CDAS: A Cognitive Decision-Making Architecture for Dynamic Airspace Sectorization for Efficient Operations
IEEE Transactions on Intelligent Transportation Systems(2019)
摘要
In this paper, a cognitive decision-making architecture for dynamic airspace sectorization (CDAS) to handle increasing traffic flow and provide an efficient decision making process for operations is presented. The main objective of CDAS is to determine optimal 3-D sector shapes such that the conflicting workloads of air traffic controllers are balanced along with marginal changes to sector shapes over a time horizon. CDAS broadly comprises two major components, namely, a cognitive engine and a metacognitive decision maker. The cognitive engine includes an airspace sectorization model and a multi-objective optimization solver. The problem of 3-D dynamic airspace resectorization is cast as a multi-objective optimization problem with safety constraints and is solved using the non-dominated sorting genetic algorithm II. The metacognitive decision maker utilizes the Pareto-optimal solutions obtained from the cognitive engine along with the air traffic control requirements and predicted traffic pattern to identify the best solution that can be implemented along with a rule to decide on
when-to-do
a resectorization when needed. A detailed performance evaluation of CDAS is presented using the actual flight data over the Singapore flight information region. The results clearly indicate that CDAS provides an efficient dynamic sectorization solution over the existing solution of split-and-merge of a specific sector to handle heavy traffic.
更多查看译文
关键词
Monitoring,Shape,Optimization,Engines,Atmospheric modeling,Complexity theory,Decision making
AI 理解论文
溯源树
样例
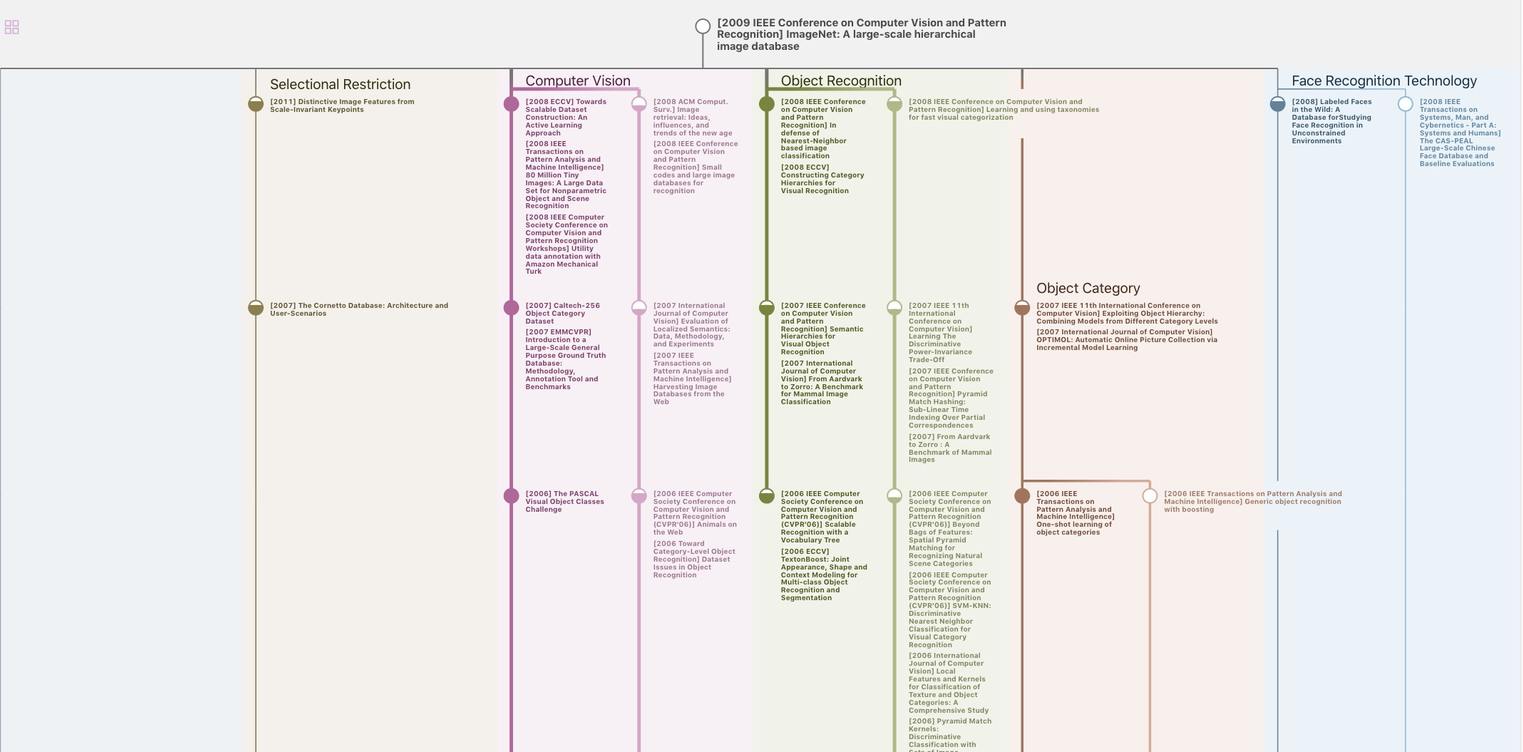
生成溯源树,研究论文发展脉络
Chat Paper
正在生成论文摘要