Learning Disease vs Participant Signatures: a permutation test approach to detect identity confounding in machine learning diagnostic applications.
arXiv: Applications(2018)
摘要
Recently, Saeb et al (2017) showed that, in diagnostic machine learning applications, having data of each randomly assigned to both training and test sets (record-wise data split) can lead to massive underestimation of the cross-validation prediction error, due to the presence of subject identity confounding caused by the classifieru0027s ability to identify subjects, instead of recognizing disease. To solve this problem, the authors recommended the random assignment of the data of each to either the training or the test set (subject-wise data split). The adoption of subject-wise split has been criticized in Little et al (2017), on the basis that it can violate assumptions required by cross-validation to consistently estimate generalization error. In particular, adopting subject-wise splitting in heterogeneous data-sets might lead to model under-fitting and larger classification errors. Hence, Little et al argue that perhaps the overestimation of prediction errors with subject-wise cross-validation, rather than underestimation with record-wise cross-validation, is the reason for the discrepancies between prediction error estimates generated by the two splitting strategies. In order to shed light on this controversy, we focus on simpler classification performance metrics and develop permutation tests that can detect identity confounding. By focusing on permutation tests, we are able to evaluate the merits of record-wise and subject-wise data splits under more general statistical dependencies and distributional structures of the data, including situations where cross-validation breaks down. We illustrate the application of our tests using synthetic and real data from a Parkinsonu0027s disease study.
更多查看译文
AI 理解论文
溯源树
样例
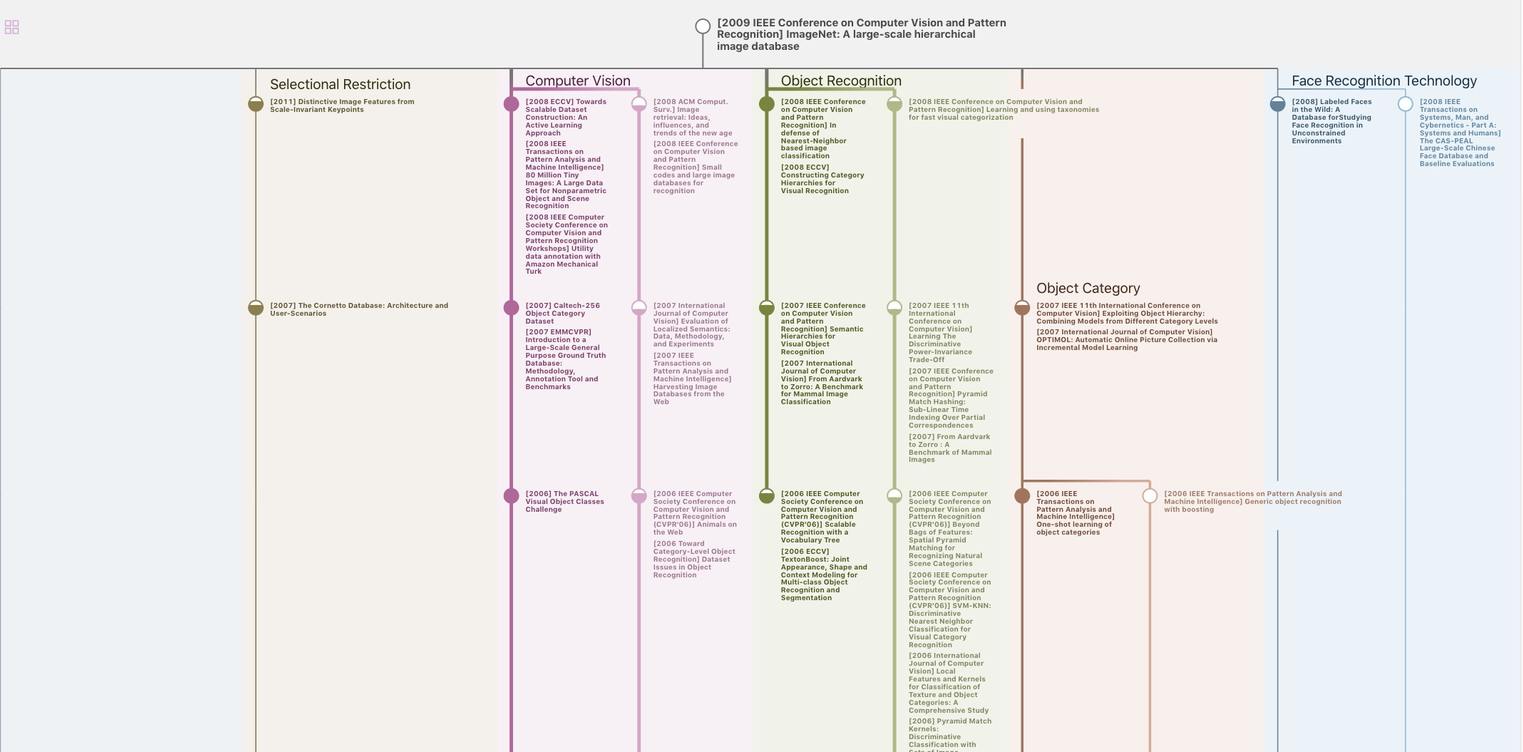
生成溯源树,研究论文发展脉络
Chat Paper
正在生成论文摘要