Subspace Analysis in Multi-Class Datasets: An Application to Novelty Detection Ensembles
2018 IEEE International Conference on the Science of Electrical Engineering in Israel (ICSEE)(2018)
摘要
Subspace analysis is a recurring subject in many real-world applications of novelty detection ensembles. Yet, most of proposed works focused on one-class datasets, leaving multiclass datasets for classical tasks of classification with rejection. We propose a novel subspace analysis method for multi-class datasets, named ‘Multi-Class Agglomerative Attribute Grouping (MAAG)’ to be used in ensembles for novelty detection. The MAAG method aims at achieving a stable set of meaningful subspaces relying on a novel distance metric derived from information theory principles to evaluate the ‘information distance’ between groups of data attributes. The MAAG combines the proposed distance with a term derived from the chain rule of Mutual Information to generate compact clusters of observations, while maintaining them well separated among the different classes. Therefore, the MAAG aims at facilitating the merging of objectives for classification and novelty detection simultaneously. Our empirical evaluation shows that the proposed MAAG boosts the novelty-detection performance of methods based on ensemble-of-classifiers, and outperforms on average, other state-of-the-art subspace analysis approaches applied to each individual class in multi-class settings.
更多查看译文
AI 理解论文
溯源树
样例
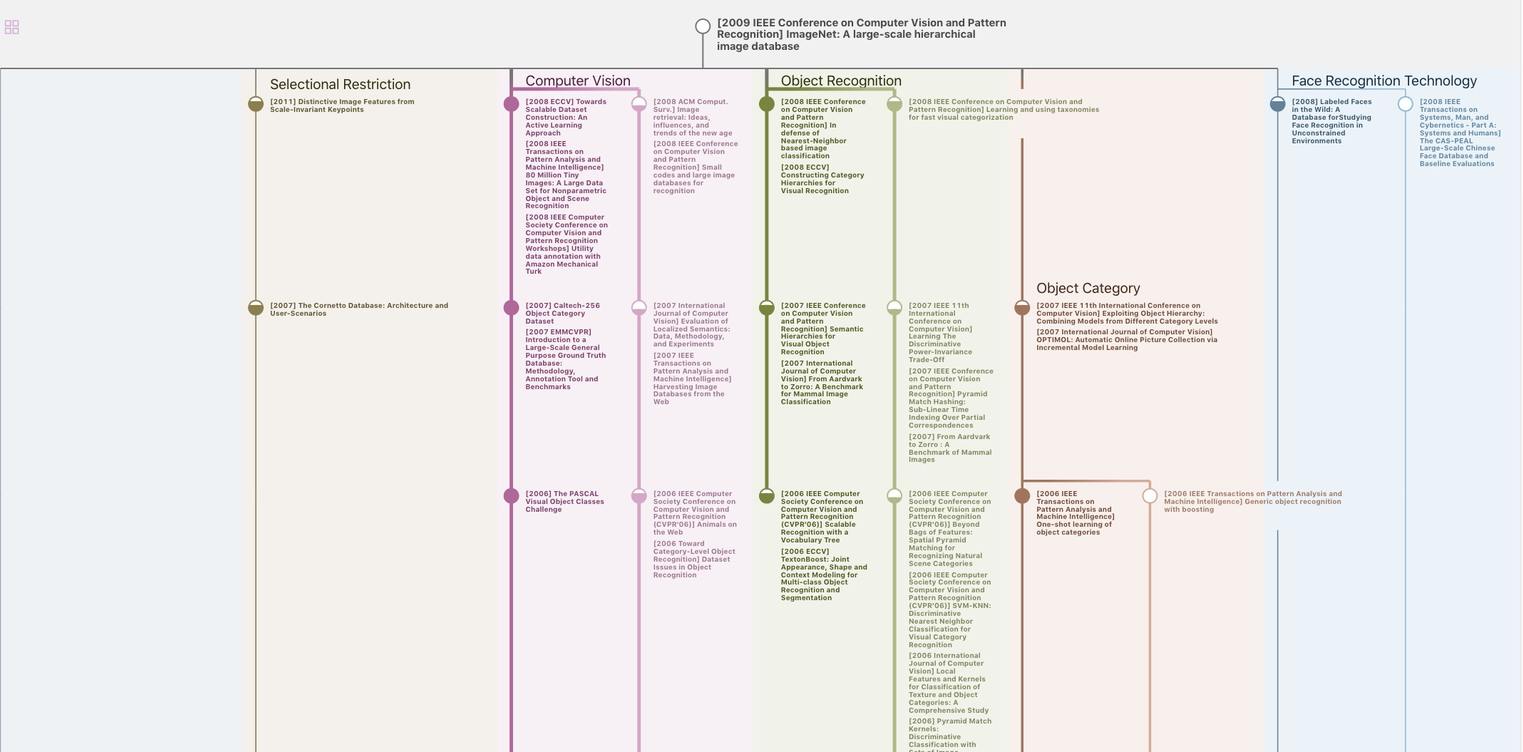
生成溯源树,研究论文发展脉络
Chat Paper
正在生成论文摘要