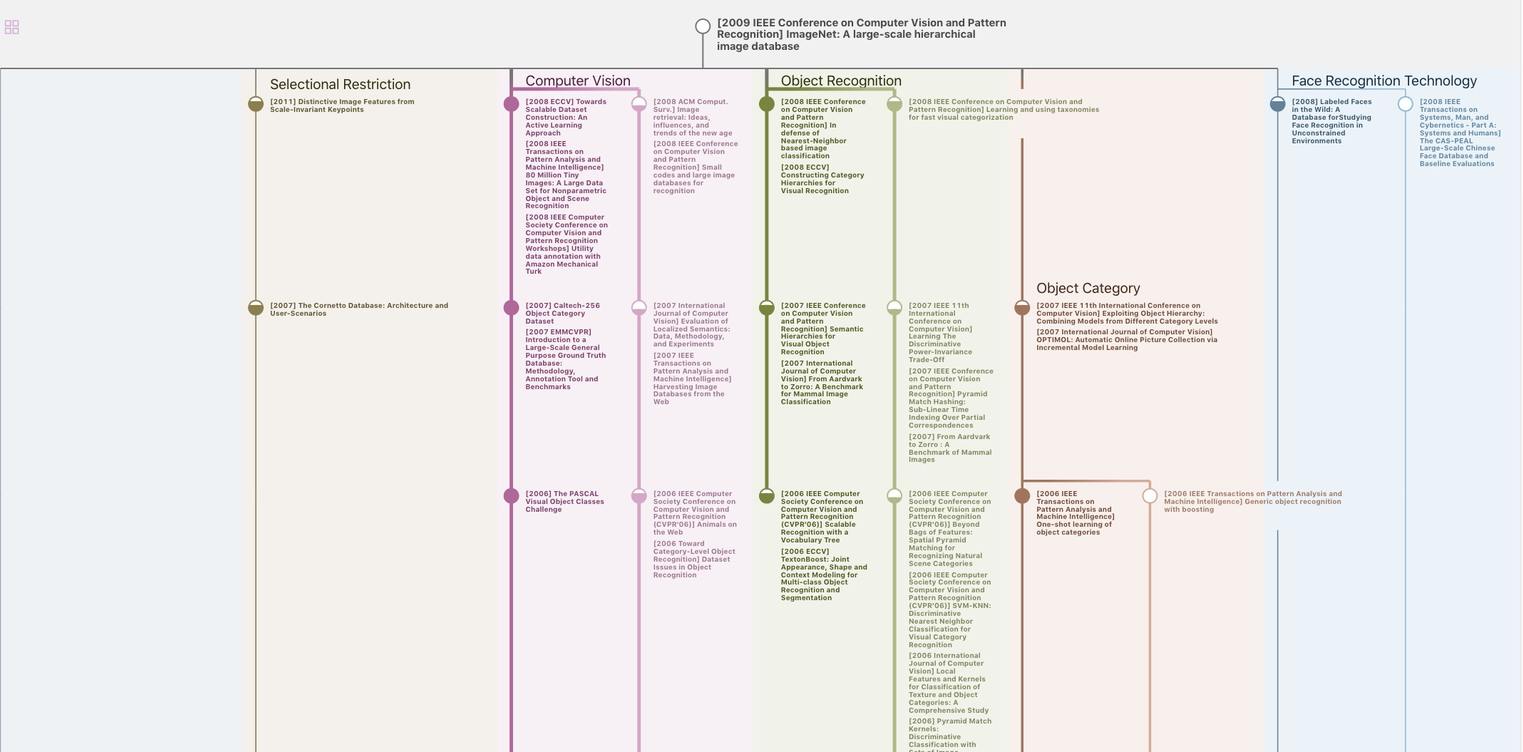
生成溯源树,研究论文发展脉络
Chat Paper
正在生成论文摘要