New metrics for learning and inference on sets, ontologies, and functions
arXiv: Machine Learning(2016)
摘要
We propose new metrics on sets, ontologies, and functions that can be used in various stages of probabilistic modeling, including exploratory data analysis, learning, inference, and result interpretation. These new functions unify and generalize some of the popular metrics on sets and functions, such as the Jaccard and bag distances on sets and Marczewski-Steinhaus distance on functions. We then introduce information-theoretic metrics on directed acyclic graphs drawn independently according to a fixed probability distribution and show how they can be used to calculate similarity between class labels for the objects with hierarchical output spaces (e.g., protein function). Finally, we provide evidence that the proposed metrics are useful by clustering species based solely on functional annotations available for subsets of their genes. The functional trees resemble evolutionary trees obtained by the phylogenetic analysis of their genomes.
更多查看译文
AI 理解论文
溯源树
样例
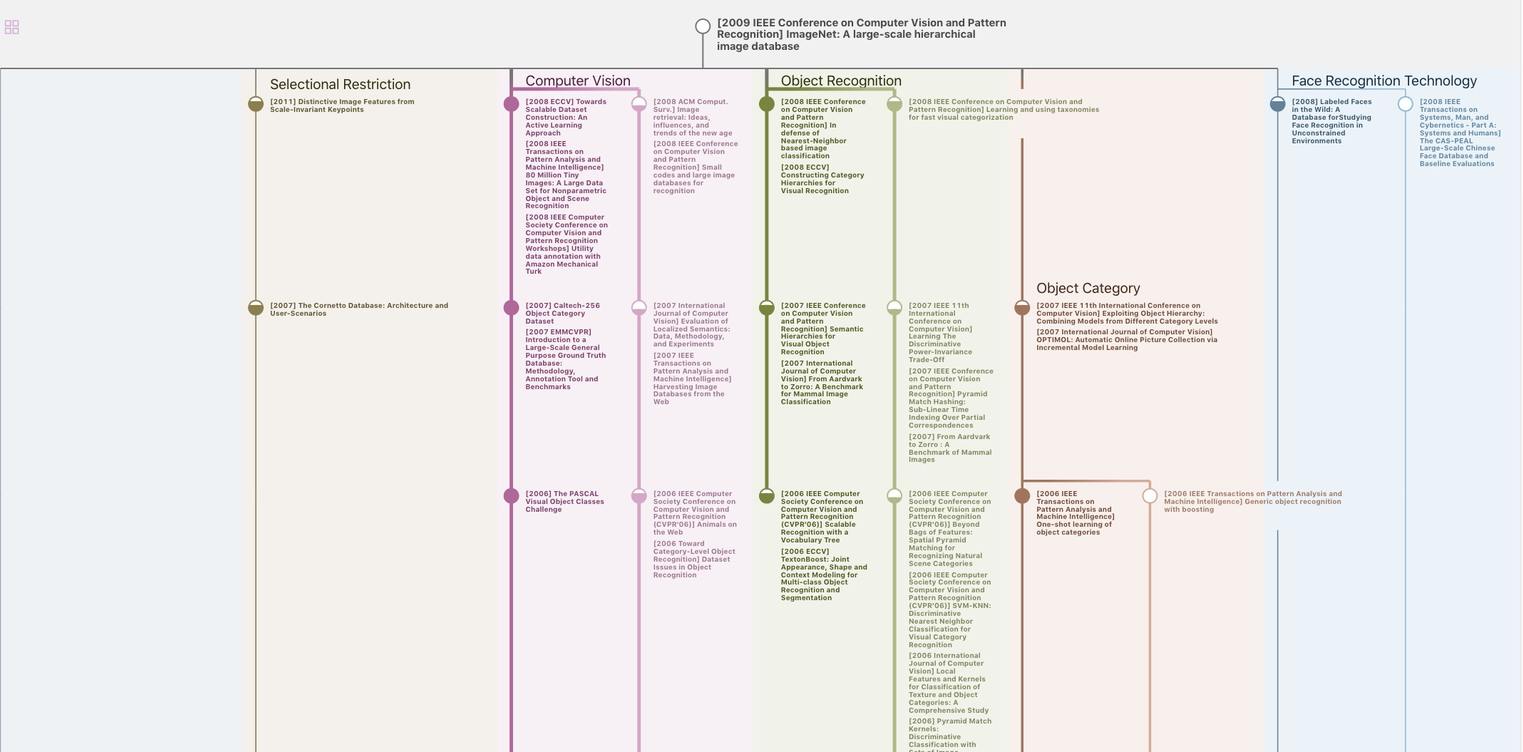
生成溯源树,研究论文发展脉络
Chat Paper
正在生成论文摘要