Convolutional Neural Networks in Automatic Recognition of Trans-differentiated Neural Progenitor Cells under Bright-Field Microscopy
2015 Fifth International Conference on Instrumentation and Measurement, Computer, Communication and Control (IMCCC)(2015)
摘要
The study of cell morphology changes leads the investigation of the cell fate decision and its function. Bright-field imaging analysis allow us to use a labeling free and non-invasive approach to measure the morphological dynamics during cellular reprogramming, which includes induced pluripotent stem cells (iPSCs), and trans-differentiated neural progenitor cells (NPCs) from somatic cell source. However, the traditional method to study the NPC differentiation and its related function involves staining, and cell lysis, which can not materialized further for the clinical uses. In order to automatically, non-invasively, non-labelled analyze and cultivate cells, a system classifying NPCs under bright-field microscopic imaging is necessary. In this paper, we propose a novel recognition system based on convolutional neural networks, which could pre-process images and classify NPCs and non-NPCs. Experimental results prove that the proposed system provides a new tool for fundamental research in iPSCs and NPCs based generation medicine.
更多查看译文
关键词
machine learning,deep learning,convolutional neural networks,trans-differentiated neural progenitor cells,non-invasive,non-labelled,bright-field microscopy
AI 理解论文
溯源树
样例
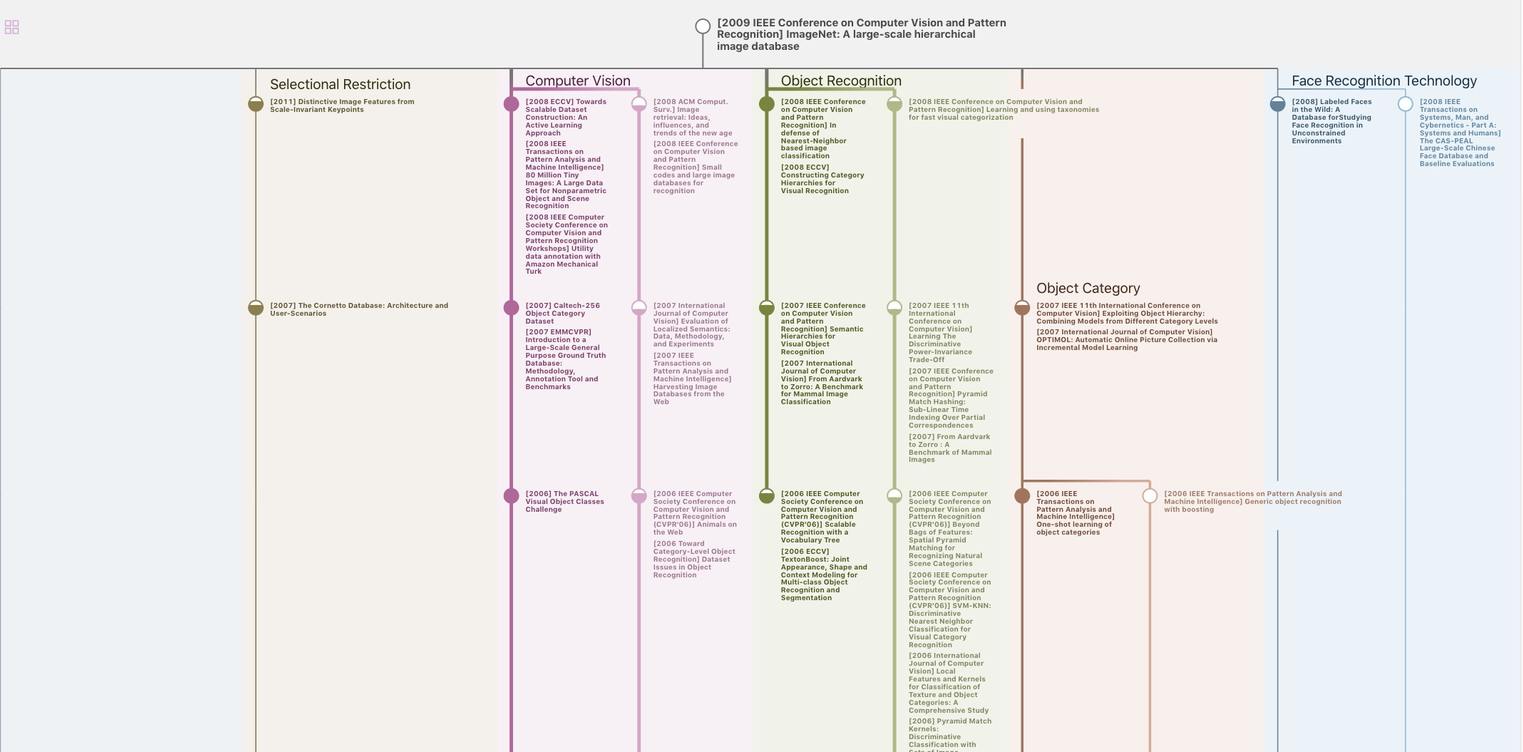
生成溯源树,研究论文发展脉络
Chat Paper
正在生成论文摘要