Maximum Entropy Framework For Inference Of Cell Population Heterogeneity In Signaling Networks
bioRxiv(2019)
摘要
Predictive models of signaling networks are essential tools for understanding cell population heterogeneity and designing rational interventions in disease. However, using network models to predict signaling dynamics heterogeneity is often challenging due to parameter non-identifiability and the variability of network parameters across cell populations. To address these challenges, we present here an inference framework based on the principle of maximum entropy (ME). The developed approach allows us to estimate the joint probability distribution over network parameters that is consistent with experimentally observed cell-to-cell variability in abundances of network species. We apply the inference framework to investigate the heterogeneity in the signaling network activated by the epidermal growth factor (EGF) and leading to phosphorylation of protein kinase B (Akt). Using the inferred parameter distribution, we predict heterogeneity of phosphorylated Akt levels and the distribution of EGF receptor abundance hours after EGF stimulation. We also discuss how the developed framework can be generalized and applied to problems beyond modeling of heterogeneous signaling dynamics.
更多查看译文
AI 理解论文
溯源树
样例
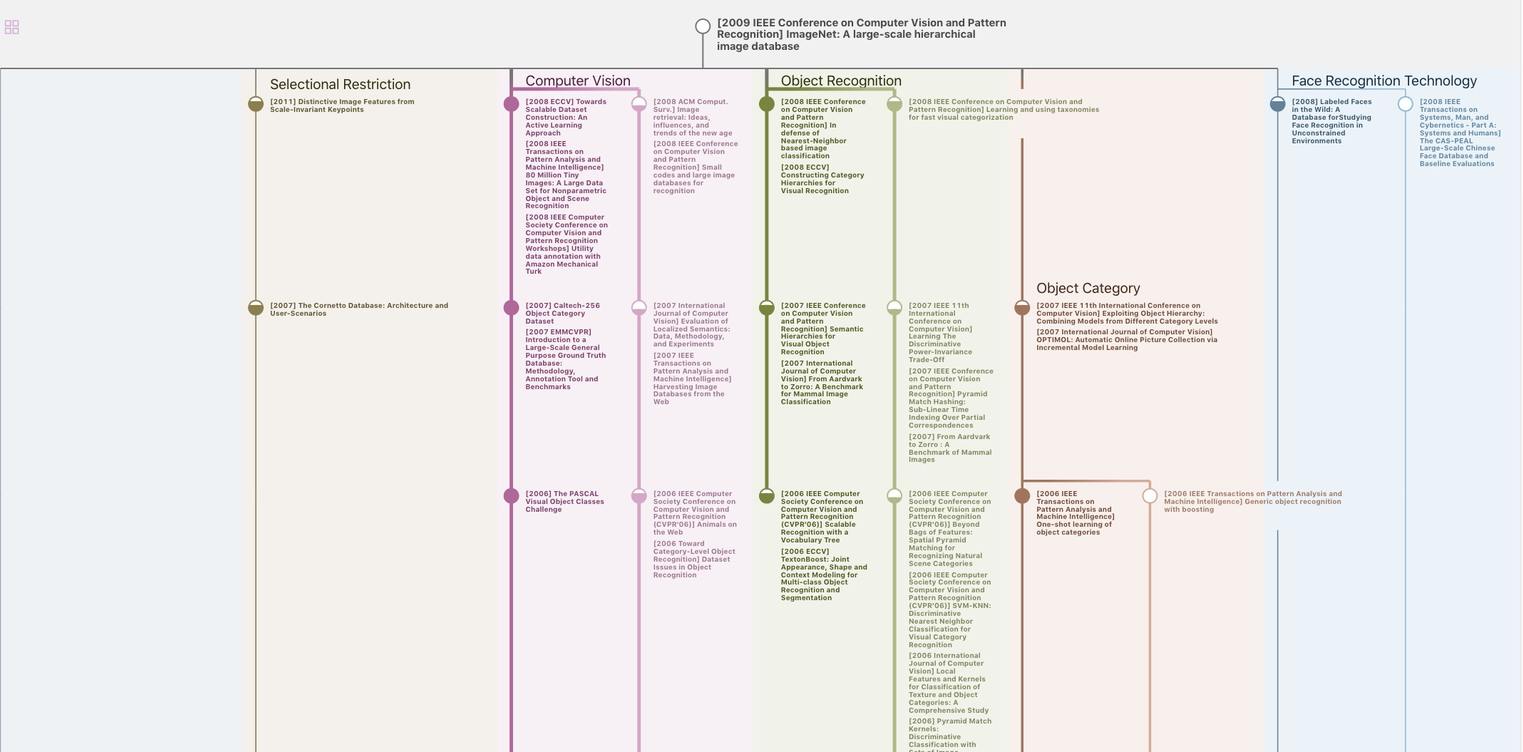
生成溯源树,研究论文发展脉络
Chat Paper
正在生成论文摘要