SDA-based neural network approach to digit classification
IEEE SoutheastCon-Proceedings(2016)
摘要
Image classification and object recognition are important issues in machine learning. With the increased usage of the internet and smart-based applications, image classification has become more important in various social, financial, and domestic spheres. Deep learning algorithms have proven useful and powerful, having successfully classified many types of images, objects, etc. This paper uses a type of deep neural network, stacked denoising autoencoders SDA), to classify the handwritten digits in the MNIST database. The method proposed transforms the images into the wavelet domain, for faster, more efficient processing, as compared to the spatial domain. Furthermore, the low frequency and high frequency components separately provide pertinent feature details learned by the SDA to correctly classify each digit. The fusion of the learned low and high frequency features, and processing the combined feature mapping results in an increased detection accuracy. Compared to traditional spatial) SDA and other classification algorithms, experimental results of this method show an increase in speed, efficiency, and accuracy in image classification and object recognition.
更多查看译文
关键词
SDA,Neural Network,Deep Learning,Wavelet,Classification,Fusion,Machine Learning,Object Recognition,Digits
AI 理解论文
溯源树
样例
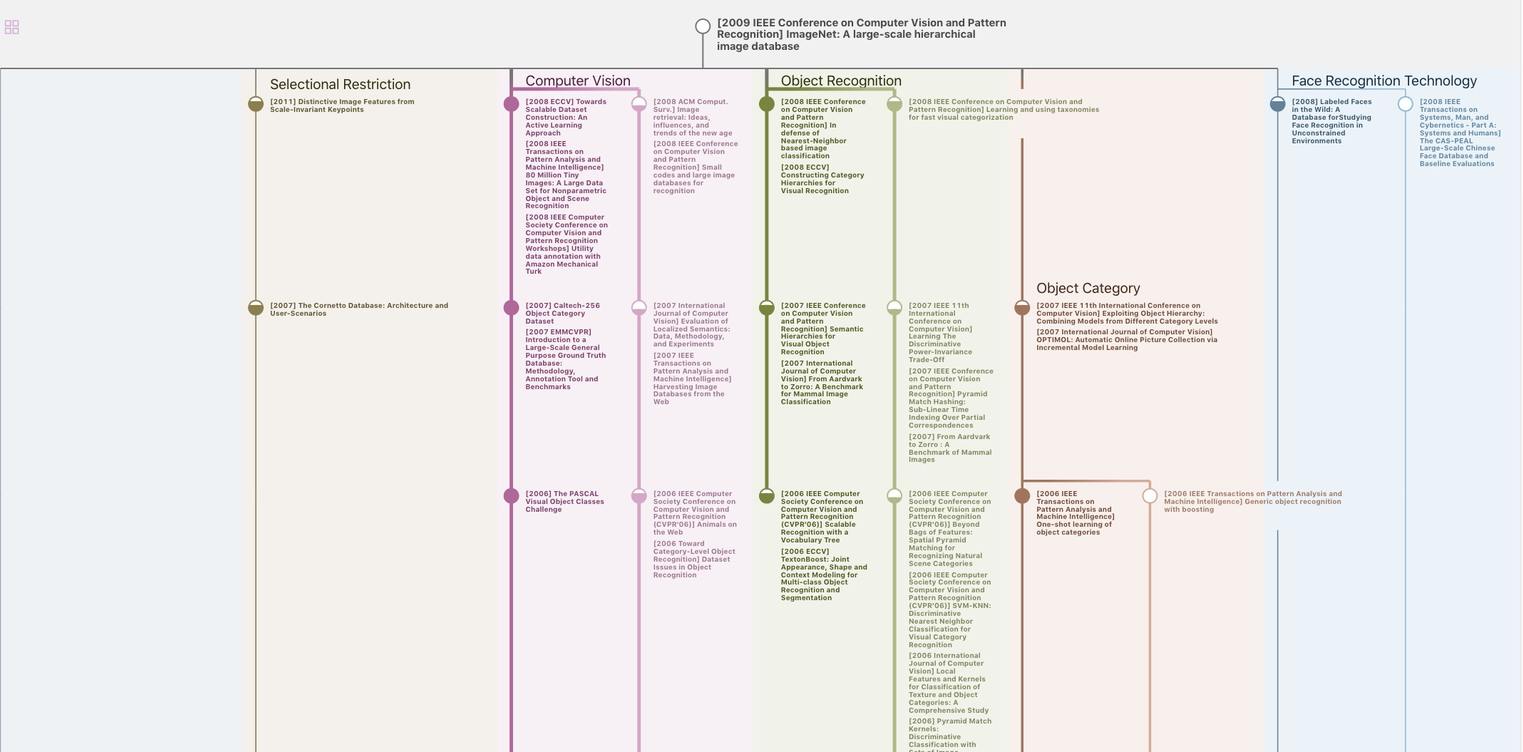
生成溯源树,研究论文发展脉络
Chat Paper
正在生成论文摘要