An unsupervised meta-graph clustering based prototype-specific feature quantification for human re-identification in video surveillance
Engineering Science and Technology, an International Journal(2017)
摘要
Human re-identification is an emerging research area in the field of visual surveillance. It refers to the task of associating the images of the persons captured by one camera (probe set) with the images captured by another camera (gallery set) at different locations in different time instances. The performance of these systems are often challenged by some factors—variation in articulated human pose and clothing, frequent occlusion with various objects, change in light illumination, and the cluttered background are to name a few. Besides, the ambiguity in recognition increases between individuals with similar appearance. In this paper, we present a novel framework for human re-identification that finds the correspondence image pair across non-overlapping camera views in the presence of the above challenging scenarios. The proposed framework handles the visual ambiguity having similar appearance by first segmenting the gallery instances into disjoint prototypes (groups), where each prototype represents the images with high commonality. Then, a weighing scheme is formulated that quantifies the selective and distinct information about the features concerning the level of contribution against each prototype. Finally, the prototype specific weights are utilized in the similarity measure and fused with the existing generic weighing to facilitates improvement in the re-identification. Exhaustive simulation on three benchmark datasets alongside the CMC (Cumulative Matching Characteristics) plot enumerate the efficacy of our proposed framework over the counterparts.
更多查看译文
关键词
Human re-identification,Video surveillance,Prototype discovery,Clustering
AI 理解论文
溯源树
样例
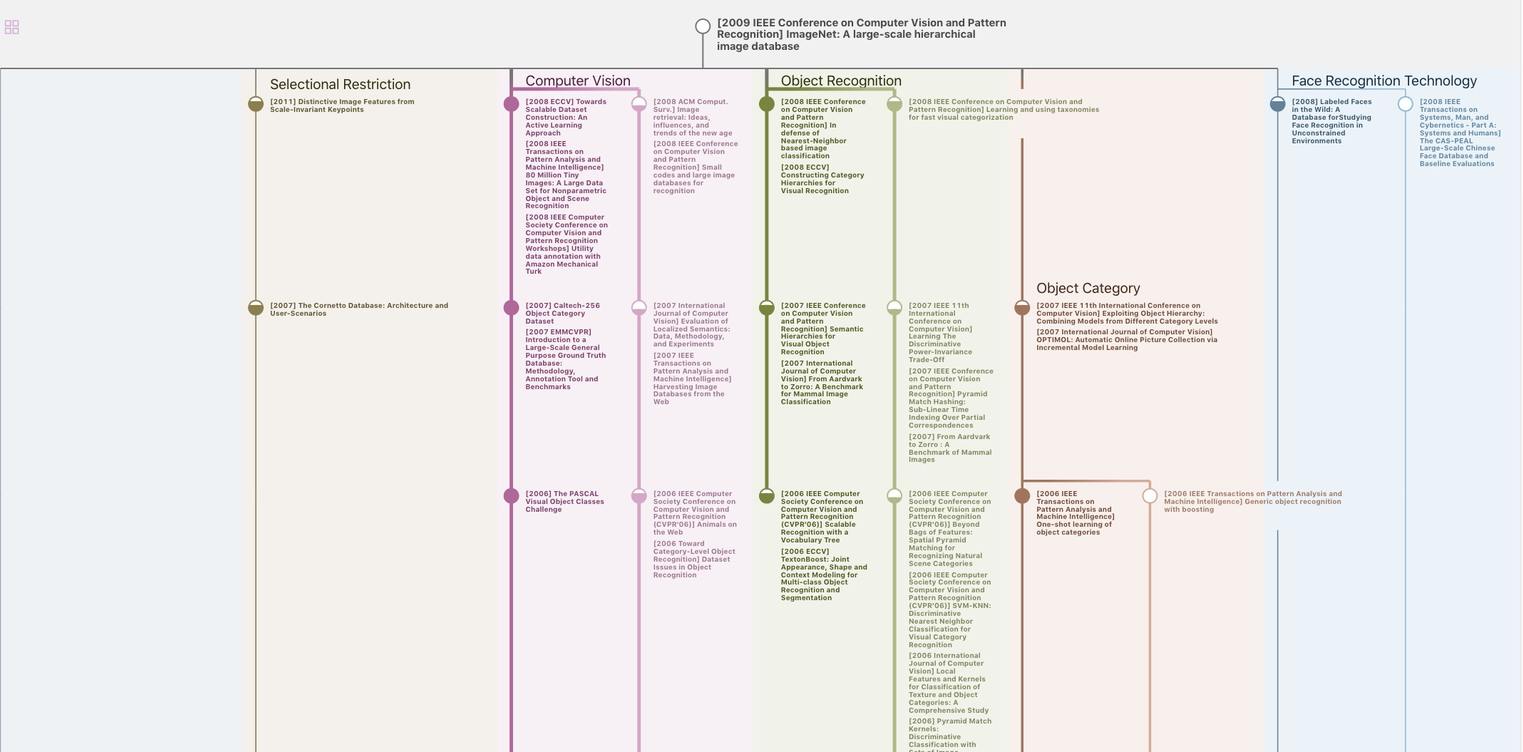
生成溯源树,研究论文发展脉络
Chat Paper
正在生成论文摘要