Parameter-state ensemble data assimilation using ApproximateBayesian Computing for short-term hydrological prediction
Hydrology and Earth System Sciences Discussions(2017)
摘要
Abstract. The main sources of uncertainty in hydrological modelling can be summarized as structural errors, parameter errors, and data errors. Operational modellers are generally more concerned with predictive ability than model errors, and Data Assimilation (DA) methods are commonly employed to merge models with observations to improve predictive ability. This paper presents an example of Approximate Bayesian Computing (ABC), or a simplified Particle Filter (PF), to simultaneously assimilate model states and parameters, calling the method Parameter-State Ensemble DA (P-SEDA). The case study is from June to October, 2014 for a small (1324 km 2 ) watershed just north of Lake Superior in Ontario, Canada using the Canadian semi-distributed hydrologic land-surface scheme MESH. The study examines how well the approach works given various levels of certainty in the data; beginning with certainty in the streamflow and precipitation, followed by uncertainty in the streamflow and certainty in the precipitation, and finally uncertainty in both the streamflow and precipitation. The approach is found to work in this case when streamflow and precipitation is fairly certain, while being more challenging to implement in a forecasting scenario where future streamflow and precipitation is much less certain. The main challenge is determined to be related to parametric uncertainty and ideas for overcoming this challenge are discussed.
更多查看译文
AI 理解论文
溯源树
样例
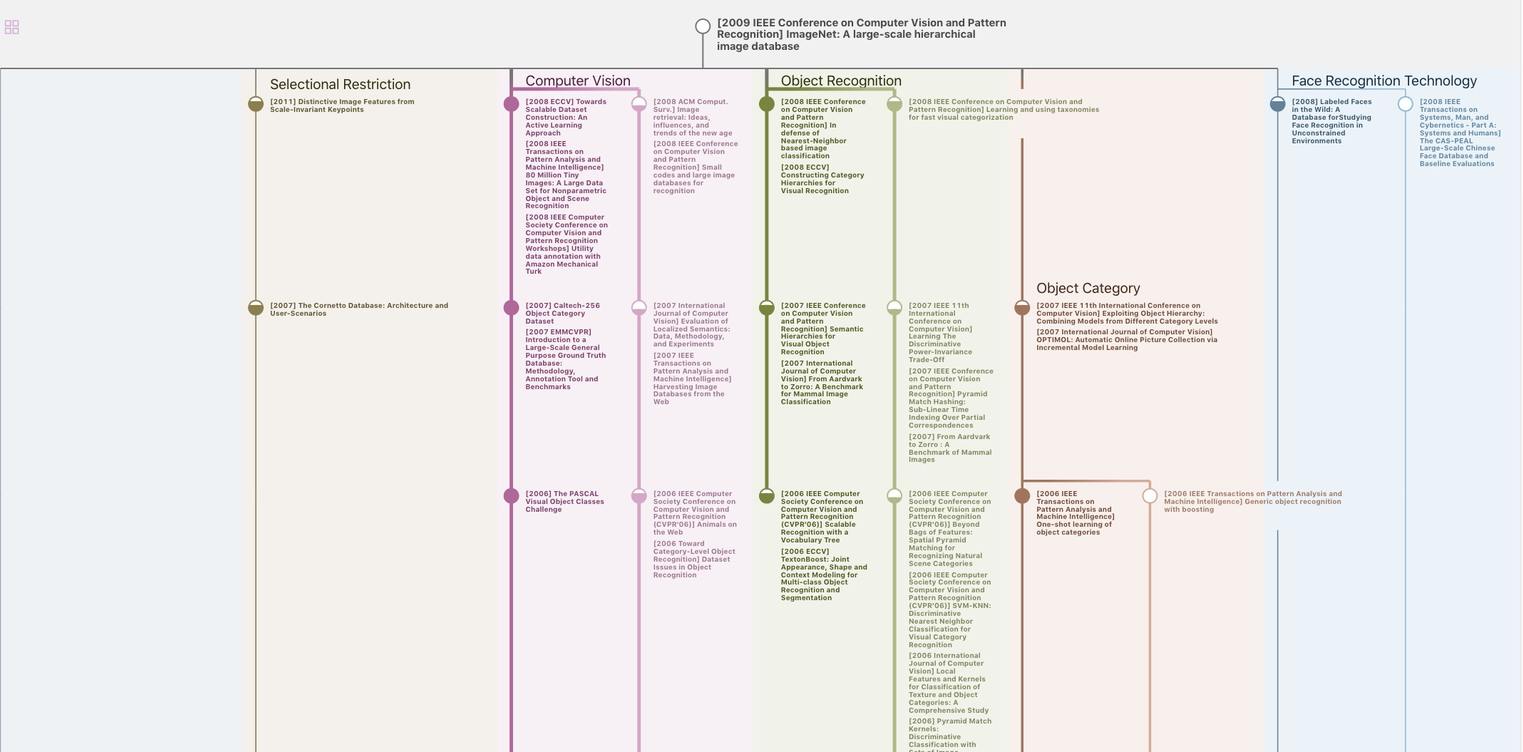
生成溯源树,研究论文发展脉络
Chat Paper
正在生成论文摘要