BIND: An indexing strategy for big data processing
TENCON IEEE Region 10 Conference Proceedings(2017)
摘要
With the huge amount of data continuously accumulated and shared by individuals and organizations, it has become necessary to meet the emerging processing and information retrieval requirements associated with these large volumes of data. This could be achieved by indexing the data sets and reducing heavy computational overhead accustomed to most current indexing strategies during processing of very large amounts of data sets. This study proposes a novel Indexing strategy called Big Data INDexing Strategy (BIND), using a concept of high performance parallel computing. BIND supports parallel distribution of data and performs processing in a MapReduce fashion. To develop the BIND strategy, Ian Foster's task-scheduling concept for parallel processing is applied. The proposed indexing strategy was first tested on a 2-node cluster environment where varying sizes of datasets were used to note if the performance improves or declines as the size of the data increases. Subsequently, it was tested on a 3-node cluster to note the performance when the number of computation resources are increased. The results demonstrates that BIND minimizes the processing and query time as compared to the current strategy. The findings have significant implication in efficiently managing Big Data and facilitating data processing and information retrieval for users and organizations that manage Big Data.
更多查看译文
关键词
Big Data Analytics,Indexing Strategy,MapReduce,Information Retrieval
AI 理解论文
溯源树
样例
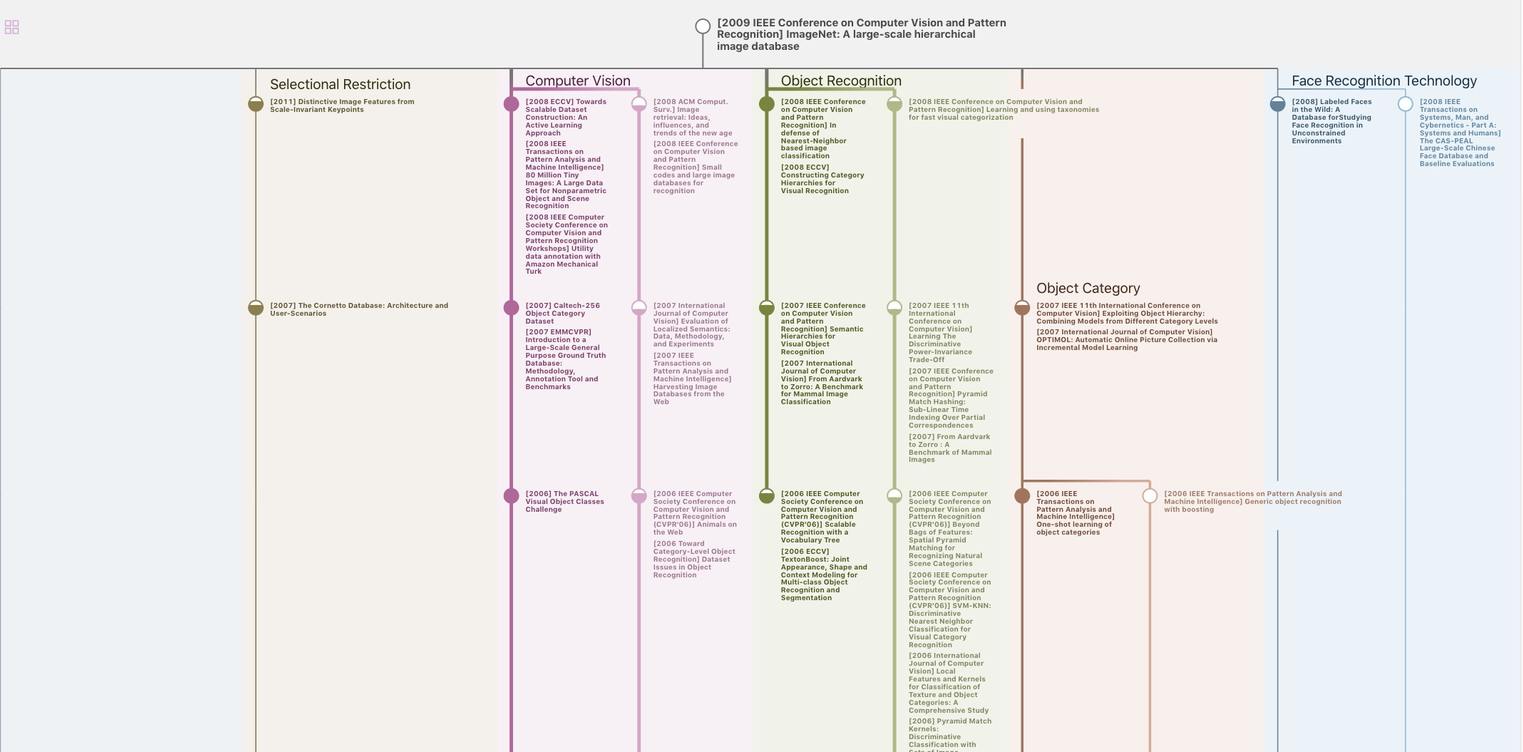
生成溯源树,研究论文发展脉络
Chat Paper
正在生成论文摘要