Learning scene-aware image priors with high-order Markov random fields
Applied Informatics(2017)
摘要
Many methods have been proposed to learn image priors from natural images for the ill-posed image restoration tasks. However, many prior learning algorithms assume that a general prior distribution is suitable for over all kinds of images. Since the contents of the natural images and the corresponding low-level statistical characteristics vary from scene to scene, we argue that learning a universal generative prior for all natural images may be imperfect. Although the universal generative prior can remove artifacts and reserve a natural smoothness in image restoration, it also tends to introduce unreal flatness and clutter textures. To address this issue, in this paper, we present to learn a scene-aware image prior based on the high-order Markov random field (MRF) model (SA-MRF). With this model, we jointly learn a set of shared low-level features and different potentials for specific scene contents. In prediction, a good prior can be adapted to the given degenerated image with the scene content perception. Experimental results on the image denoising and inpainting tasks demonstrate the efficiency of the SA-MRF on both numerical evaluation and visual compression.
更多查看译文
关键词
Image restoration,Markov random fields,Scene-aware image prior learning
AI 理解论文
溯源树
样例
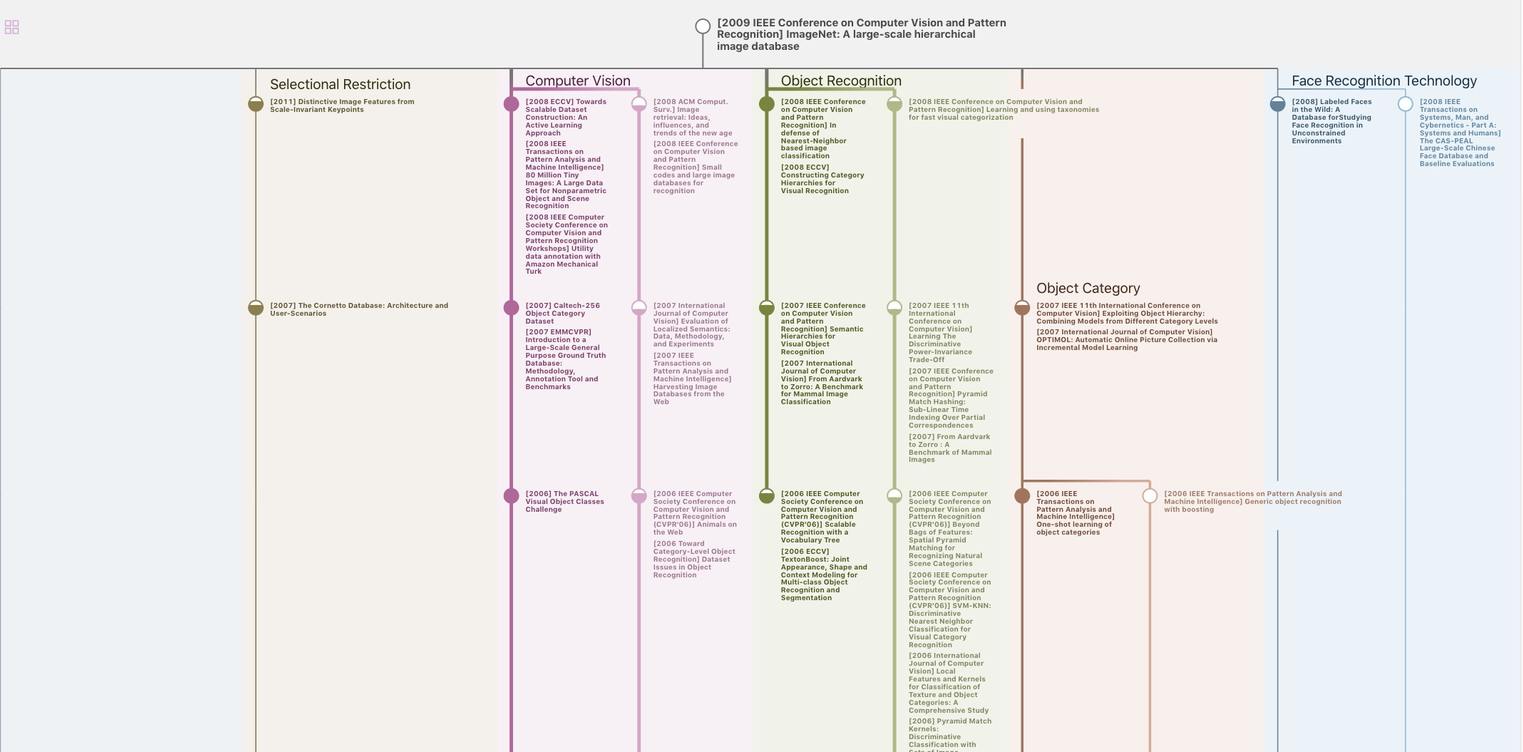
生成溯源树,研究论文发展脉络
Chat Paper
正在生成论文摘要