Variability of human-annotations of 12-lead ECG features collected using a web system: Students vs. practitioners
Journal of Electrocardiology(2018)
摘要
Introduction: The electrocardiogram (ECG) is often interpreted incorrectly with up to 33% of interpretations containing a significant error. The difficulty in ECG interpretation is two-fold; 1) it demands an extensive knowledge of cardiac physiology, and 2) the ECG inflates cognitive workload due to the complex nature of its presentation. To make a diagnosis, the reader is required to measure ECG features in order to contrast these annotations with diagnostic criteria. Whilst signal processing algorithms can provide automated measurements, they are often imprecise. Based on these observations, a web-based system was developed to allow the interpreter to measure and input their own ECG annotations. These annotations are then processed by a rule-based algorithm, which presents a set of suggested diagnoses.However, imprecise and inconsistent human annotations would affect both the readeru0027s diagnostic decision making and also the accuracy of the diagnoses suggested by the algorithm (junk in = junk out). Our study measures the variability of manual annotations collected using our web system. Clinical physiology students (n = 10) and medical practitioners (n = 11) participated in our study.Results: Annotations for the same ECG are as follows (all participants = A, students = S, medical practitioners = P):Heart Rate (A[mean = 89.39 bpm, SD = 9.95], S[mean = 88.7 bpm, SD = 4.27], P[mean = 91.4 bpm, SD = 14.68], p = 0.73), P-wave duration (A[mean = 0.08 s, SD = 0.02], S[mean = 0.09 s, SD = 0.03], P[mean = 0.08 s, SD = 0.01], p = 0.39), P-wave amplitude (A[mean = 0.18 mv, SD = 0.04], S[mean = 0.19 mv, SD = 0.05], P[mean = 0.18 mv, SD = 0.3], p = 0.38), P-R interval (A[mean = 0.16 s, SD = 0.04], S[mean = 0.18 s, SD = 0.05], P[mean = 0.16 s, SD = 0.03], p = 0.49), cardiac axis (A[mean = 58.11°, SD = 13.23], S[mean = 60°, SD = 0], P[mean = 51.5°, SD = 18.8], p = 0.46), Q-T interval (A[mean = 0.32 s, SD = 0.14], S[mean = 0.41 s, SD = 0.06], P[mean = 0.24 s, SD = 0.17], p u003c 0.01), R-R interval (A[mean = 0.63 s, SD = 0.21], S[mean = 0.72 s, SD = 0.13], P[mean = 0.53 s, SD = 0.27], p = 0.06) and QTc (A[mean = 0.4 s, SD = 0.15], S[mean = 0.48 s, SD = 0.09], P[mean = 0.33 s, SD = 0.2], p = 0.02).Discussion: Students annotated more features (5/8) with less variance. Students annotate interval measurements with 47% less variation than medical practitioners (Σ interval measurement; students SD = 0.36, practitioners SD = 0.68). Students also had less variation in measuring heart rate, P-wave amplitude and cardiac axis. Two of the annotated features (QT-interval and QTc) from both cohorts were statistically different (p ≤ 0.05).Conclusion: In order to make an accurate diagnosis precise ECG annotations are required. This study determined the variability of manual ECG annotations on a cohort containing both students and practitioners.
更多查看译文
关键词
web system,features,human-annotations
AI 理解论文
溯源树
样例
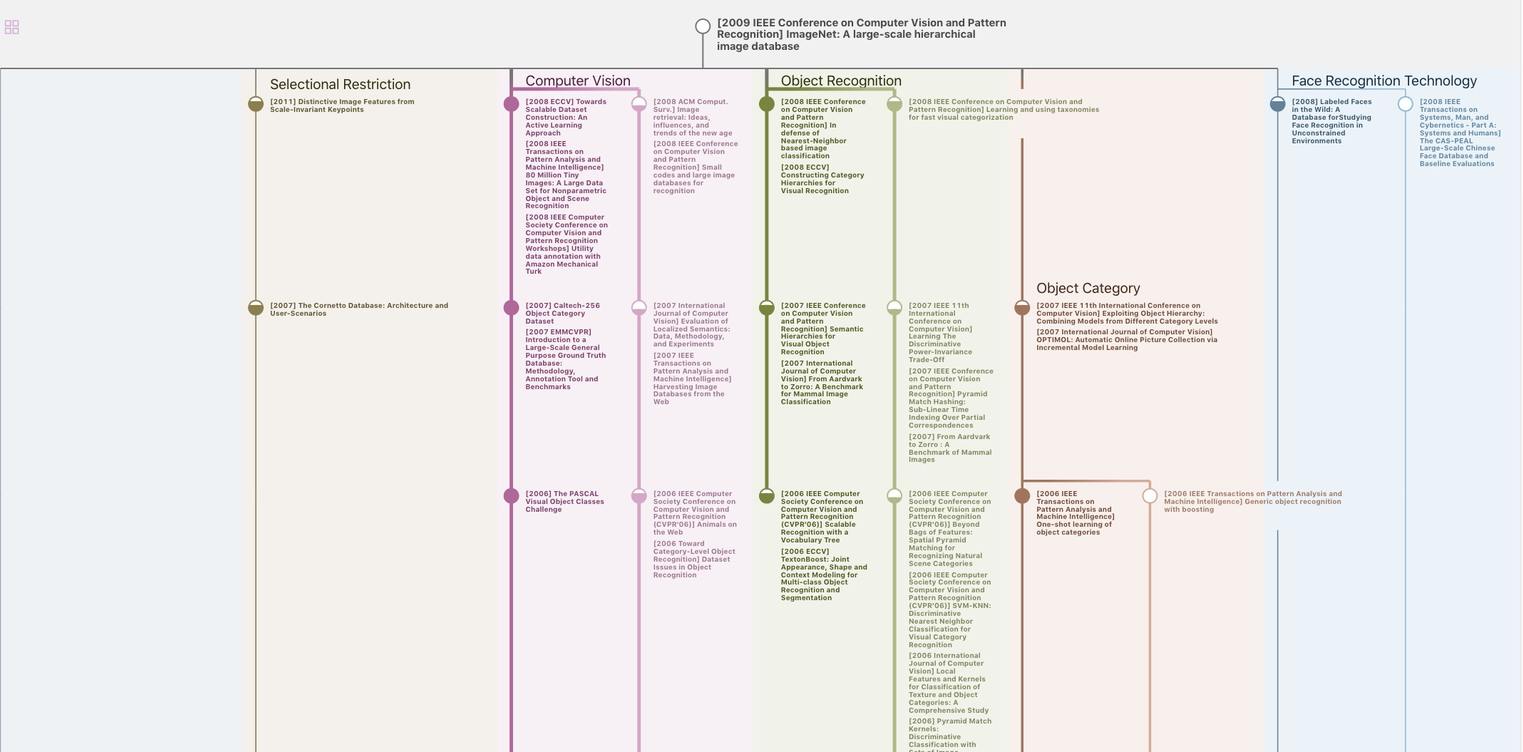
生成溯源树,研究论文发展脉络
Chat Paper
正在生成论文摘要