Beyond spike-timing-dependent plasticity: a computational study of plasticity gradients across basal dendrites.
bioRxiv(2017)
摘要
Synaptic plasticity is thought to be the principal mechanism underlying learning in the brain. The majority of plastic networks in computational neuroscience combine point neurons with spike-timing-dependent plasticity (STDP) as the learning rule. However, a point neuron does not capture the complexity of dendrites. These long branches emanating from the soma allow non-linear local processing of the synaptic inputs. Furthermore, experimental evidence suggests that STDP is not the only learning rule available to neurons. Implementing a biophysically realistic neuron model, we wondered how dendrites allow for multiple synaptic plasticity mechanisms to coexist in a single cell. We show how a local, cooperative form of synaptic strengthening becomes increasingly powerful at larger distances from the soma, typically depending on dendritic spikes, explaining recent experimental results. The cooperative mechanism compensates the ineffectiveness of STDP on weak inputs and in distal parts of the dendrites, enhances the flexibility of a neuron by gating STDP, and allows for novel and robust associative learning schemes. Our work predicts that many plasticity rules increase the computational power of a neuron, and their implementation in future network models could be crucial to gain a deeper understanding of learning in the brain.
更多查看译文
AI 理解论文
溯源树
样例
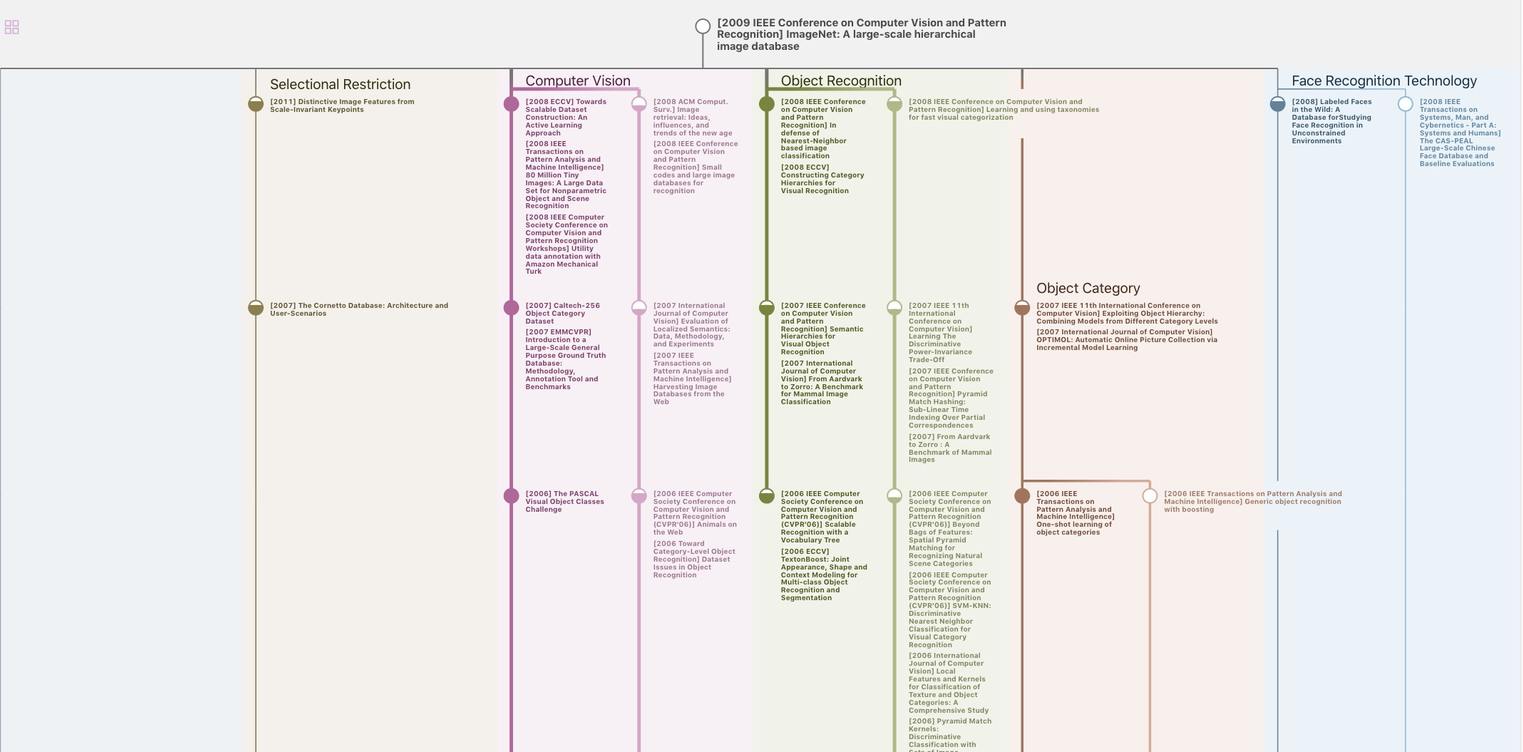
生成溯源树,研究论文发展脉络
Chat Paper
正在生成论文摘要