Work-In-Progress: Cloud-Based Machine Learning For Iot Devices With Better Privacy
embedded software(2017)
摘要
Recently, cloud-based machine learning (ML) services are getting popular. A client sends input data to a cloud, which performs ML algorithms based on neural networks (NN) using its powerful server, and returns the result back to the client. In this scenario, the server can easily collect the users' sensitive data, raising the privacy issues and making users reluctant to use the services. This paper proposes a new approach to ML services that can reduce the privacy concern, thus making the services safer. The basic idea is that instead of sending the raw input data, the client sends the partially-processed feature data obtained from the early stage of the NN, and the server continues to execute the rest of the NN. Since it is not simple to reverse-engineer the original data from the feature data, especially when the feature data is obtained from the later stage of NN, the privacy issue can be reduced. On the other hand, partial processing at the client can affect the performance, as more computations are performed at the client. We evaluated the performance impact, considering the network speed as well. We are also trying to devise a privacy metric to evaluate the partially-processed feature data.
更多查看译文
关键词
Embedded System,Privacy,Neural Network,Cloud Computing
AI 理解论文
溯源树
样例
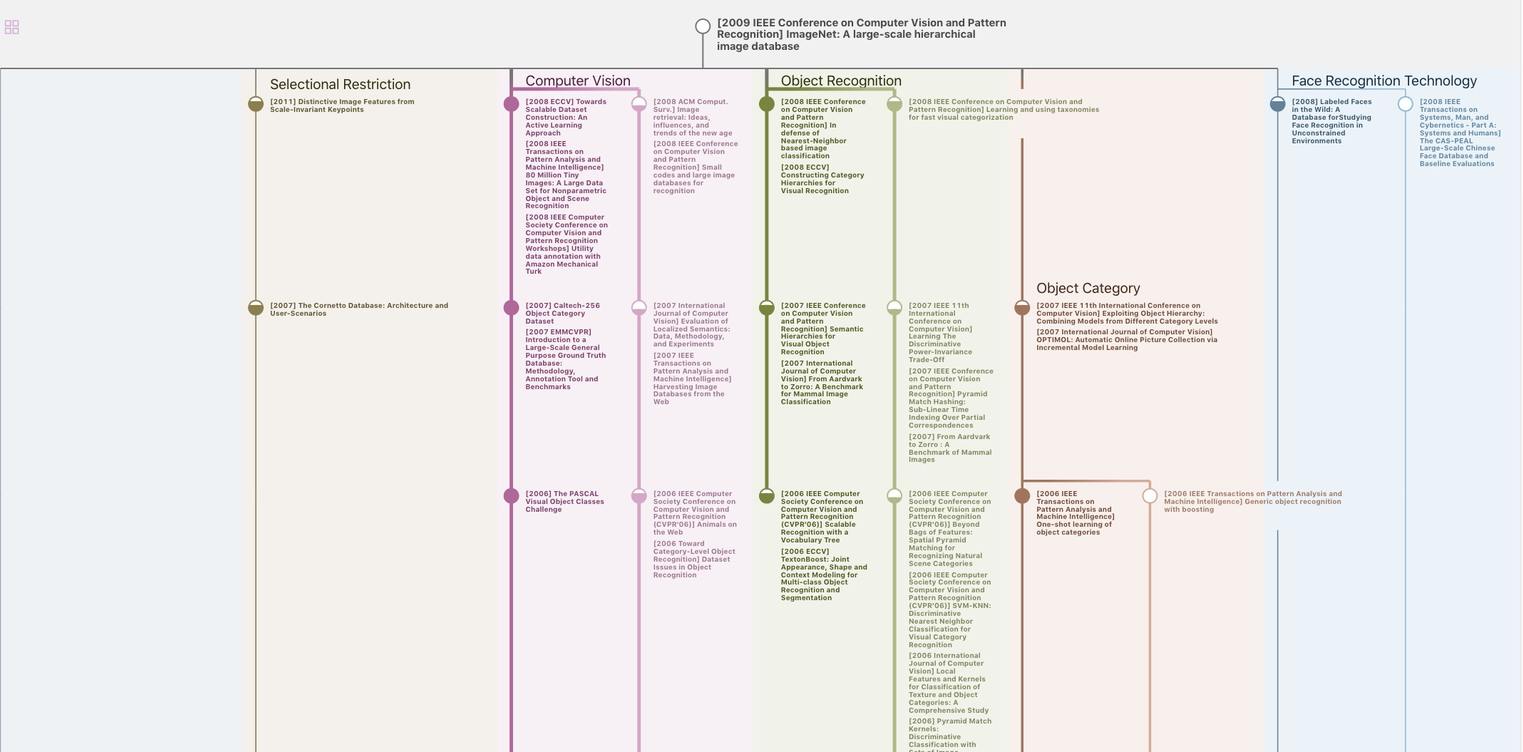
生成溯源树,研究论文发展脉络
Chat Paper
正在生成论文摘要