A kernel matrix dimension reduction method for predicting drug-target interaction
Chemometrics and Intelligent Laboratory Systems(2017)
摘要
The prediction of drug-target interactions plays an important role in the drug discovery process, which serves to identify new drugs or novel targets for existing drugs. However, experimental methods for predicting drug-target interactions are expensive and time-consuming. Therefore, the in silico prediction of drug-target interactions has recently attracted increasing attention. In this study, we proposed a kernel matrix dimension reduction method (KMDR) for predicting drug-target interactions, and in order to facilitate benchmark comparisons, two other representative algorithms, the Regularized Least Squares classifier (RLS) and the semi-supervised link prediction classifier (SLP), were also used to predict drug-target interactions on a same dataset. The results show that the kernel matrix reduction dimension method could improve the performance on drug-target interaction prediction; in particular, KMDR could significantly improve performance on low degree drug target interaction prediction. We further show that, in theory, the formulations of above three algorithms have a unified form, which could be seen as a kernel matrix transformation based on eigenvalue. This finding could provide us a research direction -- to design better algorithms for predicting drug-target interaction by optimize kernel matrix transformation based on eigenvalue.
更多查看译文
关键词
Bipartite graph link prediction,Drug-target interaction network,Kernel matrix dimension reduction method,Kernel matrix transformation
AI 理解论文
溯源树
样例
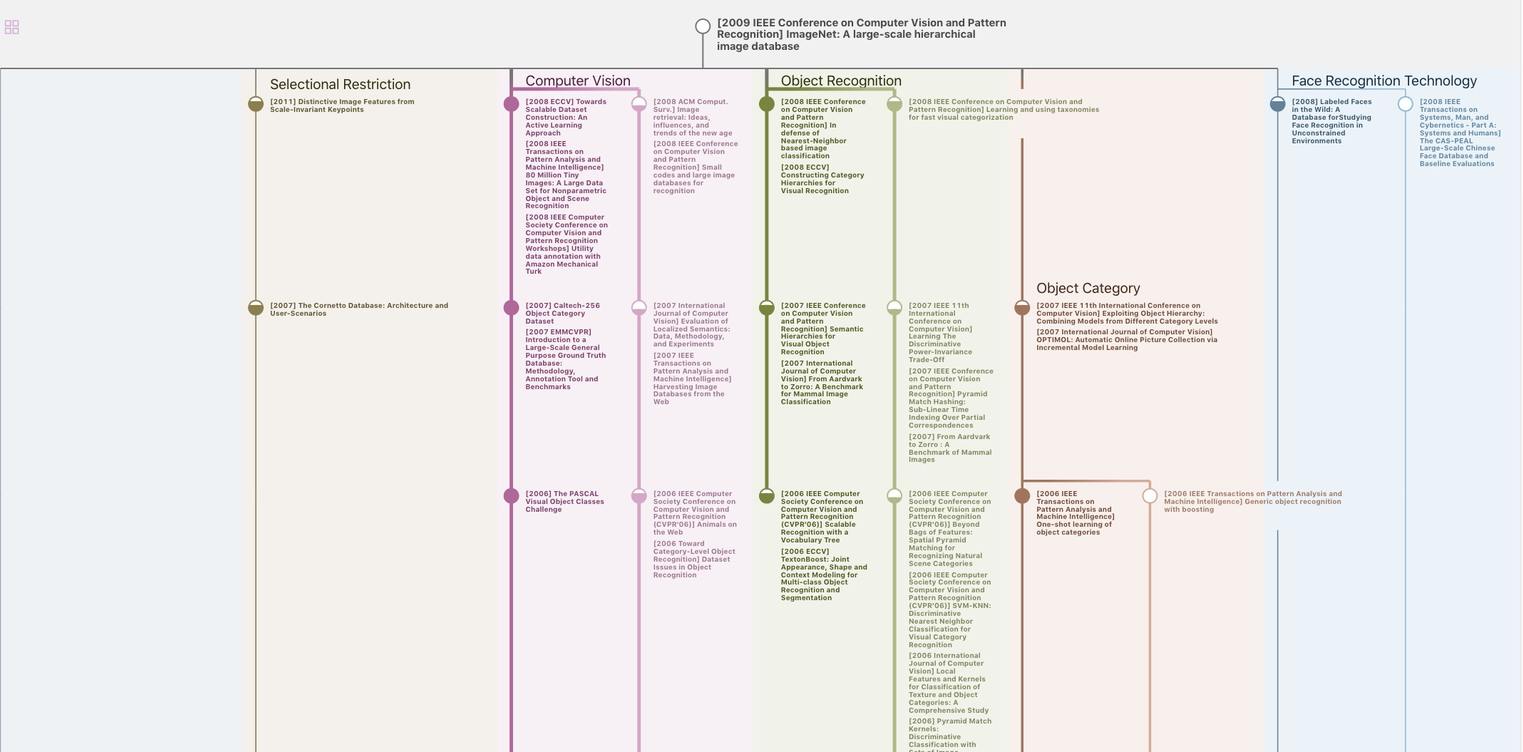
生成溯源树,研究论文发展脉络
Chat Paper
正在生成论文摘要