Machine learning for variability aware statistical device design: The case of perpendicular spin-transfer-torque random access memory
2017 75th Annual Device Research Conference (DRC)(2017)
摘要
Spin-transfer-torque random access memory (STT RAM) is a promising memory technology due to its scalability, endurance and non-volatility. Addressing the process induced variations during realistic device fabrication process is a challenge, while trying to meet performance specifications, more so with the technology scaling leading to smaller device dimensions. In a simplified picture, the performance parameters of an STT RAM cell such as switching current density for a given pulse length or the switching delay for a given applied current density, depend on a variety of material parameters such as magnetic anisotropy and damping constant of the “free layer” (FL), the information storage layer. Besides material parameters, device dimensions, such as the diameter and the thickness of FL also vary about the target values, due to imperfections during thin-film deposition, lithography and ion-beam etching, among other process steps. To consider process variations in such parameters, Monte-Carlo simulations can be used, where each of the parameters can be, e.g., a random number from a Gaussian distribution about its target value. However, a modest number of 5 parameters and 100 values for each of them would require (10
2
)
5
= 10
10
device simulations, and would be computationally infeasible for e.g., micromagnetic simulation. A possible route to circumvent such a prohibitively large computational load would be to use a compact model [1, 2]. However, a method like this relies on the so-called macrospin approximation and assumes spins across FL to be parallel to each other at all times during switching and might not be a true representation. Also, for many emerging devices, a compact model still is not available. Machine learning (ML) has been used in the past to model array of resistive random access memory (RRAM) [3]. In this work, we propose an ML driven simulation methodology to take the effect of process variation into account using micromagnetic simulations with reasonable computational effort. We employ support vector regression (SVR), a method used in supervised learning to anticipate the behavior of a system based on previously obtained “training data”, to predict performance of an STT RAM cell. We use STT RAM as a model system, although the proposed scheme should be usable for other devices too.
更多查看译文
关键词
machine learning,variability aware statistical device design,perpendicular spin-transfer-torque random access memory,process induced variations,technology scaling,device dimensions,performance parameters,STT RAM cell parameters,switching current density,switching delay,material parameters,device simulations,ML driven simulation,micromagnetic simulations,support vector regression,SVR,supervised learning,performance prediction
AI 理解论文
溯源树
样例
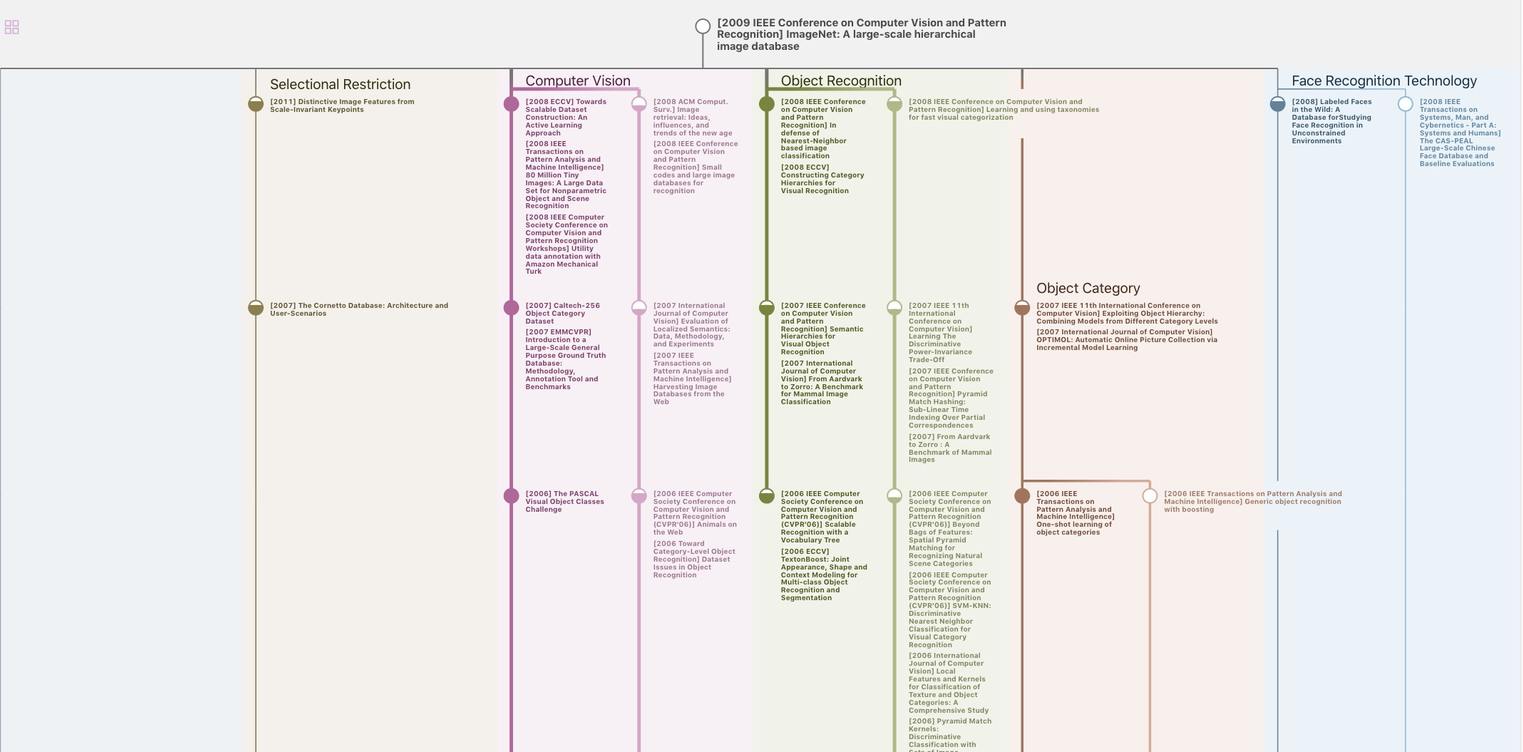
生成溯源树,研究论文发展脉络
Chat Paper
正在生成论文摘要