Platform Variability in Edge-Cloud Vision Systems
Proceedings of the 20th International Workshop on Mobile Computing Systems and Applications(2019)
摘要
Modern edge devices such as smartphones, tablets, smart glasses, VR and AR headsets have gotten more capable with high-resolution displays, powerful CPU/GPUs, multiple sensing modalities and multiple connectivity options. These platform capabilities are enabling complex sensing applications that integrate visual, inertial, and other sensing modalities in real-time to enable applications such as indoor navigation, object recognition, face recognition, activity recognition, and others. Typically, such applications are computationally-intensive and require execution of complex computer vision pipelines for effective execution. While edge devices are getting more powerful, it is still challenging to run such computationally intense applications exclusively on the edge. To address this challenge, several solutions propose splitting the computing between the cloud and the edge. However, these solutions are typically tested for a few seconds/minutes. Our observation is that this ignores platform constraints when multiple apps are running on the same edge device and/or long-term operation, resulting in platform variability such as voltage/frequency scaling, shutting down of CPU cores, etc. all of which affect the application performance. We build an edge-cloud vision system and use it to demonstrate this concern through experiments. Our proposal is that future edge-cloud systems need to explicitly test for platform variation due to long-term operation in order to demonstrate feasibility.
更多查看译文
关键词
edge computing, edge intelligence, edge-cloud systems, object-detection and tracking, performance of edge systems, tensorflow, vision systems
AI 理解论文
溯源树
样例
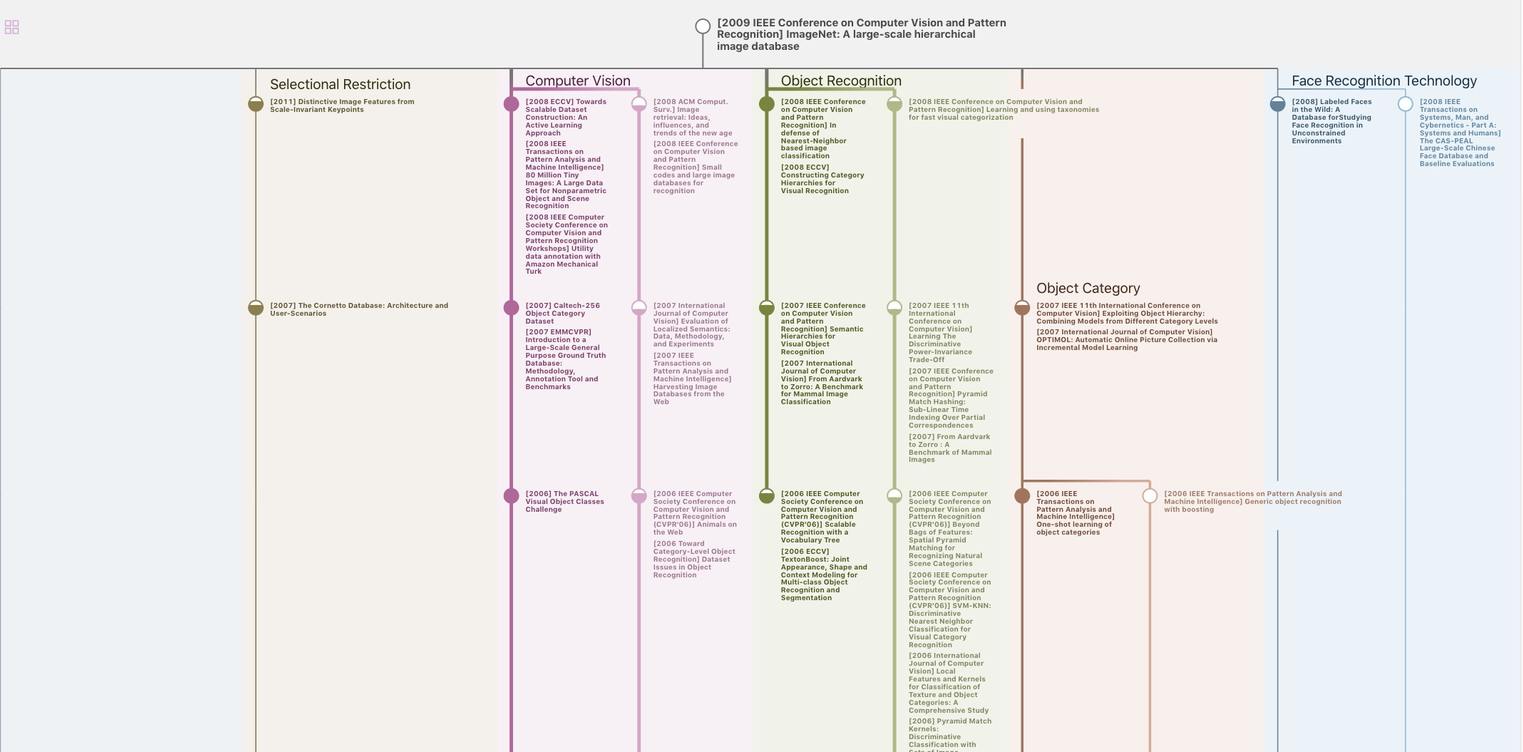
生成溯源树,研究论文发展脉络
Chat Paper
正在生成论文摘要