Speaker Differentiation Using a Convolutional Autoencoder
2018 IEEE International Conference on the Science of Electrical Engineering in Israel (ICSEE)(2018)
摘要
In this work, a deep learning solution for differentiating speaker voices in audio given two microphone sources is presented as a step towards solving the cocktail party problem. A convolutional autoencoder was trained using a small sample size of data to associate audio snippets with categorical labels. Audio snippets collected as part of this work were used for training and evaluating the model. Audio was converted to mel-frequency cepstrum representation prior to classification. The collective processed data was labeled according to the person or collection of persons speaking. The model was trained and evaluated using data with two, three, four, five, and six categories. The result was a model that recognizes when different people are speaking in a 2-person, 3-person, 4-person, 5-person, and 6-person conversation with an accuracy of 99.29%, 97.62%, 96.43%, 93.43%, and 88.1%, respectively. Experimental comparisons between the five versions of the model are presented.
更多查看译文
关键词
deep learning,convoluional neural network,voice separation,signal processing
AI 理解论文
溯源树
样例
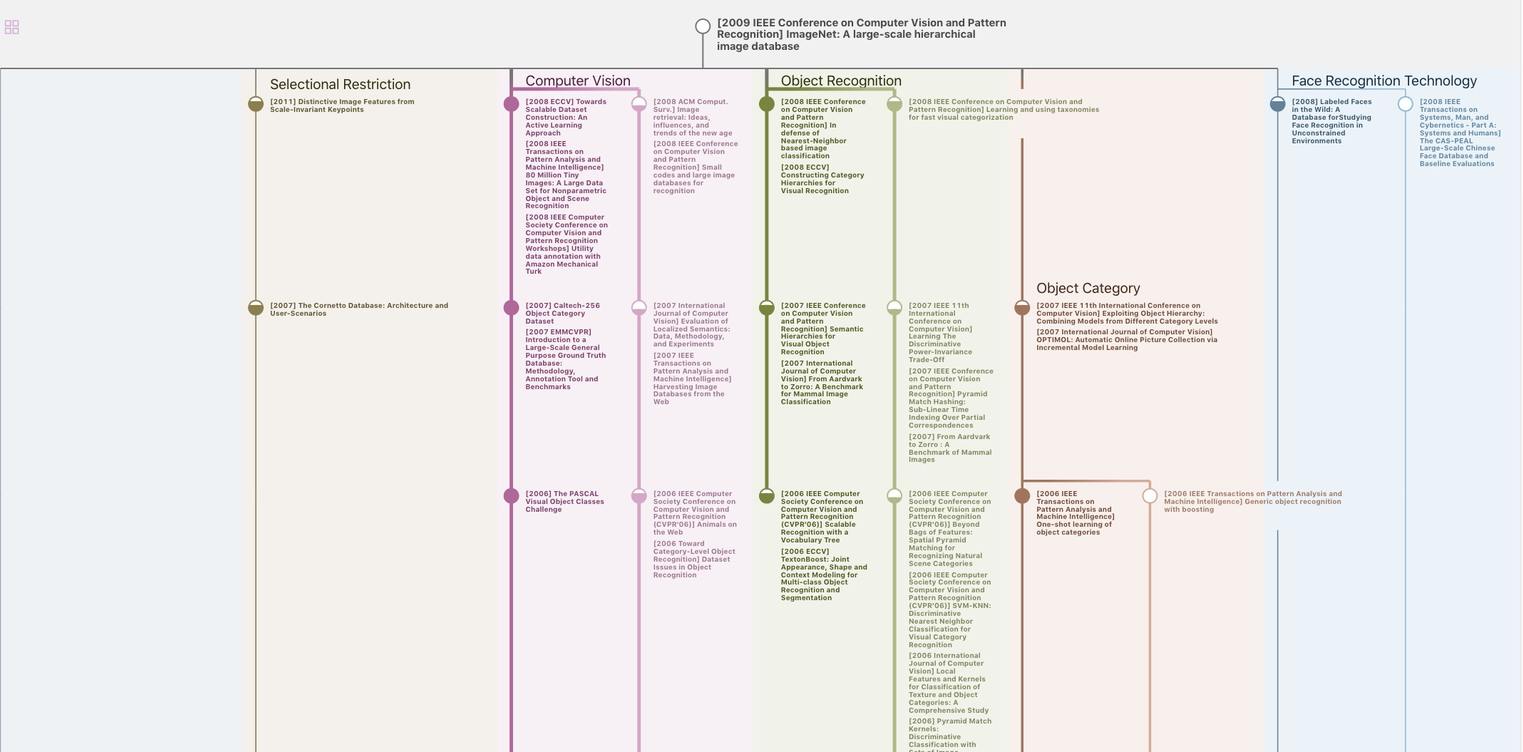
生成溯源树,研究论文发展脉络
Chat Paper
正在生成论文摘要