Quasi Markov Chain Monte Carlo Methods
arXiv: Statistics Theory(2018)
摘要
Quasi-Monte Carlo (QMC) methods for estimating integrals are attractive since the resulting estimators typically converge at a faster rate than pseudo-random Monte Carlo. However, they can be difficult to set up on arbitrary posterior densities within the Bayesian framework, in particular for inverse problems. We introduce a general parallel Markov chain Monte Carlo (MCMC) framework, for which we prove a law of large numbers and a central limit theorem. In that context, non-reversible transitions are investigated. We then extend this approach to the use of adaptive kernels and state conditions, under which ergodicity holds. As a further extension, an importance sampling estimator is derived, for which asymptotic unbiasedness is proven. We consider the use of completely uniformly distributed (CUD) numbers within the above mentioned algorithms, which leads to a general parallel quasi-MCMC (QMCMC) methodology. We prove consistency of the resulting estimators and demonstrate numerically that this approach scales close to n^-2 as we increase parallelisation, instead of the usual n^-1 that is typical of standard MCMC algorithms. In practical statistical models we observe multiple orders of magnitude improvement compared with pseudo-random methods.
更多查看译文
AI 理解论文
溯源树
样例
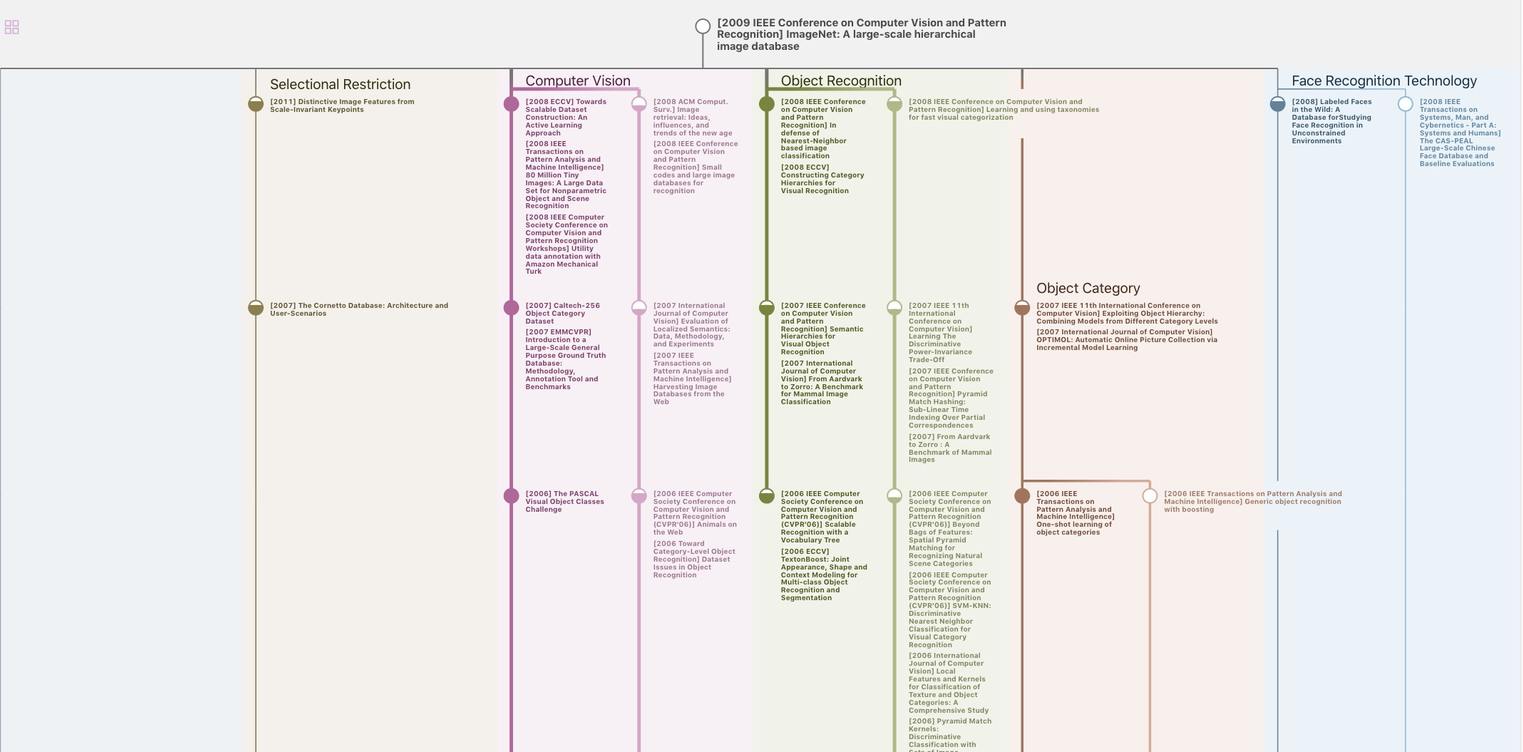
生成溯源树,研究论文发展脉络
Chat Paper
正在生成论文摘要