Modeling Activation Processes in Human Memory to Predict the Reuse of Tags
web science(2016)
摘要
Several successful tag recommendation mechanisms have been developed, including algorithms built upon Collaborative Filtering, Tensor Factorization, graph-based and simple most popular tags approaches. From an economic perspective, the latter approach has been convincing since calculating frequencies is computationally efficient and effective with respect to different recommender evaluation metrics. In this paper, we introduce a tag recommendation algorithm that mimics the way humans draw on items in their long-term memory in order to extend these conventional most popular tags approaches. Based on a theory of human memory, the approach estimates a tagu0027s reuse probability as a function of usage frequency and recency in the useru0027s past (base-level activation) as well as of the current semantic context (associative component). Using four real-world folksonomies gathered from bookmarks in BibSonomy, CiteULike, Delicious and Flickr, we show how refining frequency-based estimates by considering recency and semantic context outperforms conventional most popular tags approaches and another existing and very effective but less theory-driven, time-dependent recommendation mechanism. By combining our approach with a simple resource-specific frequency analysis, our algorithm outperforms other well-established algorithms, such as Collaborative Filtering, FolkRank and Pairwise Interaction Tensor Factorization with respect to recommender accuracy and runtime. We conclude that our approach provides an accurate and computationally efficient model of a useru0027s temporal tagging behavior. Moreover, we demonstrate how effective principles of recommender systems can be designed and implemented if human memory processes are taken into account.
更多查看译文
AI 理解论文
溯源树
样例
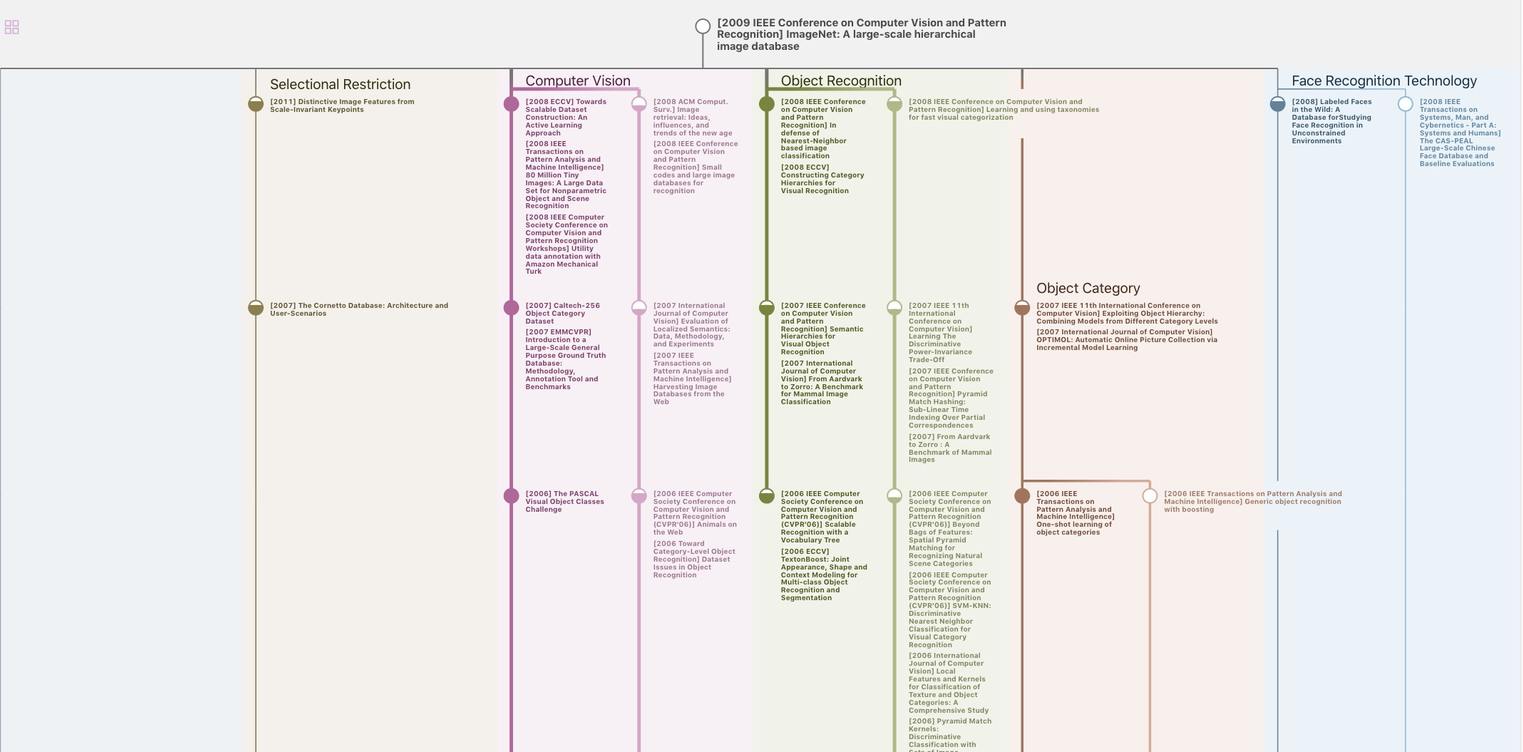
生成溯源树,研究论文发展脉络
Chat Paper
正在生成论文摘要