A Bayesian Covariance Graphical and Latent Position Model for Multivariate Financial Time Series
arXiv: Applications(2017)
摘要
Current understanding holds that financial contagion is driven mainly by the system-wide interconnectedness of institutions. A distinction has been made between systematic and idiosyncratic channels of contagion, with shocks transmitted through the latter expected to be substantially more likely to lead to systemic crisis than through the former. Idiosyncratic connectivity is thought to be driven not simply by obviously shared characteristics among institutions, but more by latent characteristics that lead to the holding of related securities. We develop a graphical model for multivariate financial time series with interest in uncovering the latent positions of nodes in a network intended to capture idiosyncratic relationships. We propose a hierarchical model consisting of a VAR, a covariance graphical model (CGM) and a latent position model (LPM). The VAR enables us to extract useful information on the idiosyncratic components, which are used by the CGM to model the network and the LPM uncovers the spatial position of the nodes. We also develop a Markov chain Monte Carlo algorithm that iterates between sampling parameters of the CGM and the LPM, using samples from the latter to update prior information for covariance graph selection. We show empirically that modeling the idiosyncratic channel of contagion using our approach can relate latent institutional features to systemic vulnerabilities prior to a crisis.
更多查看译文
关键词
bayesian covariance graphical,latent position model,financial
AI 理解论文
溯源树
样例
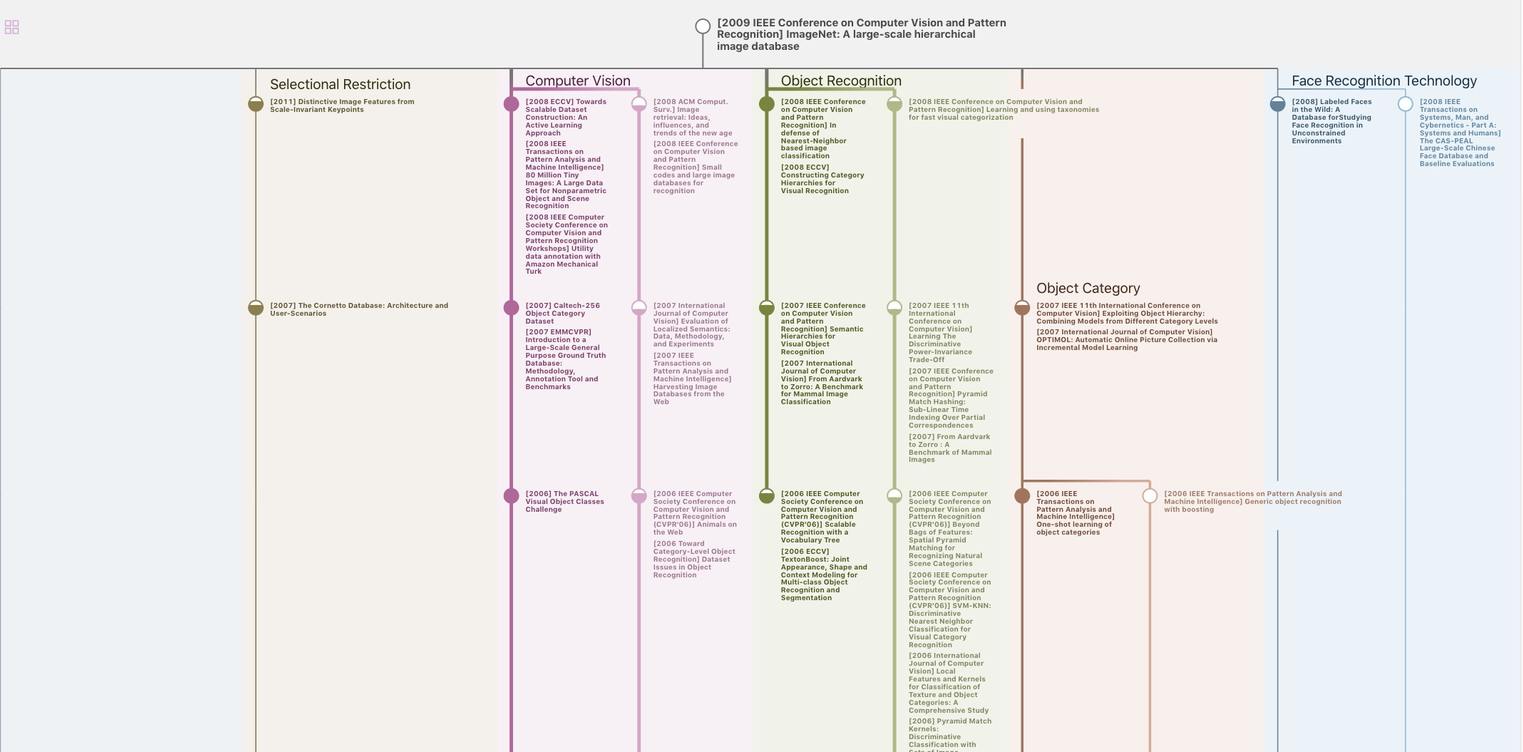
生成溯源树,研究论文发展脉络
Chat Paper
正在生成论文摘要