The Effect of Gradient Noise on the Energy Landscape of Deep Networks
arXiv: Learning(2015)
摘要
We analyze the regularization properties of additive gradient noise in the training of deep networks by posing it as finding the ground state of the Hamiltonian of a spherical spin glass in an external magnetic field. We show that depending upon the magnitude of the magnetic field, the Hamiltonian changes dramatically from a highly non-convex energy landscape with exponentially many critical points to a regime with polynomially many critical points and finally, "trivializes"' to exactly one minimum. This phenomenon, known as topology trivialization in the physics literature, can be leveraged to devise annealing schemes for additive noise such that the training starts in the polynomial regime but gradually morphs the energy landscape into the original one as training progresses. We demonstrate through experiments on fully-connected and convolutional neural networks that annealing schemes based on trivialization lead to accelerated training and also improve generalization error.
更多查看译文
AI 理解论文
溯源树
样例
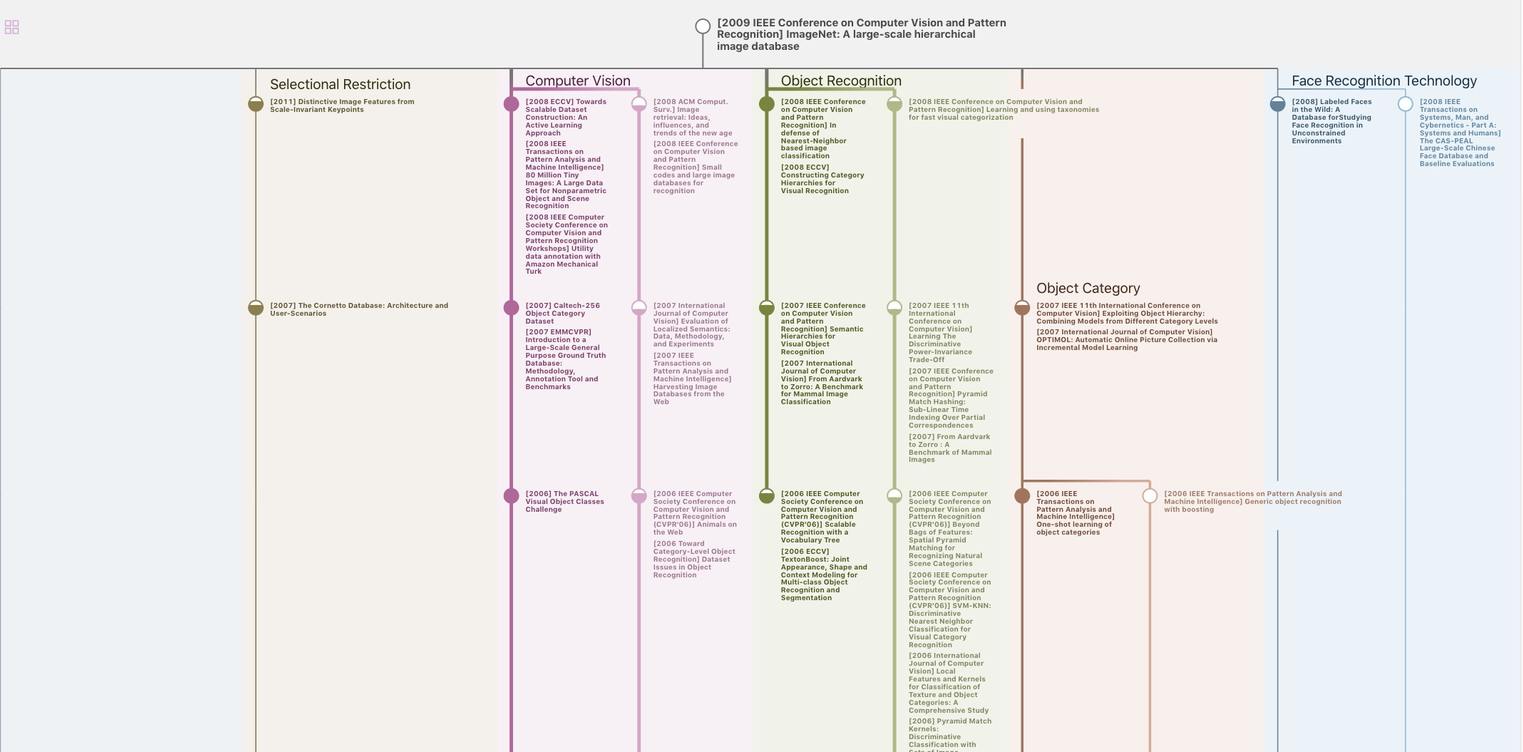
生成溯源树,研究论文发展脉络
Chat Paper
正在生成论文摘要