mrMoulder: A recommendation-based adaptive parameter tuning approach for big data processing platform.
Future Generation Computer Systems(2019)
摘要
Nowadays the world has entered the big data era. Big data processing platforms, such as Hadoop and Spark, are increasingly adopted by many applications, in which there are numerous parameters that can be tuned to improve processing performance for big data platform operators. However, due to the large number of these parameters and the complex relationship among them, it is very time-consuming to manually tune parameters. Therefore, it is a challenge to automatically configure parameters as quickly as possible to optimize the performance of the current job. Existing auto-tuning methods often take a certain time before job runs to get the optimal configuration, which would increase the job’s total processing time and reduce the overall efficiency of cluster. In this paper, we propose an adaptive tuning framework, mrMoulder, to recommend a near-optimal configuration for the new job in a short time. mrMoulder sets a self-extending configuration repository and a collaborative filtering based recommendation engine, to speed up the process of optimizing parameter configuration. We have deployed mrMoulder in a Hadoop cluster, and the experiment results have demonstrated that, for a new big data application, the recommend time of mrMoulder is only 20% to 30% of that for the existing auto-tuning methods, while the recommendation quality remains almost unchanged.
更多查看译文
关键词
Big data processing,Performance optimization,Parameter tuning,Online configuration recommendation,Collaborative filtering
AI 理解论文
溯源树
样例
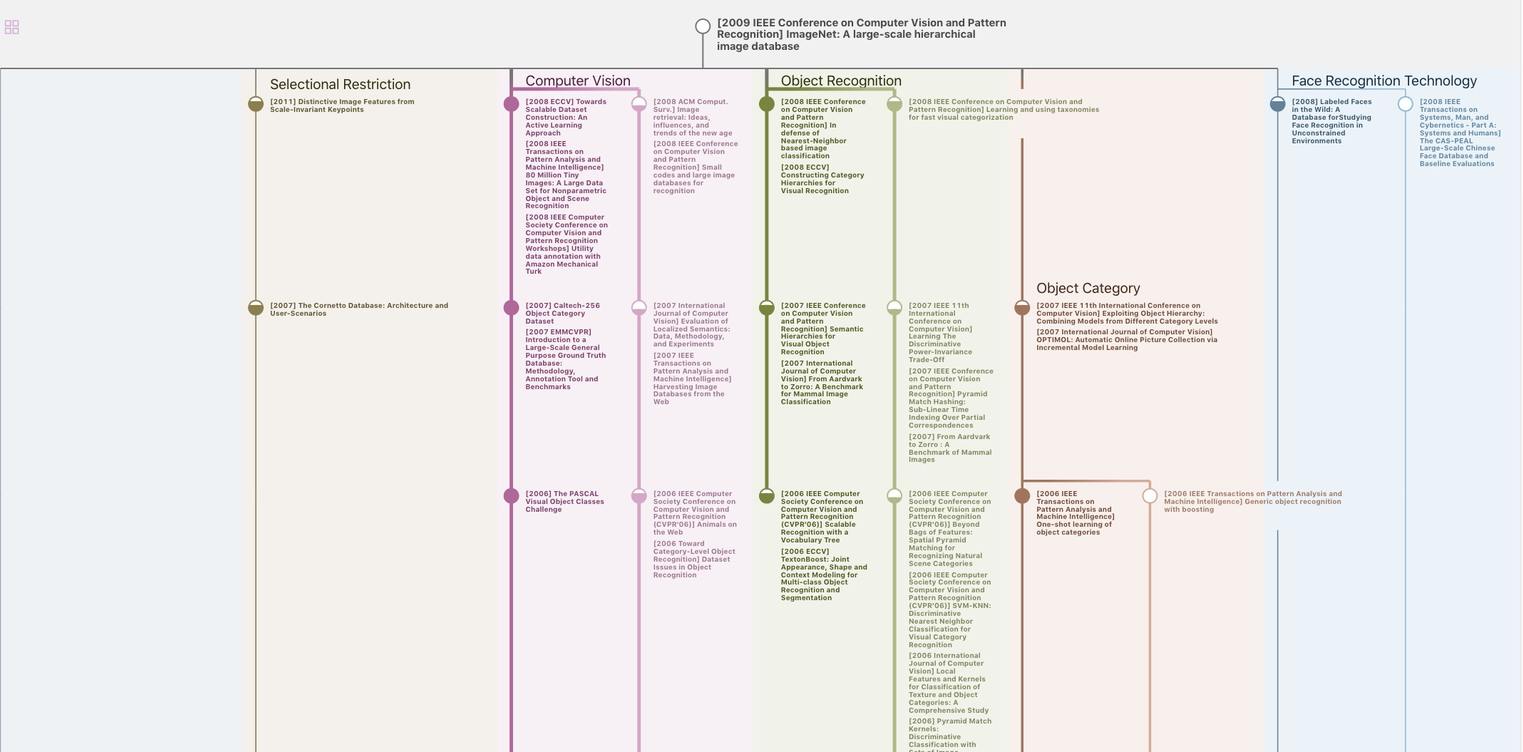
生成溯源树,研究论文发展脉络
Chat Paper
正在生成论文摘要