On the Taylor Expansion of Value Functions
Periodicals(2020)
摘要
AbstractTaylored MDPsBrownian approximations often capture structural relationships that are inaccessible in the original, “too” detailed dynamic programming problem. However, they have not been widely used as a way to reduce computational complexity. Inspired by perturbation techniques that were recently developed for the approximation of stationary queues by “Brownian queues,” the authors of “On the Taylor Expansion of Value Functions” introduce a framework for approximate dynamic programming and apply it to discrete-time-and-state chains with countable action sets. The framework stipulates applying a second-order Taylor expansion to the value function, replacing the Bellman equation with one in continuous space and time in which the transition matrix is reduced to its first and second moments. Bounds on the optimality gap are developed, and they can be viewed as a conceptual underpinning for the good performance of controls derived from Brownian approximations. The framework leads to an “aggregation” approach with performance guarantees.We introduce a framework for approximate dynamic programming that we apply to discrete-time chains on ℤ+d with countable action sets. The framework is grounded in the approximation of the (controlled) chain’s generator by that of another Markov process. In simple terms, our approach stipulates applying a second-order Taylor expansion to the value function, replacing the Bellman equation with one in continuous space and time in which the transition matrix is reduced to its first and second moments. In some cases, the resulting equation can be interpreted as a Hamilton–Jacobi–Bellman equation for a Brownian control problem. When tractable, the “Taylored” equation serves as a useful modeling tool. More generally, it is a starting point for approximation algorithms. We develop bounds on the optimality gap—the suboptimality introduced by using the control produced by the Taylored equation. These bounds can be viewed as a conceptual underpinning, analytical rather than relying on weak convergence arguments, for the good performance of controls derived from Brownian approximations. We prove that under suitable conditions and for suitably “large” initial states, (1) the optimality gap is smaller than a 1 – α fraction of the optimal value, with which α ∈ (0, 1) is the discount factor, and (2) the gap can be further expressed as the infinite-horizon discounted value with a “lower-order” per-period reward. Computationally, our framework leads to an “aggregation” approach with performance guarantees. Although the guarantees are grounded in partial differential equation theory, the practical use of this approach requires no knowledge of that theory.
更多查看译文
关键词
Taylor expansion,Markov decision process,Hamilton-Jacobi-Bellman equation,approximate dynamic programming,approximate policy iteration
AI 理解论文
溯源树
样例
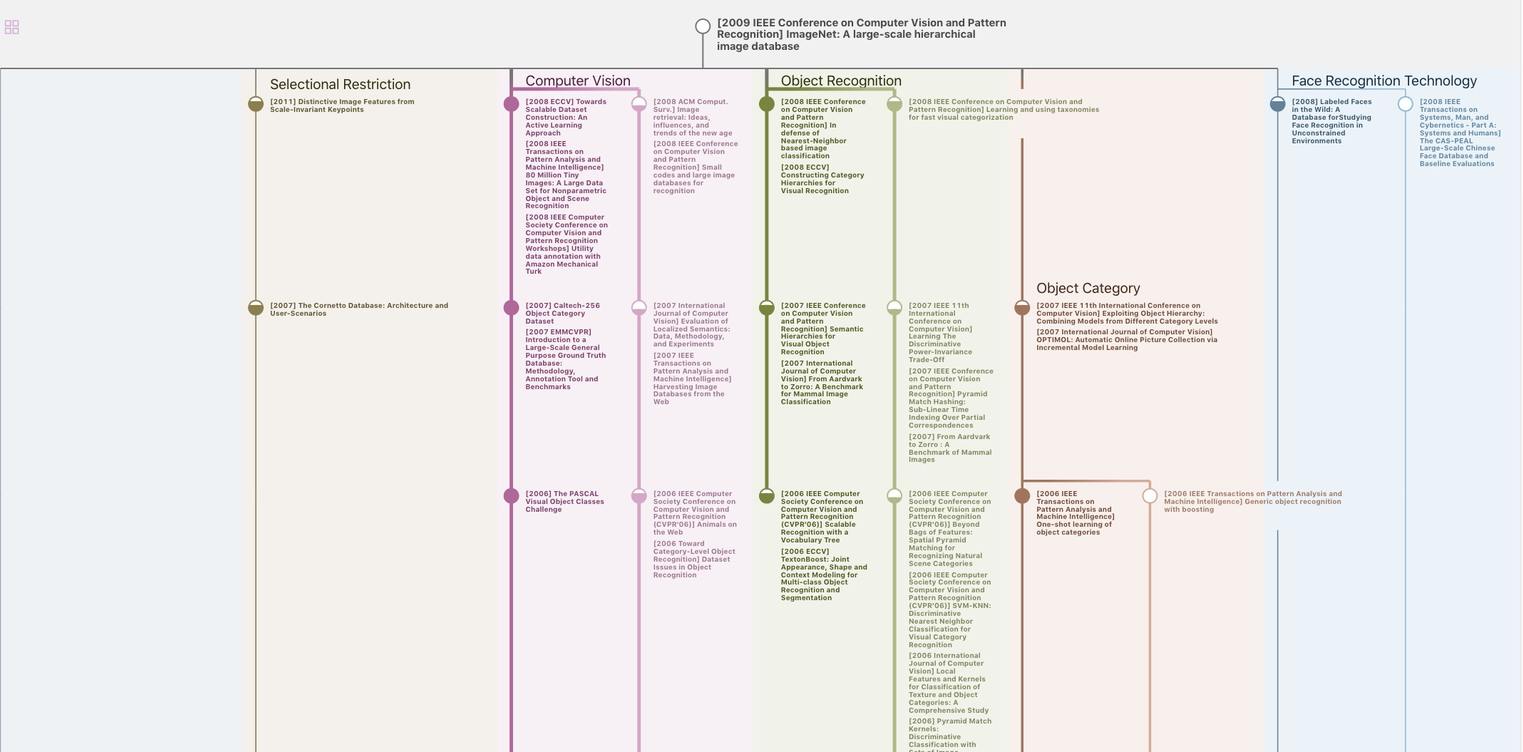
生成溯源树,研究论文发展脉络
Chat Paper
正在生成论文摘要