Analysis of Compounds Activity Concept Learned by SVM Using Robust Jaccard Based Low-dimensional Embedding
Schedae Informaticae(2015)
摘要
Support Vector Machines (SVM) with RBF kernel is one of the most successful models in machine learning based compounds biological activity pre- diction. Unfortunately, existing datasets are highly skewed and hard to analyze. During our research we try to answer the question how deep is activity concept modeled by SVM. We perform analysis using a model which embeds compoundsu0027 representations in a low-dimensional real space using near neighbour search with Jaccard similarity. As a result we show that concepts learned by SVM is not much more complex than slightly richer nearest neighbours search. As an addi- tional result, we propose a classification technique, based on Locally Sensitive Hashing approximating the Jaccard similarity through minhashing technique, which performs well on 80 tested datasets (consisting of 10 proteins with 8 dier- ent representations) while in the same time allows fast classification and ecient online training.
更多查看译文
AI 理解论文
溯源树
样例
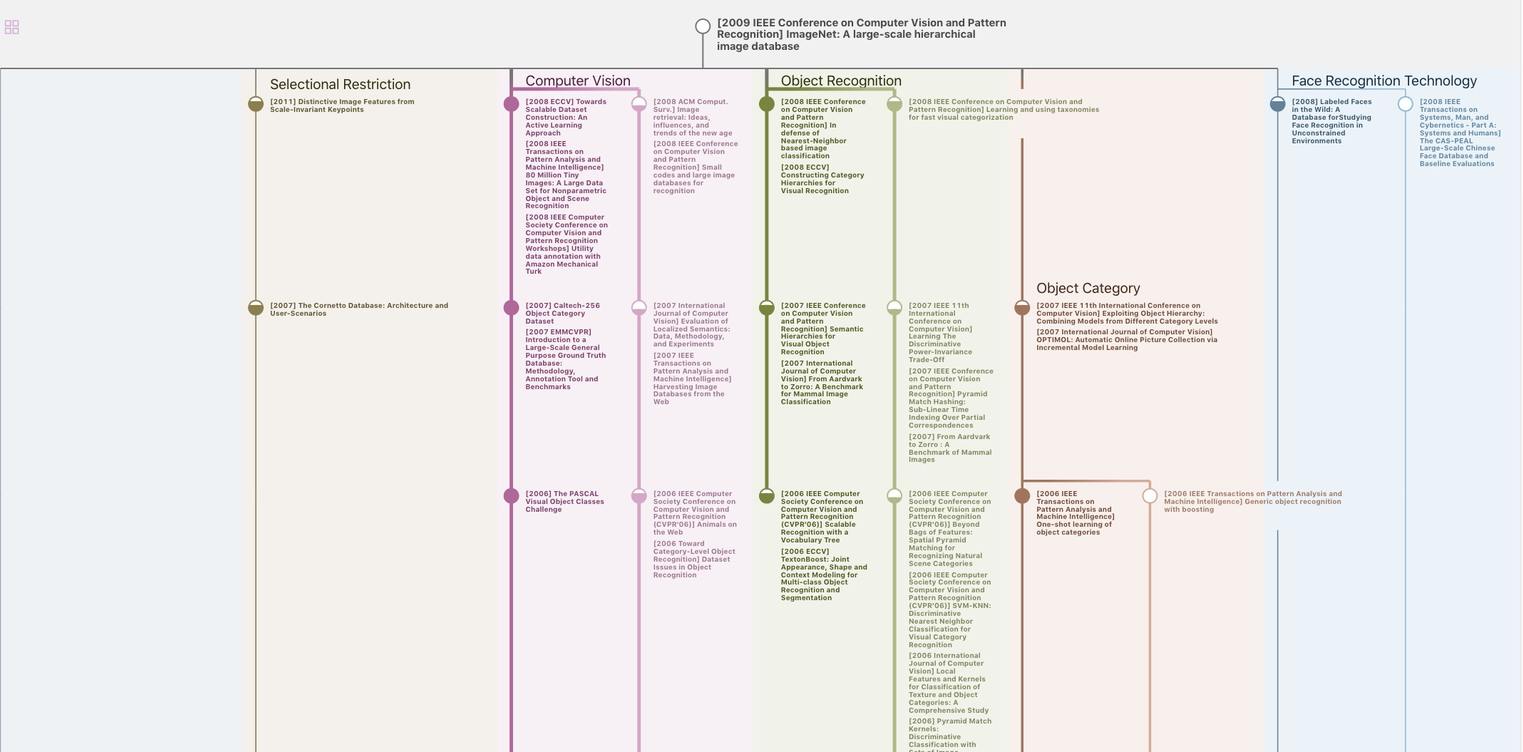
生成溯源树,研究论文发展脉络
Chat Paper
正在生成论文摘要