User identity linkage across social networks via linked heterogeneous network embedding
World Wide Web(2018)
摘要
User identity linkage has important implications in many cross-network applications, such as user profile modeling, recommendation and link prediction across social networks. To discover accurate cross-network user correspondences, it is a critical prerequisite to find effective user representations. While structural and content information describe users from different perspectives, there is a correlation between the two aspects of information. For example, a user who follows a celebrity tends to post content about the celebrity as well. Therefore, the projections of structural and content information of a user should be as close to each other as possible, which inspires us to fuse the two aspects of information in a unified space. However, owing to the information heterogeneity, most existing methods extract features from content and structural information respectively, instead of describing them in a unified way. In this paper, we propose a Linked Heterogeneous Network Embedding model (LHNE) to learn the comprehensive representations of users by collectively leveraging structural and content information in a unified framework. We first model the topics of user interests from content information to filter out noise. Next, cross-network structural and content information are embedded into a unified space by jointly capturing the friend-based and interest-based user co-occurrence in intra-network and inter-network, respectively. Meanwhile, LHNE learns user transfer and topic transfer for enhancing information exchange across networks. Empirical results show LHNE outperforms the state-of-the-art methods on both real social network and synthetic datasets and can work well even with little or no structural information.
更多查看译文
关键词
User identity linkage, Network embedding, Transfer learning, Heterogeneous social network
AI 理解论文
溯源树
样例
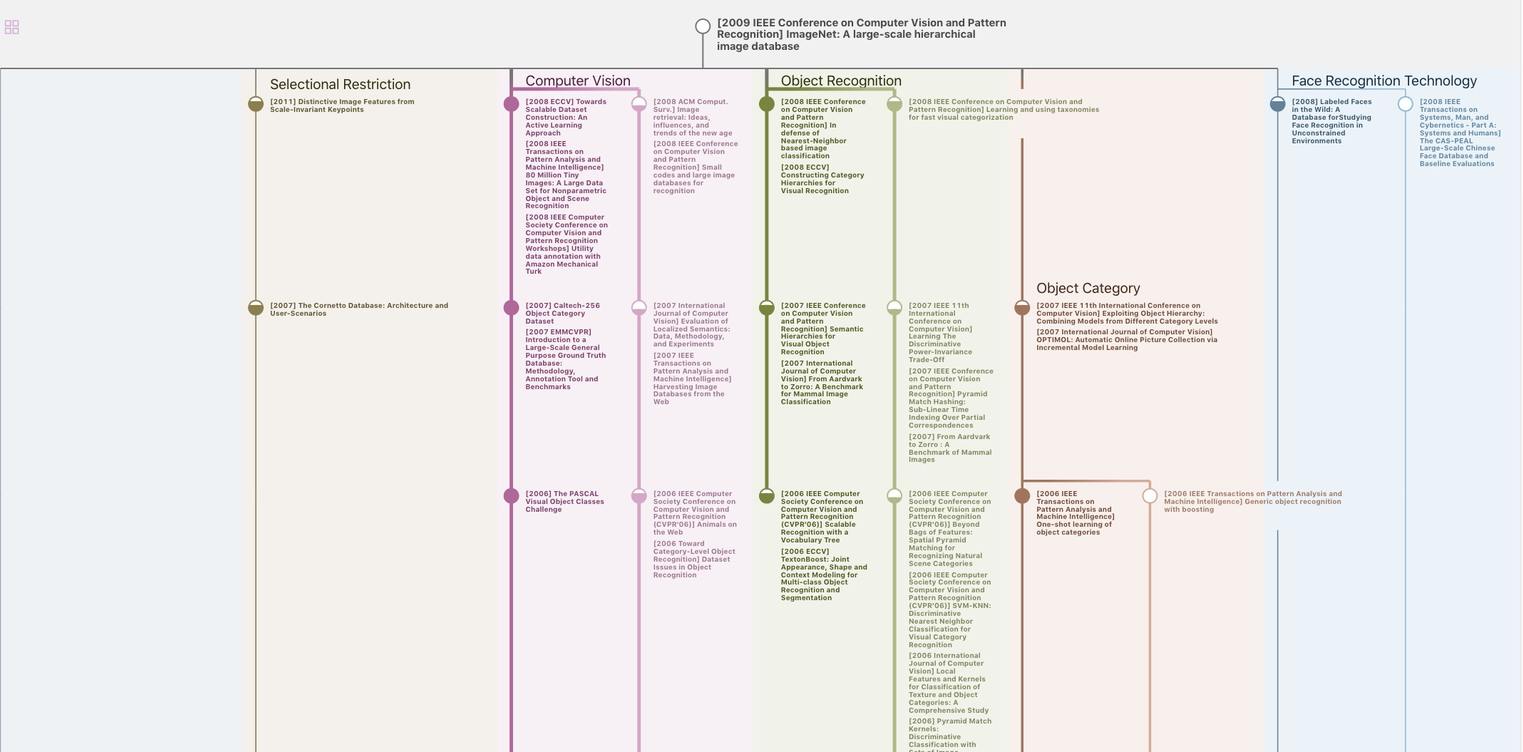
生成溯源树,研究论文发展脉络
Chat Paper
正在生成论文摘要